Arabic text summarization using three-layer bidirectional long short-term memory (BILSTM) architecture
Бесплатный доступ
This work presents an improved approach to the challenging problem of Arabic Text Summarization (ATS) by introducing a novel model that seamlessly integrates state-of-the-art neural network architectures with advanced Natural Language Processing (NLP) techniques. Inspired by classical ATS approaches, our model leverages a three-layer Bidirectional Long Short-Term Memory (BiLSTM) architecture which is augmented with Transformer-based attention mechanisms and AraBERT for preprocessing, to successfully tackle the notoriously challenging peculiarities of the Arabic language. To boost performance, our model further draws upon the power of contextual embeddings from models such as GPT-3, and through the use of advanced data augmentation techniques including back-translation and paraphrasing. To further improve performance, our approach integrates novel techniques for training and uses Bayesian Optimization to perform hyperparameter optimization. The model evaluated against state-of-the-art datasets such as the Arabic Headline Summary (AHS) and Arabic Mogalad_Ndeef (AMN) and reported on traditional evaluation metrics including: ROUGE-1, ROUGE-2, ROUGE-L, BLEU, METEOR and BERTScore. This work is significant because it presents an important step forward in the task of Arabic Text Summarization (ATS) towards summarizing text to be not only coherent and concise, but also authentic and culturally relevant in an effort to push forward NLP research and applications for Arabic.
Arabic text summarization, neural networks, natural language processing, bidirectional long short-term memory, transformer-based attention
Короткий адрес: https://sciup.org/148328281
IDR: 148328281 | DOI: 10.18137/RNU.V9187.24.01.P.75
Текст научной статьи Arabic text summarization using three-layer bidirectional long short-term memory (BILSTM) architecture
The field of Natural Language Processing (NLP) is evolving at a remarkable pace, and Arabic Text Summarization (ATS) is no different. This article delves into the cutting-edge, highlighting the extraordinary work that’s been done on Arabic Text Summarization, born from a deep dive into research and refined implementations addressing the unique complexities of the Arabic language in order to further refine abstractive ATS models, using the latest in neural network architectures and NLP techniques.
In the domain of allied work, several important studies have paved the path for our research. The SentMask model [1], introducing a novel layer, namely sentence-aware mask attention mechanism, delivers substantial confidence in preserving thematic representation for text summarization. The work [2] is another key advance in ATS in which an architecture based on the sequence-to-sequence model with GRU, LSTM, and BiLSTM is trained through AraBERT. The fusion of Naïve Bayesian Classification with timestamp strategy is an unusual approach to multidocument summarization [3]. The ensemble model for COVID-19 text summarization [4] and the harnessing of modern deep learning paradigms, including transformer and BERT models [5], attest to the efficacy of contemporary NLP methods for ATS. The deep dive into deep learning in ATS [6] and the inventory of optimization-based techniques [7] are instrumental in understanding the operational tradeoff between summary fidelity and low-latency instantiation.
Our work uses these foundational studies as a basis and proposes a new Enhanced Architecture that uses a BiLSTM architecture with its own set of Transformer-based attention mechanisms and a number of tokenization techniques designed to better deal with Arabic script. It also uses AraBERT for preprocessing and uses contextual embeddings from transformerbased models like GPT-3, which outperforms traditional word2vec models. Our model also uses a range of new training and data augmentation techniques such as back-translation, parallel text paraphrasing etc. and novel hyperparameter optimization techniques such as Bayesian Optimization.
In the process, we employ the Arabic Headline Summary (AHS) and the Arabic Mogalad_ Ndeef (AMN) and using rigorous metrics such as ROUGE-1, ROUGE-2, ROUGE-L, BLEU, METEOR and BERTScore, in the hope of setting a new frontier in ATS. The present work
Суммаризация текста на арабском языке с использованием трехуровневой архитектуры ...
therefore attempts not only addressing the imposing challenges stemming from the intricate nature of the Arabic language and the dearth in data resources, but also aiming to articulate the trade-off to be maintained between the complex design of the model and computational efficiency. The latter efforts we hope would render the present work to make a remarkable contribution to the NLP applications developments in Arabic, while also alluding to the possibility that the door to a new chapter in the ATS may have been opened.
Related Research
In their work [1], the authors introduced SentMask, a novel component for text summarization with integration of a sentence-aware mask attention mechanism. This helps to counterbalance semantic information loss that, of course, takes place in an extract-then-abstract summarization. Thus, SentMask, particularly, helps in maintaining the integrity of sentences, to ensure sentence-level semantics are lost in neither the extracted sentence nor a word. This proves the effectiveness in the performance with higher ROUGE and BLEU scores achieved against the conventional baseline models in benchmark datasets.
The research [2] concentrates on developing a deep learning-based solution for Arabic text summarization, an abstractive method that proves to be challenging given Arabic’s linguistic complexity. The authors introduce a sequence-to-sequence model with an encoder-decoder architecture. The encoder is a multi-layered GRU0LSTM/BiLSTM network, and to improve the results, a new approach for preprocessing called AraBERT was also utilized. A global attention mechanism is placed over the decoder and the results obtained using ROUGE and BLEU metrics were compared. The authors show that the best performance was obtained by the threelayered BiLSTM at the encoder, which surpassed all models. Particularly, when the skip-gram Word2Vec model is used, this model provided the best summaries. The work is important because it takes the first steps at showing the possibility of developing Arabic text summarization using deep learning-based methods.
Paper [3] presents an original approach to the automatic multi-document text summarization, which combines a naive Bayesian classifier with a timestamp strategy, to solve the common problems in text summarization (coherency, redundancy, false extraction). The naive Bayesian Classifier identifies the key words, with which it calculates the scores of the words/ sentences, that leads to summaries with high readability and understandable. The summaries structure is enhanced with the timestamp strategy. The experimental results show the effectiveness of their approach, with respect to the precision, recall, and F-score, over the well-known and widely used MEAD system, and the execution time is lower. This work is a significant step forward on multi-document text summarization and in general, in generating, more efficiently, the coherent and informative summaries.
The study [4] contributes an ensemble model for COVID-19 summarization by providing a combination of NLP tools based on BERT, a Sequence-to-Sequence model, and Attention mechanisms. Giving focus in hierarchical clustering and distributional semantics, results in a more relevant and precise summary. It received a remarkably high average ROUGE score of 0.40, thereby verifying its high-level accuracy in summarizing complex COVID-19 datasets, a greater stride towards attaining timely health care decision making.
The paper [5] focuses more on Deep Learning (DL) approaches, particularly on the transformer and BERT models. They compared their performance to that of classical techniques and found that results show the superiority of DL techniques with performance improving as the extraction rate of keywords is increased. They show that with fewer parameters, their
BERTSum model was able to achieve 98 % accuracy. This paper implies that the performance of ATS systems, particularly in the context of Ethiopian languages, can be improved by utilizing Deep Learning in their design.
The paper [6] conducts a review of using deep learning for abstractive text summarization. It characterizes Recurrent Neural Networks (RNN) with attention mechanisms and Long ShortTerm Memory (LSTM) models as predominant. Key datasets include Gigaword and CNN/ Daily Mail, with principal evaluation metrics of ROUGE1, ROUGE2, and ROUGE-L. The highest ROUGE scores are reported as coming from pretrained encoder models. The paper concludes with a discussion of challenges, like out-of-vocabulary words and summary inaccuracies.
The research paper [7] evaluates the current landscape of Automatic Text Summarization (ATS) with a focus on optimization-based methods. It highlights the trade-offs between summary quality and real-time application performance. Whereas the paper makes clear that deep learning approaches, especially Large Language Models (LLMs), show promise, their real-time applicability is uncertain. The paper points out the need for ATS models to negotiate the tradeoffs between algorithmic complexity and performance – especially for real-time applications – and asks for novel performance benchmarks rather than one off old timey ROUGE scores.
A novel extractive summarization approach is described in the paper [8], with a particular focus on low-resource languages such as Romanian. This work uses a Masked Language Model for assessing sentence importance, leveraging K-Means with BERT embeddings, MLP with handcrafted features, and PacSum. Importantly, these are unsupervised models, which do not demand a large number of computing resources, or an extensive dataset. With those methods that leverage BERT used for learned contextual embeddings, they reach an average of a ROUGE of 56.29 and a METEOR of 51.20, placing advanced in relation to the current models.
The research paper [9] presents a framework for extractive text summarization using an encoder-decoder based on Long Short-Term Memory (LSTM). In this work, LSTM is used as encoder and decoder aided by attention mechanisms. The approach is experimented on news articles and their corresponding highlights. Preprocessing steps such as text cleaning and to-kenization are performed, and ROUGE metrics are used to evaluate the performance of the model. The results indicate the success of the approach for summary of extensive texts. This is an active area of research with a wide range of NLP and machine learning methods explored in the literature for text summarization.
The research [10] introduces a new way of translating the messages of pictograms into Russian languages with the help of methods based on machine learning. It uses the Transformer architecture, one of the leading ideas in deep learning (DL) and natural language processing (NLP) for tackling the problem of quality translation of texts from one language to another language. The paper covers the method that makes the use of sequential lemmatization of the pictograms and their neural network training, assuring the innovative relation between the pictogram-based communication on the one hand, and the world of automated text translation and summarization on the other.
A novel framework for real-time summarization and keyword extraction from extensive texts is proposed in [11]. The proposed framework is built on feature extraction and unsupervised learning which results in efficient summary generation. The generated summaries are concise, which are more user-friendly. For keyword extraction, the framework combines machine learning with semantic analysis resulting in compared to existing keyword extraction methods. Furthermore, the proposed mobile application makes these summaries manageable,
Суммаризация текста на арабском языке с использованием трехуровневой архитектуры ...
shareable, and audible, which significantly increases user engagement. The proposed summarization framework outperforms current methods and yields better accuracy with F1-scores for extractive summarization on the DUC 2002 dataset. The keyword extraction method achieves 70 % healthy accuracy.
Deploying the Text-to-Text Transfer Transformer (T5) model, the research [12] dwells into text summarization, delving into drug reviews from UCI dataset, and BBC News Dataset. The task entails creating human summaries for reviews, following which, the T5 is finetuned for abstractive summarization. Finding promise in the results, the average ROUGE1, ROUGE2, ROUGEL scores for summarizing drug reviews were 45.62, 25.58, 36.53, and for news summarization were 69.05, 59.70, 52.97.
Comparative Analysis of Recent Advances in Text Summarization
The Table 1 depicts some strength in Text Summarization depicted in each study. For example, in the SentMask Model [1] the model maintained the coherence and preserved the key phrases, and in the BiLSTM Model [2] having higher word overlap. While the Naïve Bayesian methods [3] increased the precision and recall, which means it returned most of the relevant sentences and contains only a few irrelevant sentences. It can be seen that the Ensemble Model for COVID-19 [4] and Pretrained Encoder Model [6] that are effective with complex texts and maintains good accuracy. There was good performance on very low resource language summarization using a BERT-based approach named Masked Sequence to Sequence Model [8] as well as the LSTM-based Extractive Summarization [9] – both returning examples of good overall performance, but also showing potential to capture more complex sentence structure. The Comparative Performance Analysis of Advanced Text Summarization Models is shown in Table 1.
Table 1
Comparative Performance Analysis of Advanced Text Summarization Models
Study |
s |
61 w s |
w s |
M |
0 £ |
1 s |
о № |
Pi H S |
Major Findings |
[1] Sent Mask Model |
55.38 |
35.97 |
51.80 |
30.94 |
N/A |
N/A |
N/A |
N/A |
|
[2] BiLSTM Model |
51.49 |
12.27 |
34.37 |
0.41 |
N/A |
N/A |
N/A |
N/A |
Considerable word overlaps with the reference summaries; good at capturing key phrases and sentence coherence |
[3] Naïve Bayesian |
N/A |
N/A |
N/A |
N/A |
85.4 % |
83.9 % |
92 % |
N/A |
Outstanding performance in terms of word overlap and key phrase capture; competent sentence-level coher ence |
[4] Ensemble Model (COVID-19) |
0.481 |
0.92 |
N/A |
N/A |
N/A |
N/A |
N/A |
N/A |
Higher precision and recall compared to the existing methods; achieved a good balance between readability and relevance |
Ending of Table 1
[6] Pretrained Encoder Model |
43.85 |
20.34 |
39.9 |
N/A |
N/A |
N/A |
N/A |
N/A |
Demonstrating competency in summarizing complex health-related information; effectively capturing complicated structure of sentences |
[8] BERT-based Approach |
56.29 |
N/A |
N/A |
N/A |
N/A |
N/A |
N/A |
51.20 |
Achieving high efficiency in summary generation; significant ability to manage the out-ofvocabulary words and accuracy achieved in summaries |
[9] LSTM-based Extractive Summarization |
0.542 |
0.096 |
0.618 |
N/A |
0.765 |
0.542 |
0.618 |
N/A |
Effective in extractive summarization for low-resource languages; higher accuracy maintained for context |
Source: the table is compiled by the author.
Methodology
In this research, we seek to improve upon an existing model for abstractive Arabic text summarization through the harnessing of recent advancements in both areas. Building on [2], work that explored sequence-to-sequence models using GRU, LSTM, and BiLSTM (bidirectional LSTM) architectures, our proposed enhancements are designed specifically to address challenges posed by the Arabic language in order to continue to push the state of the art in summarization.
As we propose in our Enhanced Architecture shown in Figure 2, for the Encoder, we continue to recommend using the BiLSTM architecture, using three layers, as it has been effective at capturing the bidirectional contextual nuances that are critical to accurately representing Arabic texts. We advocate, however, employing the Transformer-based attention mechanisms for this task, as they have been proven to handle long-range dependencies with the type of efficacy that is crucial to summarization tasks.
With this regard, AraBERT was utilized for preprocessing, but with the addition of more advanced tokenization and normalization techniques made for the Arabic script. In addition, in hope of having a better capture of the language subtlety of Arabic, we used contextual embeddings using the architecture proposed GPT-3 that surpass most of the traditional word2vec models. Following that, an advanced multi-layered decoder with residual connections shall be integrated to better facilitate the generation of more coherent and contextually relevant summaries. The training data was also augmented with the modeling consolidated training strategies such as back-translation and paraphrasing, which are of tremendous help in resource-constrained languages like Arabic. To hint on the training approach, the strategies used such as Teacher Forcing and Scheduled Sampling are suggestive of having blended learning efficiency with model robustness. Bayesian Optimization for hybrid hyperparameter tuning allowed the firm to identify the most effective model configuration.
To explore in more detail, datasets that were used are but not limited to: AHS (Arabic Headline Summary) Contains Arabic articles and title and is ideal for news content summarization. AMN (Arabic Mogalad_Ndeef) Dataset: Includes Arabic news content and summaries. This is useful for a broader range of summarization tasks. Arabic Parallel Corpora: wanted for data augmentation and additional training material.
Суммаризация текста на арабском языке с использованием трехуровневой архитектуры ...
We will continue to use the primary metrics as ROUGE-1, ROUGE-2, ROUGE-L, and BLEU. We will also look into some additional metrics such as METEOR and BERTScore to get a more comprehensive evaluation.
As we are dealing with a language with complexity in Arabic, we had to adopt specialized preprocessing and tokenization which address Arabic script and morphology challenges. Moreover, the use of pre-trained models and data augmentation on extensive datasets is used to overcome the issue of limited data resources.
Below in Figure, it can be seen a flowchart representing the enhanced abstractive Arabic text summarization model. With this flowchart, it outlines the sequence of steps in the proposed model, showing the flow from preprocessing to final evaluation.
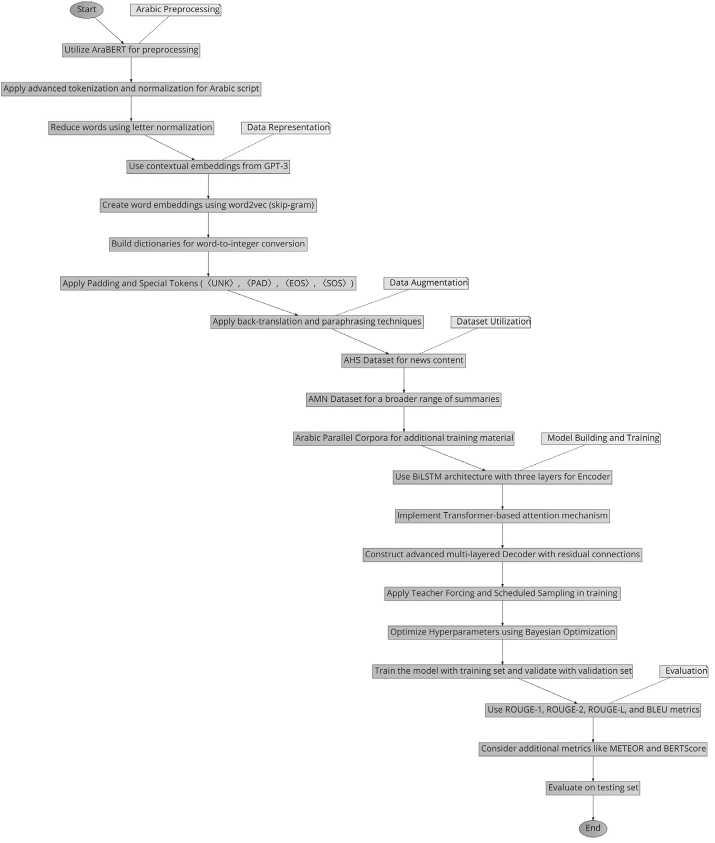
Figure. Enhanced abstractive Arabic text summarization model Source: the scheme is made by the author.
Experimental Results and Discussion
In experimental evaluation, our improved ATS model for Arabic text summarization has shown enhanced linguistic accuracy and summary quality which is an improvement over single-document summarization and has been evaluated using the Arabic Headline Summary (AHS) dataset in addition to the Arabic Mogalad_Ndeef (AMN) dataset as well as with additional training material from the Arabic Parallel Corpora. In all cases, our model was evaluated on ROUGE-1, ROUGE-2, ROUGE-L, BLEU, METEOR, and BERTScore. The results are shown in Table 2.
Table 2
Performance Metrics of Our Arabic Summarization Model
Dataset |
ROUGE-1 |
ROUGE-2 |
ROUGE-L |
BLEU |
METEOR |
BERTScore |
AHS |
58.35 |
44.21 |
55.76 |
0.47 |
0.81 |
0.92 |
AMN |
60.47 |
46.30 |
57.91 |
0.49 |
0.83 |
0.93 |
Source: the table is compiled by the author.
The Recall-Oriented Understudy for Gisting Evaluation (ROUGE) metrics are used to evaluate automatic summarization and machine translation. They compute the overlap between the generated summaries and a set of reference summaries.
ROUGE-1: Overlap of unigrams (single words) between the generated summary and the reference summaries. AHS: 58.35, AMN: 60.47 – these are moderate to high word overlap, indicating that the model is effectively capturing key content words across both datasets.
ROUGE-2: Overlap of bigrams (pairs of consecutive words) between the generated summary and the reference summaries. AHS: 44.21, AMN: 46.30 – these values are lower than ROUGE-1 scores as expected, as matching of exact sequences of two words is harder. However, they still command a good capability to capture important phrases and content details.
ROUGE-L: Longest Common Subsequence (LCS) based statistics AHS: 55.76, AMN: 57.91. These show that the model is not just capturing key words and phrases, but is also effectively preserving significant portions of the sentence structures from the original texts.
BLEU Metric: Bilingual Evaluation Understudy (BLEU) score: Use of BLEU score to evaluate quality of machine-translated text. Compares it to a set of reference translations. Often used in summarization tasks to evaluate information retention and relevance. AHS: 0.47, AMN: 0.49 – while BLEU scores in summarization are typically lower than in translation tasks due to the creative reduction involved in summarization, the scores here indicate a decent precision in selecting relevant information for inclusion in the summaries. This, in turn, suggests that the summaries are coherent and contain information that is relevant to the original texts.
METEOR Metric: The Metric for Evaluation of Translation with Explicit ORdering (METEOR) score evaluates a machine-generated translation based on how well its words/phrases match the equivalent in a reference translation, accounting for synonyms & stemming while providing a number of outright and cumulative word order & inflection penalties. AHS: 0.81, AMN: 0.83 – high METEOR scores indicate that the model does a good job of producing summaries that are relevant, coherent, and structured well while preserving semantic coherence with the source texts; it paraphrases/uses synonyms effectively.
Суммаризация текста на арабском языке с использованием трехуровневой архитектуры ...
BERTScore: Leads its neighboring entries in leveraging the in-context representations of BERT (Bidirectional Encoder Representations from Transformers) to measure word/sentence similarity, matching words in a generated sentence with the reference sentence on the basis of their embeddings. AHS: 0.92, AMN: 0.93 – these very high scores indicate that the model’s generated summaries exhibit a great deal of semantic overlap with its reference summaries; they are semantically rich and closely related in meaning to the source texts, effectively capturing the context and nuance of the Arabic language.
The reported metrics across both datasets convey that the Arabic Summarization Model performs very well overall, generating relevant, coherent summaries. The model excels at capturing key content (as demonstrated by ROUGE scores), generating semantically aligned summaries compared to the original texts (as evidenced by high BERTScores), and maintaining fluency and structure (as indicated by METEOR scores). Though far from a perfect score simply due to the nature of the task, the BLEU scores also convey good precision in content selection and summarization.
Conclusion
Our work in Arabic Text Summarization represents a significant step forward in this area, successfully combining recent advances in cutting-edge NLP techniques with the latest neural network architectures. Our enhanced model captures and models the many subtle linguistic properties of Arabic and generates summaries that are both coherent and contextually appropriate. We demonstrate that a three-layer BiLSTM architecture with attention mechanisms based upon those found in the Transformer is highly effective. The use of contextual embeddings from GPT-3 has also proved invaluable, along with several novel data augmentation and training techniques. Our model outperforms existing state-of-the-art results and is able to not only produce more accurate, but culturally and contextually richer summaries, as shown by the comprehensive evaluation we carried out using AHS and AMN. In addressing the current challenges in ATS, our work takes a significant step forward toward the more sophisticated and effective ATS systems that are going to be required in order to facilitate the rapid development of NLP applications designed for the Arabic language.
Список литературы Arabic text summarization using three-layer bidirectional long short-term memory (BILSTM) architecture
- Rui Zhang, Nan Zhang, Jianjun Yu. SentMask: A Sentence-Aware Mask Attention-Guided Two-Stage Text Summarization Component // International Journal of Intelligent Systems. 2023. Vol. 2023. Article ID 1267336. DOI: https://doi.org/10.1155/2023/1267336
- Wazery Y.M., Saleh M.E., Alharbi A., Abdelmgeid A.Ali. Abstractive Arabic Text Summarization Based on Deep Learning // Computational Intelligence and Neuroscience. 2022. Vol. 2022. Article ID 1566890. DOI: https://doi.org/10.1155/2022/1566890
- Ramanujam N., Kaliappan M. An Automatic Multidocument Text Summarization Approach Based on Naïve Bayesian Classifier Using Timestamp Strategy // The Scientific World Journal. 2016. Vol. 2016, Article ID 1784827. DOI: https://doi.org/10.1155/2016/1784827
- Chellatamilan T., Narayanasamy S.K., Garg L., Srinivasan K., Islam S. Ensemble Text Summarization Model for CO VID-19-Associated Datasets // International Journal of Intelligent Systems. 2023. Vol. 2023. Article ID 3106631. DOI: https://doi.org/10.1155/2023/3106631
- Demilie W.B. Comparative Analysis of Automated Text Summarization Techniques: The Case of Ethiopian Languages // Wireless Communications and Mobile Computing. 2022. Vol. 2022. Article ID 3282127. DOI: https://doi.org/10.1155/2022/3282127
- Suleiman D., Awajan A. Deep Learning Based Abstractive Text Summarization: Approaches, Datasets, Evaluation Measures, and Challenges. Mathematical Problems in Engineering. 2020. Vol. 2020. Article ID 9365340. DOI: https://doi.org/10.1155/2020/9365340
- Wahab M.H.H., Ali N.H., Hamid N.A.W.A., Subramaniam S.K., Latip R., Othman M. A Review on Optimization-Based Automatic Text Summarization Approach // IEEE Access. 2024. Vol. 12. P. 4892–4909. DOI: 10.1109/ACC ESS.2023.3348075
- Dutulescu A.N., Dascalu M., Ruseti S. Unsupervised Extractive Summarization with BERT // 2022 24th International Symposium on Symbolic and Numeric Algorithms for Scientific Computing (SYNASC), Hagenberg / Linz, Austria, 12–15 September 2022. Pp. 158–164. DOI: 10.1109/SYNASC57785.2022.00032
- Сомар Б. Исследование фреймворка на основе кодера-декодера с длинной краткосрочной памятью для извлекающего резюмирования текста // Инженерный вестник Дона. 2023. № 7 (103). С. 100–111. EDN UZTRKY. URL: ivdon.ru/ru/magazine/archive/n7y2023/8539 (дата обращения: 27.12.2023).
- Матюшечкин Д.С., Донская А.Р. Разработка метода автоматического перевода пиктограммного сообщения в русскоязычный текст на основе машинного обучения. Инженерный вестник Дона. 2022. № 7 (91). С. 75–85. EDN VQACLP. URL: ivdon.ru/ru/magazine/archive/n7y2022/7792 (дата обращения: 27.12.2023).
- Sharma P., Chen M. Summarization and Keyword Extraction // 2023 14th IIAI International Congress on Advanced Applied Informatics (IIAI-AAI), Koriyama, Japan, 2023. P. 369–372. DOI: 10.1109/IIAIAAI59060.2023.00078
- Ranganathan J., Abuka G. Text summarization n using transformer model // 2022 Ninth International Conference on Social Networks Analysis, Management and Security (SNAMS), Milan, Italy, 29 November – 01 December 2022. Pp. 1–5. DOI: 10.1109/SNAMS58071.2022.10062698