Comparison of spectral-spatial classification methods for hyperspectral images of high spatial resolution
Автор: Melnikov Pavel V., Pestunov Igor A., Rylov S.A.
Журнал: Журнал Сибирского федерального университета. Серия: Техника и технологии @technologies-sfu
Статья в выпуске: 6 т.10, 2017 года.
Бесплатный доступ
This paper reviews three methods of spectral-spatial classification for hyperspectral images of high spatial resolution: 1) pixelwise classification with post-filtering of resulting class map; 2) spectral-spatial classification based on geometric moments; 3) spectral-spatial classification based on segmentation. The paper provides the results of experimental comparison of these methods. The experiments are based on classification of images obtained by airborne hyperspectral sensor.
Hyperspectral images, local context, spectral-spatial classification
Короткий адрес: https://sciup.org/146115248
IDR: 146115248 | DOI: 10.17516/1999-494X-2017-10-6-805-811
Текст научной статьи Comparison of spectral-spatial classification methods for hyperspectral images of high spatial resolution
Description of the HSI classification methods
The first classification method used in the experiments is described in detail in [4]. The method consists of pixelwise classification of the HSI and then spatial filtering of the resulting classified image with Majority Filter (MF). Each pixel is assigned a class to which the majority of pixels in a predetermined surrounding area belong. For pixelwise classification, method of Maximum Likelihood (ML) and Support Vector Machine (SVM) were used.
The second and third classification methods are based on the use of information about image texture. There is no universally accepted definition of texture but in the area of multi- and hyperspectral imagery, texture of an object can be interpreted as the characteristic of the distribution of spectral brightness vectors of the image region occupied by an object, which is caused by the regular arrangement of non-uniform elements of the object.
The second method of classification consists of extracting textural features by using geometric moments and subsequent classification of obtained feature vectors. Geometric moments are widely used to determine the textural characteristics of the objects on monochrome images [5]. Geometrical moment
MN mp,q of the order p, q of the digital image I(i, j) (with size M × N) is defined as mp,q = EE ipjql (i, j).
i - I j - I
In the area of texture analysis geometric moments are calculated for a window (of size l × l ) surrounding the pixel in question. For hyperspectral images calculation of moments for multiple sets of p , q significantly increases the number of features, thereby only intensifying the “curse of dimensionality”. Therefore, in this study only one moment m 0,0 was used, which is the sum of the spectral brightnesses of pixels in the window of size l × l (in this case for each pixel the feature value is determined by the formula Avg ( i, j ) = m 0 0 ( i, j ) [l 2). As in the first method, the classification of resulting feature vectors was performed by ML and SVM methods.
The third method of classification is described in [6] and is based on the pre-segmentation of HSI based on spectral features. The basic idea of this method is as follows. Using only spectral features for texture classification will lead to a fragmented noisy classified image. However, in a given area of the image covered by one object the percentage of pixels of different clusters will approximately be the same while for different objects this characteristic will differ. This pattern holds for most of the textures corresponding to objects of natural origin (e.g. forest, swamp, tundra). This approach has been successfully used for textural segmentation of multispectral images based on grid clustering algorithms [7]. The advantage of this method is that it does not require a large amount of training samples; it is sufficient to provide only a few samples for each class.
Experimental results
In the experiments two images with sizes of 600×420 and 1000×350 pixels were used. Each image contained 87 spectral channels in the range 404–1016 nm. RGB composites of the images are shown in Fig. 1 a and 2 a . The spatial resolution was around 1 m. The images show areas of Savvatevskoe forestry in Tver Oblast region.
Ground-truth reference maps obtained from the surveys of forest taxation were available for areas that are presented on these images. The ground-truth maps contained classes corresponding to species and age composition of forest stands. However, reference maps were several decades older than – 807 –
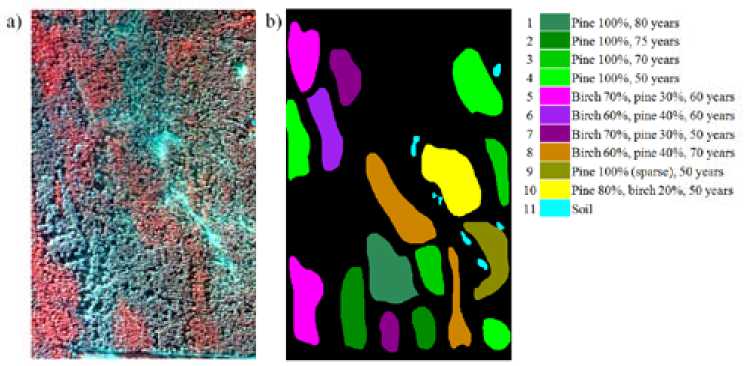
■ Нас 1004. 80 yean
Нас 1004,75 years ^■нпс 10056,70 усап В Нае 1004, 50 yean ■l)wli V». plot 30”». 60 yean ■ Hindi 604, pine 404 60 years В irvii 70*». pine 304, 50 years ^HBirrh60*a. pine404. 70 years В Нас 1004 (sparse), 50 yein
Hoc 80* к birch 204. 50 yean
*6 ill
Fig. 1. RGB-composite (channels 81, 19, 10) (a) and reference map (b) of image 1
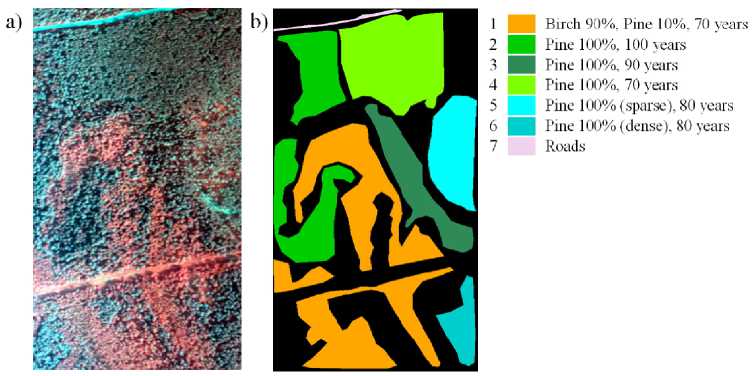
| Birch 90%, Pine 10%, 70 years
| Pine 100%, 100 years
| Pine 100%, 90 years
Pine 100%, 70 years
Pine 100% (sparse), 80 years
| Pine 100% (dense), 80 years Roads
Fig. 2. RGB composite (channels 81, 19, 10) (a) and reference map (b) of image 2
the images, so reinterpretation by visual analysis of the images was performed by experts (resulting reference maps are presented in Fig. 1 b and 2 b ). Doubtful pixels on the boundaries of the classes were assigned to the background (shown as black) and were not taken into account in the assessment of classification accuracy.
Control samples were used to assess the quality of the classification. 1000 randomly selected points of each class were used for training of classifiers. The classification results were averaged over five independent runs (with different training sets). We used the majority filter (MF) with window size of 5×5 pixels, and for calculating Avg ( i , j ) texture features we used a window of 21×21 pixels.
The results of classification using different sets of features and classification methods are shown in Fig. 3 and 4. For comparison, the figures also include the accuracy of pixelwise classification based only on spectral features. The accuracy of the spectral-textural segmentation-based classification of image 2 is shown in Figure 5. First 4 principal components were used as spectral features in this experiment.
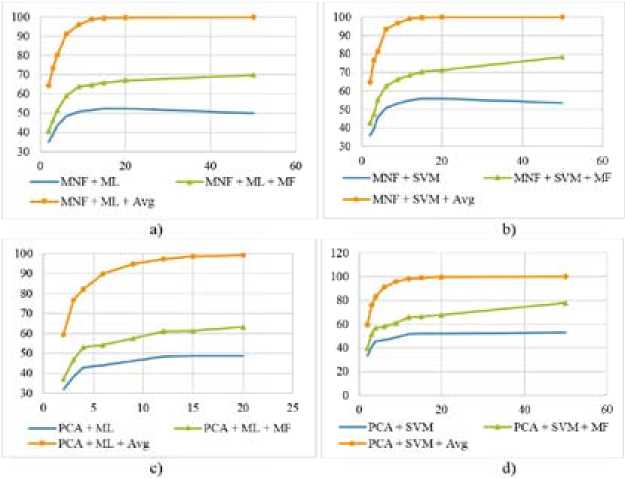
Fig. 3. Classification accuracy of image 1, based on different feature sets and classification methods, depending on number of features
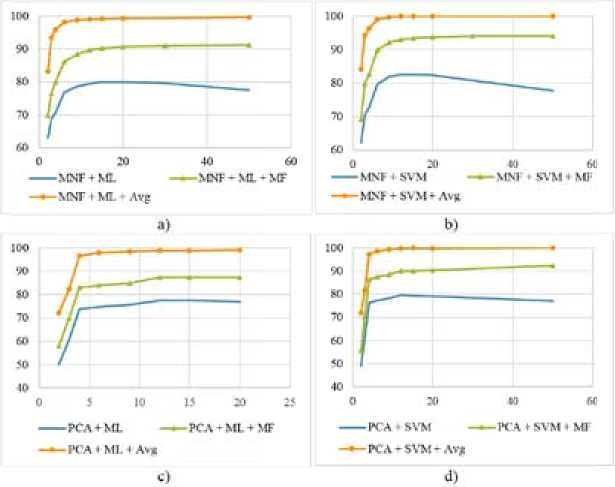
Fig. 4. Classification accuracy of image 2, based on different feature sets and classification methods, depending on number of features
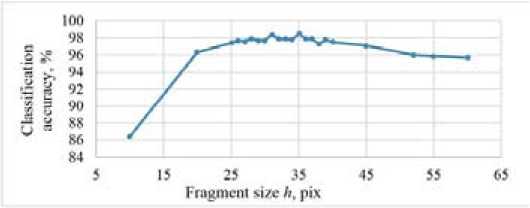
Fig. 5. Accuracy of spectral-textural classification based on pre-segmentation for image 2
Conclusion
Analysis of the results shows that spatial information makes it possible to achieve a significant improvement in the accuracy of classification (by 5–50%) in comparison to pixelwise spectral classification. For the test images used in this research the best results were achieved by classification based on geometric moments, the accuracy approached 100%.
This work was supported by RFBR (grant No. 14-07-00249-a).
Список литературы Comparison of spectral-spatial classification methods for hyperspectral images of high spatial resolution
- Дворкин Б.А., Дудкин С.А. Новейшие и перспективные спутники дистанционного зондирования Земли. Геоматика, 2013, 2, 16-21.
- Fauvel M., Benediktsson J.A., Chanussot J., Sveinsson J.R. Spectral and spatial classification of hyperspectral data using SVMs and morphological profiles. IEEE Trans. Geosci. Remote Sens., 2008, 46(11), 3804-3814.
- Kozoderov V.V., Kondranin T.V., Dmitriev E.V., Kamentsev V.P. Bayesian classifier applications of airborne hyperspectral imagery processing for forested areas. Advances in Space Research, 2015, 55(11), 2657-2667.
- Борзов С.М., Мельников П.В., Пестунов И.А., Потатуркин О.И., Федотов А.М. Комплексная обработка гиперспектральных изображений на основе спектральной и пространственной информации. Вычислительные технологии. 2016, 21(1), 25-39.
- Kumar B., Onkar D.O. Spectral-spatial classification of hyperspectral imagery based on moment invariants. IEEE Journal of selected topics in applied earth observations and remote sensing, 2015, 8(6), 2457-2463.
- Рылов С.А., Мельников П.В., Пестунов И.А. Спектрально-текстурная классификация гиперспектральных изображений высокого пространственного разрешения // Интерэкспо Гео-Сибирь. 2016; 4(1), 78-84.
- Пестунов И.А., Рылов С.А. Алгоритмы спектрально-текстурной сегментации спутниковых изображений высокого пространственного разрешения // Вестник Кемеровского государственного университета, 2012, 4/2 (52), 104-109.