Agent-based modeling of regional healthcare: addressing the task of formalizing residents' medical activity
Автор: Dianov Sergei V., Kalashnikov Konstantin N., Rigin Vasilii A.
Журнал: Economic and Social Changes: Facts, Trends, Forecast @volnc-esc-en
Рубрика: Theoretical and methodological issues
Статья в выпуске: 1 т.15, 2022 года.
Бесплатный доступ
The article addresses the task concerning the development of techniques for formalizing the behavior of agents-users of medical services in agent-based models designed to support decision-making on the spatial layout of health infrastructure facilities in the region. We analyze approaches to determining the behavior patterns of consumers of medical services and, on this basis, develop the structure of agents' behavior model. We consider the implementation of the proposed model with the help of fuzzy neural networks. While forming the network, we use the results of a sociological survey conducted by Vologda Research Center in the territory of the Vologda Oblast in 2020. Practical implementation of the network is carried out in the Microsoft Access environment. Using this tool, we conduct a number of experiments to determine residents' medical activity based on the initial data of the sociological survey. We use general scientific methods of formalization, abstraction, generalization, methods of system analysis, mathematical statistics, and fuzzy logic. The results of our work consist in designing the structure of behavior model for agents-consumers of medical services and methods for its implementation. Scientific novelty of our findings consists in the proposed structure of behavior model of agents-consumers of medical services and its practical implementation with the use of fuzzy neural networks in relation to the manifestation of medical activity. Practical significance of the results lies in the fact that the instrumental approaches proposed and partially tested in it will allow us to develop agent-based models of regional healthcare adequate to objective environmental conditions, taking into account citizens' attitudes and behavior motives in interaction with medical services. In the future, this will help us to put forward and adjust measures to improve the efficiency of spatial layout and functioning of networks. Further work will be related to the improvement of the proposed methods in the following areas: determining ways to train the developed fuzzy neural network and finding ways to implement the remaining elements of the developed model for agent behavior.
Healthcare infrastructure facilities, medical services, medical activity, agent-based modeling, fuzzy neural networks
Короткий адрес: https://sciup.org/147237317
IDR: 147237317
Текст научной статьи Agent-based modeling of regional healthcare: addressing the task of formalizing residents' medical activity
The reported study was funded by the RFBR (project number 20-010-00852 А).
In the context of development of technological capabilities of modern medicine and amid an increased interest of citizens in obtaining medical services, regions are faced with an important socio-economic task to form an effective spatial health infrastructure that ensures an accessible environment for the provision of services. In modern conditions, this problem cannot be solved without the use of simulation tools. We are implementing a project to develop scenarios for an optimal spatial layout and functioning of regional healthcare infrastructure with the use of agent-based simulation models. The main idea of the project is to form an architecture of agent-based models for the spatial network of regional healthcare, taking into account fundamental parameters of the sector’s institutional environment, including the blurring of the boundaries between the public and commercial sectors, as well as features of residents’ behavior in interaction with medical organizations, which in modern conditions assume a specific character. The novelty of the project lies in the fact that the object of simulation is the healthcare system, including not only basic government structures, but also the profit sector and the “third” sector. In addition, the constructed simulation models will be based on a set of reliable data combining official statistical reports, geo-information systems and sociological surveys conducted with our direct participation. The study and formalization of residents’ motives and behavioral attitudes in relation to their medical activity and their choice of strategies for seeking medical care at medical institutions, including commercial ones, will allow taking into account the criterion of accessibility of medical care for citizens in simulating and further elaborating on management measures. Thus, the model will be based on objective prerequisites, actual needs, motives and behavior of agents; consequently, it can used in management practice and testing alternative scenarios for decision-making and implementation of regional policy.
The essence of the project is to apply agentbased modeling so as to solve the problems of spatial layout of networks of the regional health system (in the case of the Vologda Oblast), ensuring its effective functioning in the interests of the population.
The main purpose of the study is to develop methods for formalizing the behavior of agent users of medical services in an agent-based model of optimal spatial layout and functioning of the health infrastructure in the region. To achieve this goal, the following tasks were addressed:
– developing a general concept of an agentbased model, which defines its structural and functional components;
– analyzing behavioral patterns of consumers of medical services;
– implementing a mechanism of manifestation of medical activity in the behavior of agents-users of medical services.
The novelty of our research results consists in the proposed structure of the behavior model of consumer agents of medical services and its practical implementation using fuzzy neural networks in relation to the manifestation of medical activity.
Practical significance of the results obtained consists in ensuring the possibility of implementing agent-based models designed to support decisionmaking related to the management of the healthcare system at the regional level. The results can be useful for administrative structures at the regional and municipal levels of government.
It is almost impossible to get a real picture regarding the health status of population in a particular region because of the extreme dynamism of this category and also due to limited technical capabilities of modern medicine. Anyway, there is no need to get such a picture. It is sufficient to use a system that includes the study of people’s well-being on the basis of representative surveys. Our study used data from the sociological survey “Studying the health of the population and its determining factors”, which is regularly conducted by VolRC RAS among Vologda Oblast residents; 1,500 people living in cities, towns and rural settlements of the Vologda Oblast participate in the survey. The sample of respondents is representative and quota-based. Sampling error does not exceed 3%. The article analyzes survey data for 2020 in order to identify residents’ medical activity in the context of gender, age, education, place of residence and social status. Respondents’ medical activity is assessed on the basis of questionnaires they filled in. Answers to the following questions: “What do you usually do when you are feeling unwell?” (answer options: “I try to put up with it, I don’t consult a doctor and I don’t try to find treatment on my own; it will pass, somehow”) and “What do you do to preserve and strengthen your health?” (answer option: “I go to the doctor at the first signs of illness, I regularly undergo medical examination”) help us to differentiate agents by the extent of medical activity. The proportion of positive answers to these questions is the source of determining the parameters of membership functions.
Health consistently ranks first in the priorities of Russians; in contrast, their satisfaction with the availability of medical care is the lowest among life satisfaction parameters1. The main obstacles to an adequate response of Russian healthcare to the expectations of citizens include the lack of resource provision combined with the underutilization of numerous reserves to increase the efficiency of the use of available material, labor, and financial resources2.
The current concept for development of Russian healthcare is based on an approach according to which the priority task in the implementation of the program of state guarantees is to ensure the availability and quality of medical care that satisfies people’s needs and uses state resources effectively3. Rationing of resource consumption by the healthcare system involves comparing the costs of medical services and, in general, the amount of medical care provided, with the results identified during their provision. The best result obtained at lower costs indicates the optimal use of resources in the production of a unit of production. Medical and social effectiveness is considered from the standpoint of reducing morbidity, disability, mortality, increasing life expectancy and its quality.
A picture of what is happening can be formed by studying the situation on the basis of statistical data, i.e. on information that claims to be scientific, accurate and reliable. This approach underlies the development of management decisions in the field of healthcare and the state discourse on medicine (Mikhailova et al., 2007). Healthcare planning in the Russian Federation is carried out at three levels: federal, regional and municipal. The federal level is mainly responsible for the formation of a general strategy. At the regional and municipal levels, the planning of volumes and types of medical care is carried out, taking into account the capacity and structure of the institution, and the number of the population registered with the institution.
Healthcare planning uses various methods: analytical, balance, regulatory, etc. All these methods are almost fully used in the experimental method; any innovation is necessarily tested and evaluated during an experiment. In this regard, of great importance is the task performed by computer models to construct a socio-economic context with further “rehearsal” verification and approbation on the proposed simulation model of management decisions. Agent-based models belonging to the class of models based on the individual behavior of agents and created for computer simulations are especially promising in the economy and social sphere. Designing an agent-based model as close to reality as possible, which would reflect the set of basic and fundamental transactions between agents within the regional healthcare system, will help to adopt adequate management decisions regarding the allocation of resources and a rational spatial layout of infrastructure facilities in the sector.
In our opinion, agent-based modeling is a particularly appropriate way to design realistic scenarios for spatial location of social infrastructure objects and organize effective routing algorithms for citizens in the context of the regional spatial system. The use of agent-based modeling in addressing applied issues of public administration is to a certain extent universal. In other words, there are no instrumental differences in the application of an agent-based approach to urban economy, education or healthcare. According to some foreign studies, it is a powerful and flexible tool for developing optimal management decisions at various levels (Gaffard, Napoletano, 2012; Dawid, Neugart, 2011; Mendritzki, 2010; Mendritzki, 2010; Badham et al., 2018; Silverman, 2014). However, the very characteristics fed into the model should be extremely specific and correspond as much as possible to the actual practice of management and activity in a particular area. The experience of agent-based modeling in Russia is contained mainly in the works of scientists from the Central Economics and Mathematics Institute, RAS (CEMI RAS) under the leadership of Academician V.L. Makarov (Makarov, Bakhtizin, Sushko, 2016, 2019; Bakhtizin, 2008).
Agent-based modeling (ABM) is becoming increasingly widespread in the healthcare sector. Formerly, scientists used it only to model epidemiological diseases and their spread among populations, while nowadays the scope of application of ABM has become wider (Tracy, Cerda, Keyes, 2018). In comparison with traditional analytical approaches, ABM helps to solve a wider range of problems, so that it can shed new light on public health issues. In fact, the two main goals of ABM related to public health are to explain and predict the outcomes regarding people’s health, taking into account aspects of a complex system in which public health exists. From these goals proceed the main advantages of ABM for research, practice, and policy in the sphere of public health: these models provide insight into the underlying mechanisms that determine health behaviors and outcomes (as well as inequalities in these behaviors and outcomes), and they can be used to conduct virtual experiments on interventions aimed at reducing the burden of disease among people.
Thus, there is significant and important global experience of methodological elaboration and practical implementation of agent-based modeling in the practice of public administration in general and in healthcare in particular; but still insufficient attention is paid to aspects of spatial layout of health infrastructure facilities, which, in addition, rarely rely on people’s actual needs and behavioral attitudes of various social groups.
The conceptual basis of the models will include agents and their functioning environment. Popu- lation of the region and some elements of the resource provision of the regional healthcare system will be considered as agents. Population agents are identified with certain social groups that have similar behavior in terms of needs for medical services. The main purpose of their functioning is the most complete satisfaction of these needs. To achieve these goals, they interact with medical resources agents. We propose to create an interactive system for the formation of the structure of population agents within the framework of the models, taking into account changes in the parameters of the environment of the functioning of agents and the structure of medical resources agents. Medical resources agents act as service providers for population agents. They can be passive, which means they wait for resident agents to seek medical care from them, or they can be active, which means they search for possible clients of the services they provide. The environment of the agents’ functioning consists of elements that influence the structure and behavior of agents within the model. Such elements will include, in particular, elements of transport infrastructure, communication infrastructure, industrial infrastructure, natural and climatic features of territories, etc. The model elements have a spatial reference, according to which the nature and degree of their interaction is formed. At the same time, the agents will be able to move spatially. The proposed approaches will provide the most optimal solution to the problem of efficient use of health resources at the regional level due to the possibility of taking into account elements of the lower level of the system when making decisions, as well as the possibility of operational reconfiguration of the model in the conditions of dynamic changes in the subject area of simulation. For the successful use of the model in making managerial decisions and in comparing resource allocation options, we think that its architecture should be constructed with the use of objective data that accurately describe the demographic, institutional and economic context (the nature of settlement, the number and age structure of the population, the provision of health infrastructure facilities, medical personnel and financial resources, the development of the commercial sector of medical services). Integration with geo-information systems and reliance on data from sociological surveys that describe the actual specifics of citizens’ interaction with medical institutions will add special functionality to the model.
When building the models, we plan to focus on optimizing the spatial layout of the network of medical institutions, taking into account the levels, stages and routing of patients (rural health clinics (FAPs) – central district hospitals – regional healthcare institutions, etc.), as well as the growing influence of commercial sector services on what the patients will choose. At the same time, the main emphasis will be placed on the disparities in the availability of medical care between different categories of the population (they will be grouped into homogeneous communities and act as agents in the models). The practical significance of the model lies in the fact that it will be used as a tool for testing regional governance mechanisms. The development and testing of various scenarios for spatial placement of health infrastructure networks and agent interaction (conducting computer experiments on an artificial society) with the use of the model will helps us avoid the trial-and-error practice in the implementation of regional public health policy. Ultimately, the model should help to work out measures to improve the spatial layout of the network of medical institutions based on the behavior of agents (patients) and also depending on the influence of the designated group of factors, which will help to increase the efficiency of the system and the accessibility of medical care for citizens.
We believe that the scenarios we are going to design for the spatial placement of health infrastructure facilities should be based on objective imperatives in the behavior of residents of territories in their interaction with medical services; they should also be based on migration attitudes and a set of internal factors, including gender and age, education, welfare, place of residence, etc. Thus, when implementing the project, it is of particular importance to determine methods for formalizing the behavior of users of medical services in an agent-based model of optimal spatial layout and functioning of regional health infrastructure.
Formalization of the task
The task of simulation is based on three pillars: services, service mobility and user agents. User agents have mobility, can move freely in space, reach points of interest – services. The latter, in turn, are characterized by various mobility opportunities. A number of services have the function of moving and transporting an agent from a place of residence or an incident that caused the need for medical care (ambulance, doctor’s home visit, mobile medical services). Other services are in-patient (for example, medical appointment, pre-arranged hospital care). In general, the interactions of user agents and services are to a certain extent regulated by legislative documents, resolutions and protocols; this fact provides ample opportunities for the precise structuring of their points of contact, distribution of user placement nodes and points of interest within the agentbased model. A significant problem consists in the formalization of user behavior, since it depends on behavioral factors, habits and cultural attitudes of the population. In the simplest version, the user within the framework of the model exists in two alternative modes – the presence, the feeling of the need for services and assistance, and the absence of such a need. In some cases, primarily in emergency situations, the need becomes more objective and obvious when the fact of the agent’s need for help can be registered by other agents (a traffic accident, a violent act, a serious health deterioration causing a blackout), however, in most cases, the assessment of the need to go to the hospital is dictated solely by the free choice of the person themselves.
The task of optimal placement of health services is related to the search for options that provide the best characteristics of the system, taking into account the selected criteria. To date, there are no unambiguously defined optimality criteria. As a rule, they are formulated at the level of public administration on the basis of accepted priorities and existing opportunities. In most cases, they are associated with time restrictions on the possibility of receiving a particular service.
In general, the model is presented as follows (Dianov, 2020):
М = (U, R, S, С) , (1)
where U denotes the nodes where services and clients are located,
R denotes the connections between the nodes, S denotes services, C – users.
Services and users can be located in a limited number of places. Based on this, the model M can have a certain number of nodes where services and users are located:
Between the nodes there may be many connections – ways of moving services and users:
The nodes and connections have a set of different attributes:
U: Au = {A U ,., A Uun } ,
R: Ar = {AR..... ARrn}.
The model contains many services and users:
In relation to the task we are addressing, they possess active behavior and belong to the category of agents.
The Service agent can be described as follows:
5 = (Ut , As , Bhs^ , (7)
where Ui is the node of the permanent location of the service,
A S is a set of attributes of the service ( As = = {As, —, a asn } ),
BhS is a behavior pattern of the service.
The User agent has a similar description:
C = t , Ac , Bh C , (8)
where Ui is the node of the user’s permanent location,
A C is the set of the user’s attributes ( Ac = = {Ac, .„, aC cn }),
BhC is the user’s behavior model.
Behavior models contain modules in which agents’ behavior scenarios are defined. Modules consist of a set of rules that allow the agent to select a particular scenario depending on the current values of parameters of the model’s elements.
Two modules are defined in the Service agent’s behavior model:
Bhs = {MdR , Mdsd } , (9)
where Md l is a module of service provision scenarios,
Mdd is a module of movement scenarios.
Services agents can be of two types: stationary, rigidly tied to a specific node, and movable. For stationary agents, the module of movement scenarios is not defined: Mdd = 0 .
At the level of the module of service provision scenarios, the possibility of and the procedure for providing a service to a specific user is determined. Based on this, the rules use the values of the user and service attributes:
Mdss = {pr 1MdSs ( aS , Дс ), _, prMdSs rAs дсл)
, -■' I' PrMdSsN (a , a ))
where Pr -^^^ ^X ^ , .„, XXN ) is the i -th rule of the module, containing the parameters X 1 ,^, XXN .
At the level of the movement scenarios module, we determine the necessity, possibility, parameters and route of the agent’s movement:
M da = {Pr1Masa (As , Ac, Au, Ar ),...,
PrMasa sas Cc Au . (11)
•“, ^'PrMasaN(A ,A ,A ,A )j
Two modules are also defined in the User agent behavior model:
Bh^ = ^Mdcgu, Md^ , (12)
where MdC, is the module of scenarios for У LL generating the need for a service,
Md pu is the module of scenarios for receiving the service.
The module of scenarios for generating the need for a service provides the ability of the User agent to initiate the emergence of the need and desire to receive a specific service. It depends on the personal features of an agent and environmental factors of the agent’s existence:
Md =„ = ^Pr^tA ^ A " ).....
Pr „S“„„ (AC,A ° ))
Using the rules of the module of scenarios for receiving the service, the User agent decides upon the possibility and method of receiving the service and determines its own actions:
Md C u = ^Рг^ЧА5, Ac , Au , AR ),.
P MdCptl fAs дс ди ДТ?Л1
, ■", p, PrMdCpuN VA , А , А , А ))
The mode should have an optimality criterion. It is related to the assessment of the dynamics of changes in the properties of Services agents and User agents:
К = f(d(As ), d(Ac )) , (15)
where f( ) is the function for determining the optimality criterion,
d(x) is the function characterizing the dynamics of the change in parameter X .
The solution of the problem is connected with multiple simulation of situations with different combinations of the location of Services agents in the nodes. Based on the results of each iteration, the value of the optimality criterion is calculated. The obtained values of the criteria are compared. Based on the results of the comparison, the model with the best, in a certain sense, criterion value is selected.
Models of activity of agents of consumers of medical services
One of the tasks that needs to be solved in relation to the proposed model is to implement the rules of the module of scenarios for generating the User agent’s need for a service.
Ronald M. Andersen’s behavioral model (Andersen, 1968) is the basis of most modern concepts describing the behavior of users of medical services. The use of health services is considered as a result of the action of three groups of factors:
– factors characterizing users of health services. Demographic characteristics (gender, age) are considered as biological grounds for the use of certain types and volumes of medical care. Socio-structural parameters (education, profession) determine people’s ability to cope with emerging health-related problems and their ability to manage the available resources. The system of people’s ideas about health, i.e. their values, attitudes, as well as knowledge about the possibilities of medicine and modern healthcare can affect their awareness of the need for medical care and the very process of using medical services.
– enabling factors. They determine the conditions under which it becomes possible to use the services. These factors may include access to health insurance, the ability to pay for services from one’s own resources, and the time spent on receiving medical services.
– need. This group of factors determines the urgency of the need for medical care, measured, as a rule, with the help of a professional medical assessment of health and variables characterizing the perception and awareness of the need for medical care by an individual. The indicators reflecting the feelings of an individual are usually as follows: selfreported health status, ability to function in key types of activities, the level of anxiety concerning various painful symptoms. Their role in the use of medical services largely depends on the social and status-related features of the consumer and their general views concerning health.
Analyzing the relationship between the features that reflect the attitude toward health and illness with the use of medical care makes it possible to better understand in which situations a person considers it necessary to seek medical care and turns to the healthcare system (Rusinova, Panova, 2002).
In addition to the above parameters, the behavioral model also includes the following (Pottick, Hansell, Gutterman, White, 1994; Mirowsky, Ross, 1983; Nichol, Stimmel, Lange, 1995; Katz, 1996; Wennberg, Barnes, Zubkoff, 1982; Lurie, 1993):
– features of the environment in which medical services were received;
– features of the healthcare system (health policy, system resources, organization of medical care);
– features of the use of medical services (type and place of the service received, reasons for and frequency of consulting a doctor);
– characteristics of the environment external to the healthcare system (economic, political and social factors that determine the functioning of the healthcare system and affect the behavior of users of health services). The most important parameters of the external environment, which, as it is generally assumed, can influence the behavior of users of health services and medical professionals, are as follows: economic climate, welfare of population and its individual groups, priority development goals, the severity of stress and violence, and dominant norms in society;
– features of medical professionals and parameters of their interaction with patients.
Not everyone has a proactive attitude toward health preservation; this is due to various factors that affect people’s activity. It often turns out that an individual understands what measures are necessary to maintain good health, but does not take these measures due to certain factors. These factors act as an obstacle to health protection.
Human health depends a lot on the lifestyle, which to a large extent is individual and is determined by historical and national traditions and personal inclinations.
Usually, when studying medical activity, one assesses the completeness and timeliness of seeking medical help and the implementation of medical recommendations, the habit of resorting to selftreatment and receiving help outside the field of official medicine. Confidence in the importance of one’s own efforts to maintain health correlates with the level of education, professional status and self-assessment of income. However, the results of sociological surveys indicate that despite the awareness of the importance of prevention and early diagnosis of diseases, the majority of respondents seek medical help only if there emerge pronounced symptoms of the disease (Pokrovskaya, 2012).
In general, there are three main patterns of citizens’ behavior when they fall ill4:
Table 1 shows one of the possible options for classifying patients in medical institutions according to two types of activity – high and low. The possible variants of forecasts are also indicated here, depending on the current health status and medical activity.
Health status is the basis for seeking medical help. The frequency of visits to doctors correlates to a certain extent with the data on health perceptions. One of the indicators of medical activity is the behavior of patients when they fall ill. Manifestations of medical activity depend on many factors. Personal factors like gender, age, place of residence, education, and social status are mainly distinguished as significant.
We have developed a general scheme of the states of the agent-consumer of medical services (Fig. 1) .
Due to the objective difficulties arising when obtaining real data on the region’s population and the need to use large computing resources in simulation, it is almost impossible to consider every person living in the region as an agentconsumer of medical services. Therefore, we propose an approach, according to which, on the basis of a qualitative and quantitative analysis of the composition of the population of the territory and its spatial location, agents are generated with some frequency throughout the modelling time, and the features of the agents reflect the dynamics of emergence of citizens in need of medical care. The agent being created does not characterize a specific person with a set of inherent health characteristics, but a person who needs a specific medical service at a given time, located in a certain place. In this case, the agent is endowed with a specific set of features (diagnosis, health status, age, gender, social status, etc.), on the basis of which its further behavior is determined. The agent’s state changes throughout its lifetime in the model. To ensure objectivity of what is happening in reality, the model must take into account the changes in the extent of the need for medical services. The state of human health can change both for the better and for the worse. As a result, the need for medical intervention in both
Figure 1. Scheme of the states of the agent-consumer of medical services
Parameters characterizing the population of the territory
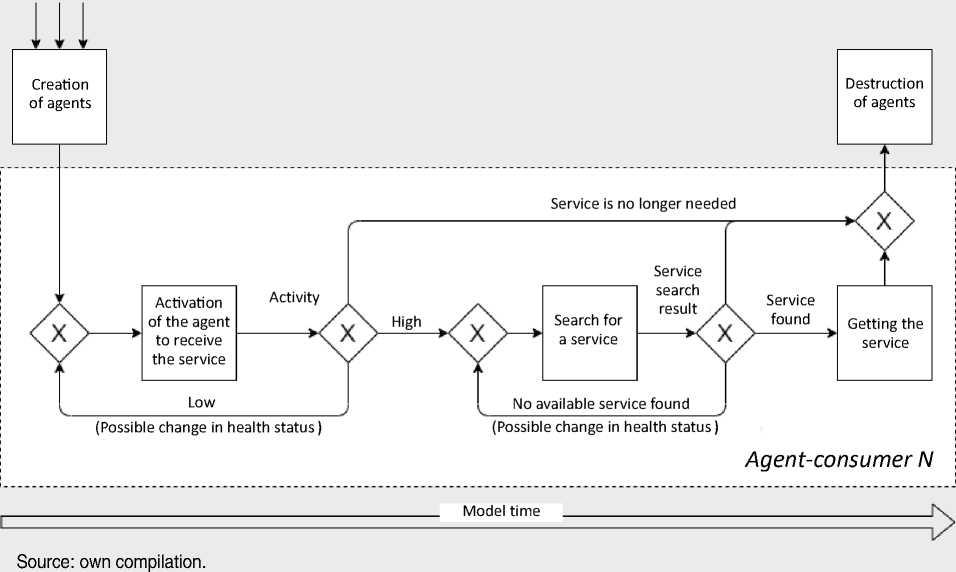
cases may disappear. Agents whose health status parameters have changed to a critical level, as well as agents to whom the service has been provided, are removed from the model. The general sequence of changing agent states is defined as follows. After the agent is generated, it goes into the “Activating the agent to receive a service” state, where the level of its medical activity is determined. When the activity is high, the agent switches to the “Searching for a service” state. If the activity is low, then after a certain interval of the model time, during which the agent’s health status may change, the level of its activity, as well as its health status, is assessed once again. Depending on the results, the agent remains in the current state, switches to the “Searching for a service” state, or is removed from the model. In the “Searching for a service” state, during one simulation step, the agent determines the current availability of the service it requires. If the service is found, the agent switches to the “Receiving the service” state. Otherwise, after a certain interval of the simulation time, during which the agent’s health status may change, the availability of the service and the state of health is determined once again. Depending on the results, the agent remains in the current state, shifts to the “Receiving the service” state, or is removed from the model. The agent stays in the “Receiving the service” state for a certain simulation time for the corresponding type of service, and then is removed from the model.
Next, we present a mechanism we have developed for determining the medical activity of an agent-consumer of medical services who is in the “Activating the agent to receive a service” state. The mechanism is associated with predicting the manifestation of active behavior by a person in their daily life in relation to their health, regardless of the current health status, as a representative of a certain category of citizens with similar behavioral patterns. The most important criterion for the manifestation of medical activity associated with the current stage of the disease will be used in integration with the criterion considered here based on the specifics of the medical services presented in the models. This is due to the fact that a person’s feeling and health perceptions in relation to different diagnoses may differ.
The type of agent activity (high, low) is determined in the “Block for activating the agent to receive a service” on the basis of the generated agent’s parameters (Tab. 2) .
Since it is impossible to determine the agent’s medical activity unambiguously, we use fuzzy neural networks in this case (Gupta, 1994; Shvetsov, Dianov, 2018; Dianov, Shvetsov, 2018). The network implements a zero-order Sugeno fuzzy inference system, has a set of input variables P corresponding to the values of the agent’s parameters. The network output is a variable that determines the activity of an agent with a set of values [0..1], where a value close to 1 determines a high degree of activity.
The network consists of five layers. Layer 1 defines fuzzy terms of input parameters. The outputs of the nodes of this layer represent the values of the membership function at specific values of the inputs. Each node of the layer is adaptive with a membership function iA l (Pj), where Af is a fuzzy term used for linguistic evaluation of the variable Pj . The membership function can be determined on the basis of statistical data analysis and the results of surveys. Thus, it is possible to form various groups of the population that have, in a certain sense, typical behavioral parameters associated with their manifestation of medical activity (Tab. 3) .
In the analyzed survey, activity can be viewed through the prism of two mutually opposite answers to the following questions:
– “What do you usually do when you are feeling unwell?” (answer option: “I try to put up with it, I don’t consult a doctor and I don’t try to find treatment on my own; it will pass, somehow”) – passive.
– “What do you do to preserve and strengthen your health?” (answer option: “I go to the doctor at the first signs of illness, I regularly undergo medical checkup”) – active.
Table 2. Factors related to the manifestation of medical activity
Factor |
Dependence |
Gender |
Women are much more likely to seek medical attention than men. |
Age |
The frequency of visits to a doctor increases in proportion to age, the “leap” occurs after 50 years of age. |
Place of residence |
Place of residence affects the frequency of visits, but the dependence here is not related to the size of the settlement and the provision of medical and preventive institutions, as one might assume. The first place in terms of frequency and regularity of seeking medical care belongs to the population of the towns under the district jurisdiction and workers’ settlements, second – to the residents of the regional center, third – to the rural population, fourth – to the population of the cities and towns under the oblast jurisdiction. |
Education |
Persons with higher education prefer to visit a doctor once or twice a year, persons with secondary and secondary vocational education visit medical institutions somewhat less often, almost a third of them less than once a year; citizens with incomplete secondary and primary education – monthly or semi-annually. |
Social status |
Pensioners rank first in terms of frequency and regularity of visits to medical institutions; public sector workers rank second; engineering and technical workers and employees rank third; workers rank fourth; the unemployed rank fifth; entrepreneurs and merchants rank sixth. |
Compiled according to: Reshetnikov A.V. (2000). Social portrait of the consumer of medical services. Ekonomika zdravookhraneniya , 12, 5–19. |
Table 3. Population groups with typical behavioral parameters associated with their manifestation of medical activity
Parameter |
Groups |
Gender |
- Men; - Women |
Age |
- 18–30 years old (inclusive); - 31–50 years old (inclusive); - from 51 years old |
Place of residence |
- City, town; - Rural area |
Education |
|
Social status |
|
Source: own compilation. |
Table 4. Results of calculation of membership functions’ parameters
Parameter |
Parameter value |
Total |
Active |
Passive |
Undecided |
|||
Amount |
% |
Amount |
% |
Amount |
% |
|||
Gender |
male |
670 |
176 |
26 |
169 |
25 |
325 |
49 |
female |
830 |
311 |
37 |
72 |
9 |
447 |
54 |
|
Age (years) |
18–30 |
267 |
68 |
25 |
58 |
22 |
141 |
53 |
31–50 |
582 |
157 |
27 |
110 |
19 |
315 |
54 |
|
51 and more |
651 |
262 |
40 |
73 |
11 |
316 |
49 |
|
Place of residence |
city, town |
1079 |
349 |
32 |
160 |
15 |
570 |
53 |
rural settlement |
421 |
138 |
33 |
81 |
19 |
202 |
48 |
|
Education |
incomplete secondary, secondary |
132 |
51 |
39 |
37 |
28 |
44 |
33 |
secondary vocational, secondary technical, incomplete higher |
1000 |
309 |
31 |
170 |
17 |
521 |
52 |
|
higher, postgraduate |
368 |
127 |
35 |
34 |
9 |
207 |
56 |
|
Social status |
students |
53 |
12 |
23 |
14 |
26 |
27 |
51 |
workers |
943 |
286 |
30 |
165 |
17 |
492 |
52 |
|
engaged in household chores |
47 |
5 |
11 |
13 |
28 |
29 |
62 |
|
pensioners |
406 |
169 |
42 |
37 |
9 |
200 |
49 |
|
other |
51 |
15 |
29 |
12 |
24 |
24 |
47 |
Own compilation based on the results of the survey of Vologda Oblast residents “Studying public health and its determining factors” conducted in 2020 by Vologda Research Center of the Russian Academy of Sciences.
The percentage of positive answers to these questions can be a source for determining the parameters of membership functions (Tab. 4) . They are differentiated by the values of the parameters of the population groups.
Some of the respondents have no clear relation to the manifestation of medical activity. We assume that they can, with a certain degree of probability, behave either actively or passively in each particular case (the fuzzy term for determining the possibility of activity includes a set {“Active”, May be active”}). Proceeding from this, the corresponding membership functions should be formed (Tab. 5).
Table 5. Membership functions for population group parameters
Parameter |
Parameter value |
Membership function |
|
Active |
May be active |
||
Gender |
male |
^ Л р1 (^') |
H-A P 2 ^^ |
female |
P A T3 (p j) |
P A p4 (Pj) |
|
Age (years) |
18–30 |
Р А .1 (Р)) |
P A . 2 (Pj) |
31–50 |
^Pj) |
P A . 4 (Pj) |
|
51 and more |
^Pj} |
P A . 6 (Pj) |
|
Place of residence |
city, town |
pam(pj) |
Pa^P?) |
rural settlement |
PA^j) |
P a „ 4 (p Z ) |
|
Education |
incomplete secondary, secondary |
P A 01 (P j} |
P A 02 ^P j^ |
secondary vocational, secondary technical, incomplete higher |
Pa^P) |
Pa^P]) |
|
higher, postgraduate |
P A osV j ) |
^ А оЛ^ / ) |
|
Social status |
students |
P A -. vP) |
P a s2 {P j ) |
workers |
P A s3 (P j) |
P A s4 (P j) |
|
engaged in household chores |
P A ss (Pj) |
P A s6 (P j) |
|
pensioners |
P A s7 (P j) |
P A s8 (P j) |
|
other |
P A s9 (P j) |
P Asla (.P j ) |
Own compilation.
The graphs representing membership functions for the values of the “Gender” parameter are shown in Figure 2 . They are based on the percentage ratio (the proportion is determined in the range from 0 to 100) associated with the activity of citizens of the territory. Each attribute can have two functions:
“active” and “may be active”. The points for the graph values are determined based on the following considerations:
– the values at which unconditional activity is manifested are determined (the value of the function is 1). We define it as the percentage of active
1.2
Figure 2. Graph of the membership function for the “Gender” parameter
0.8
0.4
0.2
0.6 Q
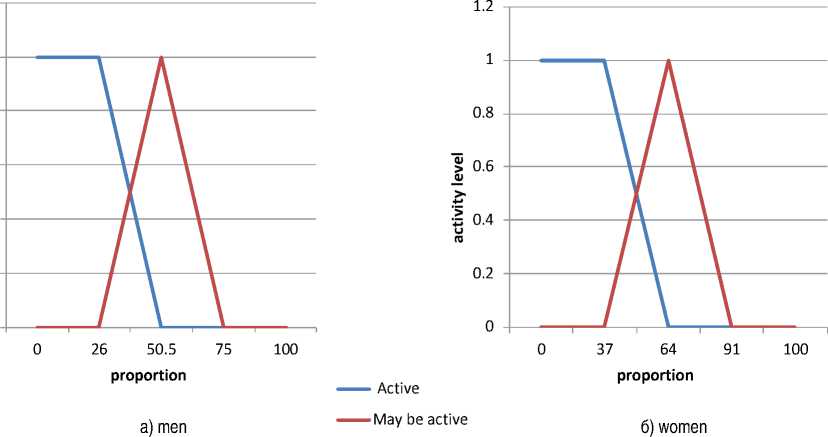
Source: own compilation.
citizens based on the survey results and postpone it from the beginning of the coordinate axis;
– the values at which the unconditional passivity of citizens is manifested are determined (the value of the function is 0). We define it as the percentage of passive citizens based on the survey results and postpone it from the end of the coordinate axis;
– we find the midpoint between the ratio of active and passive citizens.
For the remaining parameters, graphs of membership functions are constructed in the same way. It is worth noting that this order is defined for the initial stage of network construction. In the future, in the process of its training, the membership functions may change.
The membership function is selected depending on the values of the parameters of the person in question (gender, age, place of residence, education, social status):
PA pa
P r Apn
(p , )=[ (p , )=[
P Ap1 (p'),if sex = "male" Pap3(P;), if sex = "female" ' ^ Ap 2 (P ; ),if sex = "male" P Ap4 (p),if sex = "female"
( P A v1 (Pj), if age = "from 18 to 30" PaJJl) = j P AV 3 (P ; ),if age = "from 31 to 50"
IP A „ s (P ; ),if age = "from 51"
(P A „2 (p),if age = "from 18 to 30" Pa^CP) = UM(P),if age = "from 31 to 50"
I Pa^P)^ age = "from 51"
PA ma
P r Amn
(P) = I
(p) = |
PA m1 (P ; ), if place of residence = "city" - PA m3 (P j ), if place of residence = "village"
PA m 2 (P j ), if place of residence = "city" _PA m4 (P ; ),if place of residence = "village"
<PA o1 (P;-), if education = "incomplete secondary, secondary"
P A oa(Pj) = ^
PA o3 (p), if education = "secondary vocational, secondary technical, incomplete higher"
A
p Ao 2 (p),if education = "incomplete secondary, secondary"
P a 0Apj) = '
pA o4 (P ; ),if education = "secondary vocational, secondary technical, incomplete higher"
A
<PA s1 (P;-),if social status = "students" PA s 3 (p"), if social status = "workers"
P A sa(Pj) = ^
PA s5 (p), if social status = "engaged in household chores" PA s7 (P;-),if social status = "pensioners"
uAs9(P;), if social status = "other"
7^2 (P j ), if social status = "students" PA s4 (p'),if social status = "workers"
Pa^J = ^
PA $6 (P j ), if social status = "engaged in household chores"
PA s8 (P j ), if social status = "pensioners"
A
The value of the membership function parameter is determined by generating a random number in the range from 0 to 100.
Layer 2 defines the premises of fuzzy rules. This layer is not adaptive, it performs the product of inputs. The output data of this layer are the degrees of truth of the assumptions of the rules (activity and possible activity):
W 1 = ЦаД1-)* ^а ( Р)* H A ma^ * ^ AO a (P j ) * PaM’
” (17)
W 2 = ЦаД Р )) * Да^РО * M A m„ (p) * P Aop ( P j ) * Да^РУ
Each node of non-adaptive layer 3 calculates the relative degree of fulfillment of the fuzzy rule:
Wi w2
w * =---1— , w * =---2— . (18)
W1 + W2 W1 + W2
Adaptive nodes of the fourth layer calculate the contribution of each fuzzy rule to the network output using the formulas:
Ai = wl^vki, A^ = wVuk2. (19)
Clear numbers vk 1 , vk 2 define the conclusions for each rule.
A single node of layer 5 summarizes the contributions of all rules:
Ac = A^ + Ac2 . (20)
The software implementation of the network is carried out in the Microsoft Access environment. A part of the statistical survey data was imported into the database. Modules were created that implement the algorithm of the fuzzy neural network. An example of the results obtained is shown in Figure 3 .
Currently, a number of experiments have been conducted to determine medical activity of the population using a fuzzy neural network with different values of the configurable parameters of the fourth layer vk 1 , vk 2 , as well as with different levels of activity determination. Individual results are presented in Table 6 .
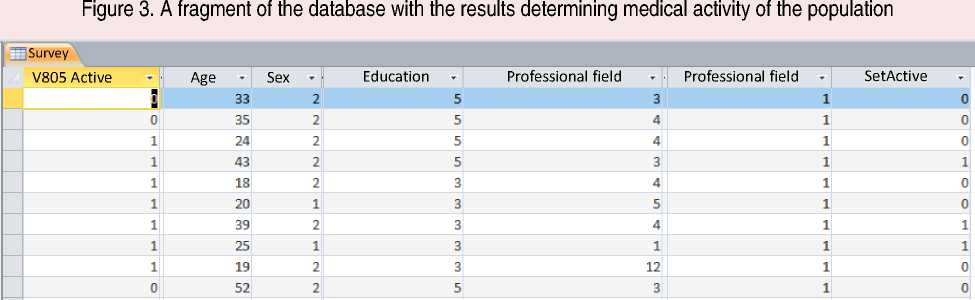
Leftmost column – activity according to the results of the survey, rightmost column – output results. Source: own compilation.
Table 6. Results of experiments to determine medical activity of the population using a fuzzy neural network
No. |
Level to determine medical activity |
vk 1 |
v k 2 |
Number of active citizens |
1 |
0.6 |
1 |
0.1 |
679 |
2 |
0.8 |
1 |
0.1 |
670 |
3 |
0.8 |
0.9 |
0.1 |
647 |
4 |
0.6 |
0.7 |
0.1 |
635 |
5 |
0.5 |
0.6 |
0.3 |
653 |
The results obtained during the experiments in order to verify the accuracy of forecasting can be compared with the results of the sociological survey itself. We considered the option when two values of the activity criterion are used: 1 – active, 0 – inactive. The respondents who chose the option “I go to the doctor at the first signs of illness, I regularly undergo a medical checkup” were determined to be active when answering the question “What do you do to preserve and strengthen your health?” With regard to the results presented in Table 6, the discrepancy with the data of the sociological survey was from 30 to 40% (the number of active users of medical services according to the survey results was 487 out of the total number of 1,500 respondents). When analyzing the data obtained, one should bear in mind that the first layer of the network was not subjected to adaptation. We plan to further refine the presented network in terms of its training and providing an opportunity to take into account the nature, specifics and severity of a medical issue for a person when calculating activity.
Specific features of agent-based modeling determine the prospects of its use for solving a variety of tasks in socio-economic systems. The main difficulty in implementing such models is their conceptualization. The results obtained in the study provide a conceptual basis for the process of constructing agent-based models of the spatial network of infrastructure facilities and resource provision of the regional healthcare system. These models are focused on the search for optimal accommodation options, taking into account the motives, attitudes and behavioral strategies of the residents of the region, as well as the role of the set of external and internal factors determining this choice. It is ensured by the possibility of using the results of sociological surveys. The presented approaches help to interpret survey data for the formation of behavioral models of agents-users of medical services. At the same time, due to the application of fuzzy inference mechanisms, an element of uncertainty in the behavior of agents is simulated, which increases the adequacy of the models in relation to the subject area. Thus, the presented work contributes to the development of theoretical and applied aspects in the creation of agent-based models related to the provision of services for social systems.
Список литературы Agent-based modeling of regional healthcare: addressing the task of formalizing residents' medical activity
- Andersen R.M. (1968). Behavioral Model of Families: Use of Health Services. Chicago: Center for Health Administration Studies, University of Chicago.
- Badham J., Chattoe-Brown E., Gilbert N., Chalabi Z., Kee F., Hunter R. F. (2018). Developing agent-based models of complex health behaviour. Health Place. 54, 170-177. DOI: 10.1016/j.healthplace.2018.08.022
- Bakhtizin A.R. (2008). Agent-orientirovannye modeli ekonomiki [Agent-Based Models of Economy]. Moscow: Ekonomika.
- Dawid H., Neugart M. (2011). Agent-based Models for Economic Policy Design. Eastern Economic Journal, no. 37, 44–50.
- Dianov S.V. (2020). The problem of optimal spatial placement of services for systems with mobile users. In: Materialy odinnadtsatoi zaochnoi mezhdunarodnoi nauchno-tekhnicheskoi konferentsii “Intellektual'no-informatsionnye tekhnologii i intellektual'nyi biznes (INFOS-2020)” (29–30 iyunya 2020 g.) [Proceedings of the Eleventh Virtual International Scientific and Technological Conference “Intellectual and Information Technologies and Intellectual Business (INFOS-2020)” (June 29–30, 2020)]. Vologda: VoGU. Pp. 53–55 (in Russian).
- Dianov S.V., Shvetsov A.N. (2018). Ispol'zovanie nechetkikh neironnykh setei dlya poiska vozmozhnykh stsenariev povedeniya v mul'tiagentnykh sistemakh organizatsionnogo upravleniya. In: Vuzovskaya nauka – regionam. Materialy XVI Vserossiiskoi nauchnoi konferentsii s mezhdunarodnym uchastiem. Vologda. Pp. 77–79 (in Russian).
- Gaffard J.-L., Napoletano M. (2012). Agent-based Models and Economic. Paris: OFCE. 448 p.
- Gupta M.M. (1994). Fuzzy neural networks: theory and applications, Proc. SPIE 2353, Intelligent Robots and Computer Vision XIII: Algorithms and Computer Vision. Available at https://doi.org/10.1117/12.188903
- Katz B.P., Freund D.A., Heck D.A., Dittus R.S., Paul J.E., Wright J., Coyte P., Holleman E., Hawker G. (1996). Demographic variation in the rate of knee replacement: a multi-year analysis. Health Services Research, 31(2), 125–140.
- Lurie N., Slater J., McGovern P., Ekstrum J., Quam L., Margolis K. (1993). Preventive care for women. Does the sex of the physician matter? N Engl J Med., 12, 329(7), 478–482. DOI: 10.1056/NEJM199308123290707
- Makarov V.L., Bakhtizin A.R., Sushko E.D. (2016). Agent-based models as a means of testing of management solutions. Upravlencheskoe konsul'tirovanie=Administrative Consulting, 12, 16–25 (in Russian).
- Makarov V.L., Bakhtizin A.R., Sushko E.D., Sushko G.B. (2019). Agent-based supercomputer demographic model of Russia: approbation analysis. Ekonomicheskie i sotsial'nye peremeny: fakty, tendentsii, prognoz=Economic and Social Changes: Facts, Trends, Forecast, 12(6), 74–90. DOI: 10.15838/esc.2019.6.66.4 (in Russian).
- Mendritzki S. (2010). Artificial Policy: Examining the Use of Agent-Based Modeling in Policy Contexts. M.S. Thesis. Alberta: University of Calgary. 130 p.
- Mikhailova Yu.V., Leonov S.A., Son I.M. et al. (2007). Current state and directions of development of national medical statistics. Sotsial'nye aspekty zdorov'ya naseleniya=Social Aspects of Population Health, 1(1). Available at: http://vestnik.mednet.ru/content/view/21/30 (accessed: November 10, 2021; in Russian).
- Mirowsky J., Ross C.E. (1983). Patient satisfaction and visiting the doctor: A self-regulating system. Social Science & Medicine, 17(18), 1353–1361. Available at https://doi.org/10.1016/0277-9536(83)90195-8
- Nichol M.B., Stimmel G.L., Lange S.C. (1995). Factors predicting the use of multiple psychotropic medications. The Journal of Clinical Psychiatry, 56(2), 1–66.
- Pokrovskaya S.E. (2012). Factors forming medical aid appealability. Sotsial'nye aspekty zdorov'ya naseleniya=Social Aspects of Population Health, 25(3), 2 (in Russian).
- Pottick K., Hansell S., Gutterman E., White H.R. (1995). Factors associated with inpatient and outpatient treatment for children and adolescents with serious mental illness. Journal of the American Academy of Child & Adolescent Psychiatry, 34(4), 425–433. Available at https://doi.org/10.1097/00004583-199504000-00009
- Rusinova N.L., Panova L.V. (2002). Access to health services: Methodological approaches and measurement methods. Zhurnal sotsiologii i sotsial'noi antropologii=Journal of Sociology and Social Anthropology, 5(4), 147–163 (in Russian).
- Shvetsov A.N., Dianov S.V. (2018). Using fuzzy neural networks to determine reactive behavior in multi-agent organizational management systems. Vestnik Vologodskogo gosudarstvennogo universiteta. Seriya: Tekhnicheskie nauki=Bulletin of Vologda State University. Series: Technical Sciences, 1(1), 64–69 (in Russian).
- Silverman B.G. (2014). Systems approach to healthcare: Agent-based modeling, community mental health, and population well-being. Artificial Intelligence in Medicine, 63(2). DOI: 10.1016/j.artmed.2014.08.006
- Tracy M., Cerdá M., Keyes K.M. (2018). Agent-based modeling in public health: Current applications and future directions. Annual Review of Public Health, 39, 77–94. Available at: https://doi.org/10.1146/annurev-publhealth-040617-014317
- Wennberg J.E., Barnes B.A., Zubkoff M. (1982). Professional uncertainty and the problem of supplier-induced demand. Social Science & Medicine, 16(7), 811–824.