Determinants of life expectancy in heterogeneous constituent entities of the Russian Federation
Автор: Dubrovskaya Julia V.
Журнал: Economic and Social Changes: Facts, Trends, Forecast @volnc-esc-en
Рубрика: Public finance
Статья в выпуске: 1 т.16, 2023 года.
Бесплатный доступ
Low life expectancy is one of the main factors hindering national economic development. Finding a solution to this problem is complicated by enormous differentiation of life expectancy among constituent entities of the Russian Federation. Therefore, assessing the factors increasing life expectancy in heterogeneous regions of the country and developing methodological tools for the application of territorial defragmentation in terms of policy to improve regional health systems are major tasks for public administration. The research aims to find a solution to these problems. On the basis of a detailed analysis of the relevant literature we form a statistical base of indicators influencing life expectancy in RF constituent entities for 10 years; with the help of “hierarchical clustering” we arrange RF constituent entities into eight groups according to the specifics of forming conditions for a long and healthy life; through econometric modeling we assess and identify the impact of individual indicators on life expectancy in different groups of RF constituent entities; on the basis of the modeling results we formulate areas of further research and priorities of the state regional policy in the field of health care. We use general scientific and special methods, including statistical, cartographic, typological, econometric, and cluster methods during the analysis of regional health systems. Statistical data processing and econometric modeling are carried out using statistical packages SPSS Statistics and Gretl. Scientific novelty of the study consists in the clustering of RF constituent entities according to the specifics of forming conditions for increasing life expectancy, which has fundamental importance for improving the effectiveness of territorial management and planning in the field of health care. Practical significance of the work is determined by the tasks of regional policy of Russia and its constituent entities in the sphere of increasing the life expectancy. The results of the study can be useful to the state regional authorities and local authorities for the formation of a set of measures to improve life expectancy.
Regional health care system, heterogeneous regions, life expectancy, strategic planning, clustering
Короткий адрес: https://sciup.org/147240251
IDR: 147240251 | DOI: 10.15838/esc.2023.1.85.8
Текст научной статьи Determinants of life expectancy in heterogeneous constituent entities of the Russian Federation
The research was carried out at the expense of the Russian Science Foundation, project no. 21-78-10134,
Life expectancy is the most important criterion of public administration efficiency and the guarantee of progressive development of territorial social systems. That is why one of the priority national goals of the Government of the Russian Federation is “to increase life expectancy to 78 years by 2024 and to 80 years by 2030”1. Objectively questioning the attainability of this target by 2024, we note that at the time of its planning, the world was not yet aware of the coming pandemic, which has actualized the issue of prioritizing budget investment in health care. For example, the effects of underfunding health infrastructure in a number of countries have led to excess mortality, lagging vaccine working out and, ultimately, slower economic growth and more uneven development.
Due to the coronavirus pandemic, public health spending in Russia rose to 4.6% of GDP in 20202. Despite this, according to 2018–2020 comparative analysis of health care financing models across 31 foreign countries by the Russian Accounts Chamber, “the Russian Federation has the lowest level of health care spending, including public spending, among all countries under review, with the highest share of off-budget spending among all the countries”3. A similar conclusion is that “in the absolute majority of countries with an economic development level close to the Russian one, states allocate more funds for health care than in Russia” was made by E.G. Potapchik (Potapchik, 2020). Insufficient investment in public health causes low values of the main indicator of the health care system quality – life expectancy. While people in developed countries live up to 80 years, the average life expectancy in Russia is less than 72 years.
At the same time, these aggregated macrodata do not fully reflect all the complexities of the functioning of the health care system in Russia, the most important of which is the enormous differentiation of the country’s constituent entities by life expectancy (from 66 to 81 years). For instance, according to Rosstat data for 2020, life expectancy at birth (for the entire population sample) is the highest in the regions of the North Caucasian Federal District (for example, the Republic of Ingushetia – 81.5 years; the Republic of Dagestan – 76.5 years; the Karachay-Cherkessia Republic – 75 years, etc. etc.), the lowest is in the regions of the Far Eastern Federal District (for example, Chukotka Autonomous Okrug –
66 years; the Amur Oblast – 67 years; the Jewish Autonomous Oblast – 67.5 years, etc.)4. At the same time, such high differences are observed in many groups of indicators of territorial development. Significant spatial heterogeneity not only complicates the implementation of a unified state policy in the field of health care, but also requires taking into account regional specifics in the plans, forecasts and programs of territorial development and strategizing. Proceeding from the above, it is objectively possible to conclude that estimation of factors promoting increasing life expectancy in heterogeneous regions of the country, and also development of methodical tools of application of territorial defragmentation in a part of the policy of improvement of regional public health systems are the major tasks of public administration. Our research is devoted to their solution.
Agreeing that “a characteristic feature of the modern development stage of economics is its mathematization, which manifests itself in the replacement of the studied economic process with an adequate economic model and the subsequent study of the properties of this model either by apathetic methods or on the basis of computational experiments” (Zang, 1999, p. 6), we note that the solution of the research tasks formulated by us earlier, which are of high applied importance for strategizing the development of regional health systems, objectively requires the formation and processing of a significant array of regional statistical data; the choice of the functional form of the model and the evaluation of coefficients using mathematical and econometric tools and programs; the appropriate interpretation of the results in order to form scientifically sound directions increase in life expectancy.
The article contains four main sections. In the first section, “Theoretical aspects of the problem and the main hypotheses”, we consider relevant scientific works. At the same time, in order to formulate research hypotheses, we carry out a detailed analysis of statistical indicators characterizing the quality of regional health systems. The second section of the work “Research methodology” contains methodological tools for determining regionally differentiated determinants of life expectancy based on the author’s clustering of Russia’s entities. We pay special attention to the justification of the choice and analysis of statistical indicators for clustering and modeling, as well as the formalization of the hypotheses put forward. The third section “Results” includes the methods obtained in the process of testing, the results and interpretation of the estimated coefficients. The fourth section “Discussions” substantiates the consistency of the author’s statements, assessments and results with the conclusions presented in the studies of other scientists, and also notes the objective shortcomings and limitations of the model. In conclusion, we highlight the most important results in brief, and formulate the scientific novelty and practical significance of the research.
Theoretical aspects of the problem and the main hypotheses
Good public health is the most important condition for the sustainable development of any territory. In this regard, there is no doubt that life expectancy is not only an indicator of the quality of life, but also an objective criterion for assessing the success of ongoing political, social and economic reforms. The high role of financial investments in the health care sector, which have a direct positive impact on labor productivity, has been proven by many scientists5 (Zubarevich, 2009; Demidova et al., 2021).
Despite the awareness of the critical importance of the health care system on the part of scientists and the state, “the concept of efficiency in health care continues to be multidimensional, while remaining a relative value” (Arkhipova, Dvoinikov, 2018, p. 26). The reason, in our opinion, is that the health care system is a multifunctional and multilevel system that is constantly influenced by internal and external factors. Its objective complexity generates the emergence of diverse research areas, among which the following groups can be conditionally distinguished: first, studying the activities of medical organizations in order to “independently assess the quality of medical care and the effectiveness of the activities of medical organizations providing medical care” (Mikhailova et al., 2016); second, the study of regional health systems, the purpose of which is “measuring the activity of the health care system in Russia in the regional context” (Kislitsyna, Chubarova, 2021); third, the study of health care as the leading branch of the social infrastructure of the national economy, which has an impact on the economic and social development of the country (Tatarkin et al., 2015).
We will focus on the previously updated problem of heterogeneity of indicators of regional development in Russia, including such an important indicator as life expectancy. The value of life expectancy, as well as the mortality rate, are referred in the scientific literature to social indicators of the effectiveness (efficiency) of the health care system
Table 1. Examples of social indicators of health care system performance
№ |
Authors |
Country, analysis period |
Statistical indicators |
1 |
A. Bhargava et al. (Bhargava et al., 2001) |
Developing countries, 1965–1990 |
Life expectancy at birth |
2 |
D.E. Bloom, P.N. Malaney (Bloom, Malaney, 1998) |
Russia, 1989–1994 |
|
3 |
R. Barro (Barro, 2013) |
100 world countries, 1960–1990 |
|
4 |
Y. Wang, C. Ni (Wang, Ni, 2015) |
China, 1987–2007 |
|
5 |
D. Barthold (Barthold, 2014) |
27 OECD countries, 1991–2007 |
Life expectancy for men/women |
6 |
S. Asiskovitch (Asiskovitch, 2010) |
27 OECD countries, 1990–2005 |
|
7 |
L.I. Vlasyuk, P.V. Stroev (Vlasyuk, Stroev, 2017) |
Russia, 2002–2015 |
|
8 |
E. Jaba, C.B. Balan, I.-B. Robu (Jaba et al., 2014) |
175 world countries, 1995–2010 |
|
9 |
K.А. Muldoon (Muldoon, 2011) |
136 world countries, 2001–2008 |
|
10 |
E. Brainerd, D.M. Cutler (Brainerd, Cutler, 2005) |
24 countries, 1989–2002 |
Mortality rates |
11 |
J.C. Anyanwu, A.E.O. Erhijakpor (Anyanwu, Erhijakpor, 2009) |
47 countries in Africa, 1999–2004 |
|
Source: own compilation based on the analysis of literary sources. |
(Orlov, Sokolova, 2010; Tuaeva, Sugarova, 2013), reflecting “the degree of achievement of socially significant goals related to the provision of medical services” 6. As a rule, social indicators are the target indicators in various programs and strategies of country and regional development, which explains their choice as endogenous variables in modeling (Kolosnitsyna et al., 2019). Table 1 gives an overview of the use of social indicators of the effectiveness of the health care system in the scientific literature.
The analysis of the research papers revealed two main groups of factors for increasing life expectancy: economic indicators of the effectiveness of the health care system and medical indicators of its quality (Kalashnikov, 2011; Panasyuk, Dasaeva, 2014; Tatarkin et al., 2015; Krivenko et al., 2019; Ulumbekova et al., 2019). Let us focus in more detail on the content and structure of these indicators.
Economic indicators of the effectiveness of territorial health care systems traditionally characterize the dependence of results on the resources expended (Jaba et al., 2014) and include in general terms such indicators as the share of health care expenditures in GDP (GRP), investments in health care, private health care spending and others.
For instance, N.V. Akindinova, A.V. Cher-nyavskii, A.A. Chepel (Akindinova et al., 2018) emphasize the importance of defining health care costs as part of “productive” budget expenditures, since they affect labor productivity. In this context, it is important to note that the level of health that determines a person’s ability to fully use their human capital can serve as a limiter of economic growth and bring economic benefits not only at the level of individual citizens, but also at the state level. Stolbov notes that “health care, along with its main task of improving people’s health, contributes to the growth of production efficiency” (Stolbov, 2016). The paper (Kalashnikov, 2011) proposes a method for calculating the scale of lost profits in the production of GRP as a result of premature mortality. The research of I.A. Erzyleva (Erzyleva, 2020) is devoted to “the analysis of the main trends and problems shaping the current health status of the Russian population, and the assessment of the impact of this factor on the socio-economic development of the state”. The author notes that Russia has a larger number of human resources, but they are unable to show and realize their labor and intellectual potential due to low life expectancy and low development level of the health care sector. M.A. Kaneva assesses the impact of the level of “health on regional growth in the framework of an approach that takes into account the costs of health care to maintain the human capital quality” (Kaneva, 2019). The results obtained indicate that “an increase in government spending on health care as a share of GRP by 1% is associated with an increase in the growth rate of GRP per capita by 1.34%”. At the same time, the results are of particular interest, according to which private spending on health care, reflecting the consumption of paid medical services, has a negative impact on economic growth.
Thus, most of the studies emphasize the need to increase public spending on health care, which will improve the quality of life and increase life expectancy. In turn, it will lead to an increase in the number of workers and labor productivity growth, positively affecting the total output. Table 2 gives an overview of the use of economic indicators of the effectiveness of health care systems in the scientific literature.
Table 2. Examples of economic indicators of the effectiveness of the health care system
No. |
Authors |
Country, analysis period |
Statistical indicators |
1 |
O.A. Demidova, E.V. Kayasheva, A.V. Dem’yanenko (Demidova et al., 2021) |
Russia, 2005–2017 |
Share of expenditures on health care, physical education and sports in GRP |
2 |
L.I. Vlasyuk, P.V. Stroev (Vlasyuk, Stroev, 2017) |
Russia, 2002–2015 |
Expenditures of consolidated budgets on health care |
3 |
G.E. Ulumbekova, A.B. Ginoyan, E.A. Chaban (Ulumbekova et al., 2016) |
Russia, 2011–2014 |
Per capita government spending on health care |
4 |
G.E. Ulumbekova et al. (Ulumbekova et al., 2019) |
Russia, 2011–2016 |
|
5 |
E. Brainerd, D.M. Cutler (Brainerd, Cutler, 2005) |
24 countries, 1989–2002 |
|
6 |
E.M. Andreev, V.M. Shkol’nikov (Andreev, Shkol’nikov, 2018) |
Russia, 2010 |
Financing under the program of state guarantees for provision of free medical care to Russian citizens in the context of Russia’s entities |
7 |
M.A. Kaneva (Kaneva, 2019) |
Russia, 2005–2013 |
|
8 |
N.S. Gorchakova (Gorchakova, 2020) |
Russia, 1995–2017 |
Health care expenditure share in GDP |
9 |
S. Gupta, M. Verhoeven, E.R. Tiongson (Gupta et al., 2003) |
76 countries, 1990–1999 |
|
10 |
A.V. Komarova, E.V. Kritsyna (Komarova, Kritsyna, 2012) |
Russia, 1974–2009 |
Investments in health care system |
Source: own compilation based on the analysis of literary sources. |
Medical quality indicators of territorial health care systems are used to assess the availability of medical care, expressed in the provision of doctors/ secondary medical personnel, provision of hospital beds, etc. We believe that these indicators have shaped the current levels of life expectancy in various countries of the world for centuries (Erakhtina, 2019).
The importance of studying medical indicators of the quality of health care systems in relation to the regions of the national economy is gaining additional relevance in connection with the health care reform in Russia since 2010, which “consisted in optimizing costs by closing inefficient hospitals and expanding the use of high-tech medical institutions” (Grishina, Karpova, 2020, p. 172). According to the calculations of specialists of the Center for Economic and Political Reforms, in the period from 2000 to 2015, the number of hospitals in Russia decreased by half – from 10.7 to 5.4 thousand. The number of polyclinics for the same period decreased by 12.7% — to 18.6 thousand institutions. Experts concluded that if such rates of hospital closure continue (approximately 353 annually) by 2021–2022, the number of medical institutions in the country will reach three thousand, as the level of the Russian Empire in 19137. Figure 1 shows the dynamics of the reduction in the number of medical institutions and changes in the mortality/birth rate in 2010– 2020 in Russia.
As a result of optimization, there was a natural reduction in expenditures in the health care sector. T. Golikova characterized the reform results as follows: “Optimization was unsuccessful in many regions”8.
Figure 1. Dynamics of reduction in the number of medical institutions and changes in the mortality/birth rate from 2010 to 2020
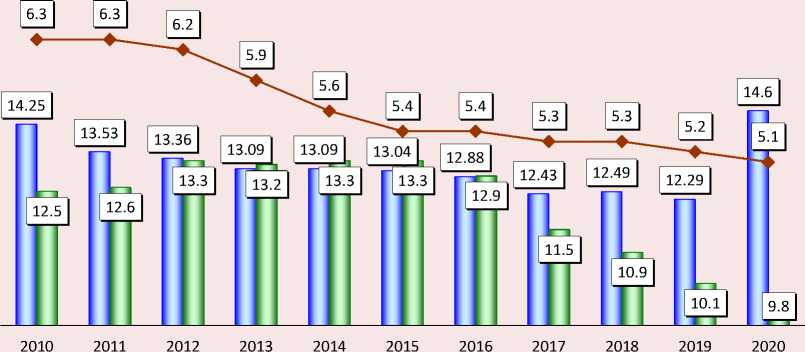
■ Fertility rate, % i i Mortality rate, %
♦ Number of hospitals, thousand
Source: Regions of Russia. Socio-Economic Indicators. 2021: Statistical Collection. Rosstat. Moscow, 2021.
The COVID-19 pandemic in 2020 showed that the country had a shortage of hospital beds for treating patients with increased morbidity. For example, according to an analysis by E.V. Reprintseva, between 2012 and 2018, the availability of inpatient beds decreased by 15%. “While in 2005 there were about 9,500 hospitals with a total number of hospital beds of more than 1.5 million units, by 2018 these figures had dropped to 5,300 hospitals and 1.2 million beds, respectively” (Reprintseva, 2020, p. 282).
Figure 2 presents the dynamics of the indicator “Capacity (number of visits per shift) of outpatient and polyclinic organizations” for the period 2004– 2020. The dot marks the value of the increase in the average capacity of one health care facility during the study period.
According to Figure 2, all federal districts have an increase in the average capacity of one medical institution during the researched period, while the number of medical organizations was decreasing. It may indicate both the construction of new spacious medical institutions with large areas, and their simple enlargement, when the load per doctor increases in the form of an increase in the number of visits. The highest growth rates are shown by the regions of the North Caucasian Federal District (Republic of Dagestan, Republic of Ingushetia and Stavropol Krai – 249, 294 and 143%, respectively).
Based on the data analyzed, demonstrating an increase in mortality with a decrease in the number of hospital beds and the number of doctors, we can verify the correctness of the conclusions of E.V. Reprintseva that “modernization of health care has led to a reduction in the number of inpatient hospital facilities with simultaneous enlargement of the hospital network. This has led to a decrease in the availability of inpatient medical care for the country’s population, especially in rural areas, and the situation may worsen in the future” (Reprintseva, 2020, p. 283). This situation requires evidence-based adjustments in the system of management decisions made by the competent authorities.
In light of the above, the choice of statistical indicators for quantitative measurement of the effectiveness of the health care system is a debatable point. Table 3 presents the results of the systematization of the relevant empirical works studied by us, in which the authors used statistical data reflecting the accessibility of medical care.
Figure 2. Dynamics of capacity of outpatient and polyclinic organizations from 2004 to 2020
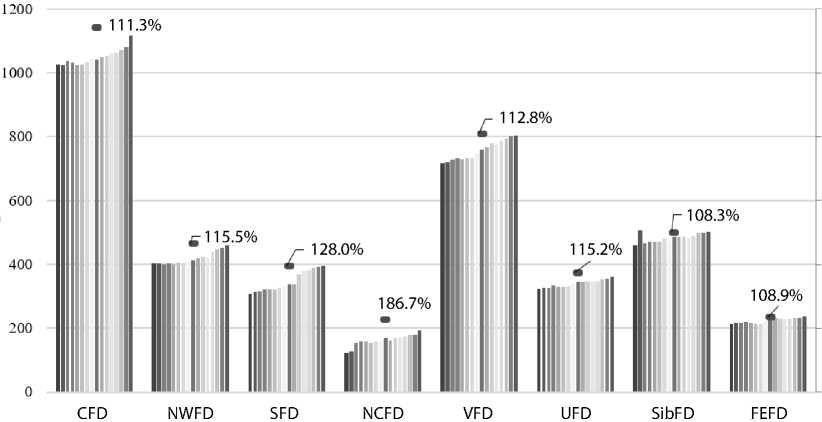
Source: Regions of Russia. Socio-Economic Indicators. 2021: Statistical Collection. Rosstat. Moscow, 2021. Pp. 366–367.
Table 3. Examples of medical indicators of health care quality
No. |
Authors |
Country, analysis period |
Statistical indicators |
1 |
L.A. Tuaeva, I.V. Sugarova (Tuaeva, Sugarova, 2013) |
Russia, 2000–2012 |
|
2 |
A.Sh. Akhmeduev (Akhmeduev, 2019) |
12 world countries, 2013–2014 |
|
3 |
N.V. Krivenko, V.M. Ivanov, L.A. Kriventsova (Krivenko et al., 2019) |
Sverdlovsk Oblast, 2013–2017 |
Certain types of medical care (outpatient care, day patient facility, round-the-clock inpatient facility) |
4 |
V.I. Starodubov et al. (Starodubov et al., 2013) |
Russia, 2010 |
|
5 |
J.C. Anyanwu, A.E.O. Erhijakpor (Anyanwu, Erhijakpor, 2009) |
47 countries of Africa, 1999–2004 |
Number of doctors/nurses |
6 |
W.J.A. van Den Heuvel, M. Olaroiu (van Den Heuvel, Olaroiu, 2017) |
31 countries of Europe, 2013 |
|
7 |
D.L. Skipin et al. (Skipin, 2022) |
Russia, 1995–2017 |
|
8 |
A.K. Zhukova et al. (Zhukova et al., 2016) |
Russia, 2014 |
|
Source: own compilation based on the analysis of literary sources. |
In addition to medical and economic, researchers identify the following factors that significantly affect the social performance of health care systems: education (Rosenzweig, Schultz, 1982; Desai, Alva, 1998; Thomas et al., 1990; Currie, Moretti, 2003; Anyanwu, Erhijakpor, 2009), prevalence of HIV infection (Gupta et al, 2003), urbanization rates and population demographics (Roberts, 2003; Anyanwu, Erhijakpor, 2009; Zhukova et al., 2016; Ulumbekova et al., 2016), government spending on social protection (Heuvel, Olaroiu, 2017), unhealthy lifestyle indicators (alcohol consumption, smoking, overeating) (Brainerd, Cutler, 2005; Heuvel, Olaroiu, 2017; Zhukova et al, 2016; Ulumbekova et al., 2016; Kossova et al., 2017; Kolosnitsyna et al., 2019, Ulumbekova et al., 2019), level of government corruption (Muldoon et al., 2011), population cash income and gross product per capita (Swift, 2011; Zhukova et al., 2016; Ulumbekova et al, 2016; Ulumbekova et al., 2019; Skipin et al., 2022), stressors (suicides, abortions) (Chekmeneva and Balina, 2019; Balina et al., 2021), poverty rates (Ulumbekova et al., 2016), environmental factors (Muldoon et al., 2011; Zhukova et al., 2016; Balina et al., 2021).
Based on the spatial features of the national economy, characterized by a high asymmetry of the development of Russia’s entities, in the analysis of the scientific literature, we identified a number of methodological aspects that objectively require improvement and that have been overlooked by researchers.
First, when assessing health care quality, scientists often use integral indices, on the basis of which ratings of the effectiveness of health care systems are further compiled (Krivenko et al., 2019; Kislitsyna, Chubarova, 2021; Ulumbekova, Ginosyan, 2021). At the same time, given the many objective limitations of applying integral indices (Masset, 2011) and the fact that their quality and accuracy crucially depend both on the completeness of available data and on methodological approaches to their compilation (Santeramo, 2015), we agree that “ideal integral indices are the exception rather than the rule” (Pavlova et al., 2018, p. 234).
Second, when building models on panel data for the purpose of health care system assessment, scientists usually include in the sample the entire population of territories for which statistical data were collected (Muldoon et al., 2011; Kossova et al., 2017; Ulumbekova et al., 2016; Ulumbekova et al., 2019). At the same time, preliminary clustering of heterogeneous territories is an important stage in studying health care systems, as it allows obtaining, as a result, more objective estimates of modeling for separate groups of regions, which is critical for the developed directions on improving the organization of health care. This thesis is confirmed in the study of E. Jaba, C.B. Balan, I.-B. Robu (Japa et al., 2014), in which the authors conducted a preliminary grouping of 175 countries in terms of “income level” and “geographic location”. The results of the models built by the researchers on panel data for 15 years showed different estimates for different groups of countries, which provides a unique opportunity to use the preliminary territorial defragmentation in developing directions for improving health care systems. Also we should note the studies in which the defragmentation of policies to improve territorial health systems is not based on clustering objects from the general population (countries/territories), but by taking into account the features of the analyzed indicators, for example the decomposition of differences in life expectancy by gender (Asiskovitch, 2010; Barthold et al, 2014; Andreev, Shkolnikov, 2018; Zhukova et al., 2016), decomposition of differences in mortality between the poor and the rich (Gupta et al., 2003).
Among the Russian studies in this context, we would like to single out, first, the work (Skipin et al., 2022), which presents the results of clustering Russia’s entities by the growth rate of life expectancy, revealing regional specificity. Second, the publication (Zaitseva et al., 2019), where a preliminary clustering of RF entities was performed in the study of regional health care systems, which allowed concluding that “socio-economic determinants have regional differentiation by the level of their potentiating or restraining effect on life expectancy” (Zaitseva et al., 2019, p. 25) seems important. However, the four groups of Russia’s entities obtained in the work are not homogeneous in structure, which significantly reduces, in our opinion, the effectiveness of the directions of improvement of regional health care systems developed on this basis. Third, it is the work (Kolosnitsyna et al., 2019), in which the analyzed countries are grouped into three clusters by the value of life expectancy and the correlation matrices of the variables characterizing population health are constructed, seems interesting.
Thus, summarizing the critical review of the scientific works, we note that an objective necessity in studying regional health care systems is a preliminary scientifically substantiated clustering of territories, which makes it possible to form unique areas of health care systems development based on the identification of regionally differentiated determinants of life expectancy, which is the purpose of our research .
The goal setting is accompanied by the formulation of scientific hypotheses. In this regard, we should note that most of the analyzed works revealed a positive statistically significant dependence of social indicators of healthcare systems performance (in particular, life expectancy) on medical and economic indicators, characterized by accessibility of medical care and health care expenditures. Thus, we can assume with a high degree of probability that the number of years of life expectancy is a consequence of the level of financing of medical institutions and the health care industry in the region as a whole.
At the same time, considering, on the one hand, that “life expectancy is a multifactorial value, depending, among other things, on natural, climatic conditions, environmental conditions...” (Krivenko et al., 2019, p. 2227), and on the other hand, the differences in the results and effects obtained in the construction of the same models for assessing the determinants of health care systems for different countries and regions (Jaba et al., 2014), we believe that consideration of territorial features when testing the hypothesis about the differential impact of indicators of the general state of the regional economy on indicators of social performance of the health care system of Russia’s entities is critical.
These conclusions, as well as the classification of the quality indicators of health care systems, constructed by us, allowed formulating a number of hypotheses, which were tested in relation to the regions of the national economy.
Hypothesis 1. The influence of economic indicators of the efficiency of the regional health care system on life expectancy is positive.
Hypothesis 2. The influence of medical indicators of the quality of the regional health care system on life expectancy is positive.
Hypothesis 3. The nature of the influence of indicators of the general state of the region’s economic development on life expectancy is conditioned by territorial features.
Research methodology
To achieve this purpose, we propose to use our own methodological toolkit for assessing the determinants of life expectancy in heterogeneous Russia’s entities, which includes five stages.
At the first stage, the statistical data base for Russia’s entities for the available period is collected in order to conduct further cluster analysis on the basis of the formation of conditions for a long and healthy life in the regions. We formed the clustering criteria taking into account the WHO study9, according to which the key factors promoting life expectancy include citizens’ lifestyle, their health status, level of organization of medical care, climate, environmental features and genetics. In addition, we followed the examples of evidencebased methodologies for grouping territories according to the specifics of life expectancy formation, which were tested by scientists and resulted in compact clusters that lend themselves to economic interpretation (Kolosnitsyna et al., 2019; Chekmenyeva, Balina, 2019; Balina et al., 2021).
The final database for clustering indicators includes four blocks: social, economic, psychological and environmental. Taking into account the importance of ensuring the adequacy and transparency of the interpretation of the cluster analysis results by minimizing the number of indicators for clustering10, as well as taking into account the availability of existing information by Russia’s regions, by analogy with the work of T.A. Balina and co-authors (Balina et al., 2021), we selected six indicators in the context of the formed blocks: life expectancy at birth; number of students; infant mortality (social block); real monetary incomes (economic block); suicide rate (psychological block); availability of clean drinking water (natural and ecological block).
At the second stage , the collected data is processed and normalized. For a number of indicators (in our case, mortality and suicide rates) the inverse values are considered. For indicators characterizing monetary relations, we make an adjustment to the annual consumer price index.
At the third stage , clustering of Russia’s entities is carried out on the basis of the formation of conditions in the regions for a long and healthy public life in order to ensure the uniformity of the distribution of the studied regional data. Regional grouping is performed based on the methodology of cluster analysis in the software package SPSS Statistics (Statistical Package for the Social Sciences). Since the total number of clusters is not known in advance, a hierarchical algorithm is used as a clustering method. The metric is the Euclidean distance, in order to take into account equally the differences of RF entities according to the selected statistical characteristics. The separation of clusters is based on the Ward method, which involves the use of variance analysis to estimate the distances between clusters. This minimizes the sum of squares for any two (hypothetical) clusters that can be formed at each step.
At the fourth stage , we stimulate the differentiated influence of the selected factors of life expectancy (determinants) in the formed regional groups. The simulation was performed in Gretl, a cross-platform software package for econometric analysis written in C language.
We generate panel data for 10 years (from 2010 to 2019) for each group of regions selected for econometric analysis. The missing data occurring in one year is restored by prolongation. Regions with data gaps for more than two years are excluded from the analysis. Then we build regression models. We used the indicator “life expectancy at birth” as an endogenous variable. We divided exogenous variables into two groups. The first category includes variables of interest – economic indicators of the effectiveness of the health care system and medical indicators of its quality. With the help of variables of interest, we test hypotheses 1 and 2, verifying assumptions about the significant positive impact of government measures to increase public spending on health care and increase the availability of medical care on life expectancy.
The second group included indicators that also have, according to previously analyzed research studies, a significant impact on life expectancy, but unlike the variables of the first group, they mostly depend on territorial characteristics rather than state regulation. These variables are assigned to the control ones necessary for leveling the bias of coefficient estimates for the model variable. In addition, our study uses control variables to test hypothesis 3, which verifies the assumption that the nature of the influence of indicators of the general development state of the region’s economy on life expectancy is due to territorial features. Let us dwell in more detail on the justification of the choice of control variables.
As we shown it in the previous section, the list of exogenous variables used by scientists in modeling life expectancy, in addition to economic indicators of the effectiveness of the health care system and medical indicators of its quality, is quite wide. When choosing a set of variables, we were guided primarily by the results of relevant studies that revealed the most important factors of life expectancy changes: education, income, non-traditional risk factors for morbidity11, and environmental factors. They quite fully reflect the quality life and standard of living from the perspective of three basic aspects: socio-economic (education and income of the population12), socio-psychological (stress factors expressed through the suicide rate13) and environmental (availability of clean air and drinking water) (Kolosnitsyna et al., 2019; Balina et al., 2021).
In addition to theoretical prerequisites, when forming the database, we took into account the actual presence of similar indicators in the official regional Rosstat statistics for the analyzed period of time. Table 4 provides information on the normalization of variables for modeling, the method of their calculation and units of measurement.
We conducted statistical tests to determine the best model specification; at the same time we evaluated three types of models: models with fixed effects, with random effects, and the pooled least square method (LSM). The best model was chosen based on the Akaike and Schwartz information criteria, as well as the results of statistical tests: the F-test, the Breusch – Pagan test, and the Hausman test. Based on the F-test results, we chose between LSM and models with fixed effects; using the Breusch – Pagan test, between LSM and models with random effects. The Hausman test allows making a correct choice between models with fixed and random effects.
At the fifth stage , based on the obtained econometric estimates, we interpret the results, formulate difficulties in the functioning of the health care system, and develop priority management decisions in the context of groups of regions.
Table 4. Indicators for simulating and evaluating the conditions for the formation of long and healthy public life in RF entities
Variable name |
Variable description |
Unit of measure |
Calculation method |
Endogenous variables |
|||
Life_exp |
Life expectancy at birth |
years |
Number of years of life to come |
Exogenous variables |
|||
Variables of interest |
|||
Hea |
Health care cost |
thousand rubles |
Expenditures of the consolidated budgets of Russia’s entities on health care, thousand rubles per person |
Doc |
Number of doctors |
people |
Number of doctors with higher medical education per 10,000 of the population employed in medical and preventive treatment organizations |
Hos_beds |
Number of hospital beds |
hospital bed |
Number of hospital beds per 10,000 people in the population. Beds that are equipped with the necessary inventory and ready to receive patients are to be counted |
Control variables |
|||
Stu |
Number of students in higher education programs |
people |
Number of students enrolled in bachelor’s, specialist’s and master’s degree programs per 10,000 people (at the beginning of the academic year) |
Inc |
Real cash income |
thousand rubles |
Cash annual income (average per capita) in the Russian Federation, adjusted for the consumer price index |
Sui |
Number of suicide |
people |
Number of registered deaths by major classes and individual causes of death (operational data) (suicide) per 100,000 people |
Wat |
Availability of clean drinking water |
people |
Availability of drinking water that meets safety requirements per 1,000 people |
Neu_Pol |
Air cleaning |
% |
Share of air pollutants captured and neutralized in the total amount of waste pollutants from stationary sources |
Source: Regions of Russia. Socio-Economic Indicators 2021: Stat. Coll. Rosstat. Moscow, 2021. |
Results
According to the described research methodology, in order to form a system of management decisions in the work, we carried out clustering of regions according to the specifics of the formation of conditions for increasing life expectancy. We conducted lustering according to the 2018 data, which was due to the need to minimize the problem of an unbalanced sample, which arose as a result of the fact that the indicators used in the aggregate were available only for the specified period. Figure 3 shows a dendrogram for 85 studied entities of Russia.
By the expert method, according to the unification schedule, we chose a distance equal to 8.5 as the threshold distance14. Each cluster was assigned conditional names reflecting their quantitative characteristics in terms of the specifics of the formation of conditions for a long and healthy public life ( Tab. 5 ).
In Table 5, we highlight the extremely high indicators in comparison with the national average in red, and on the contrary, in green is the values of
Figure 3. Region classification tree
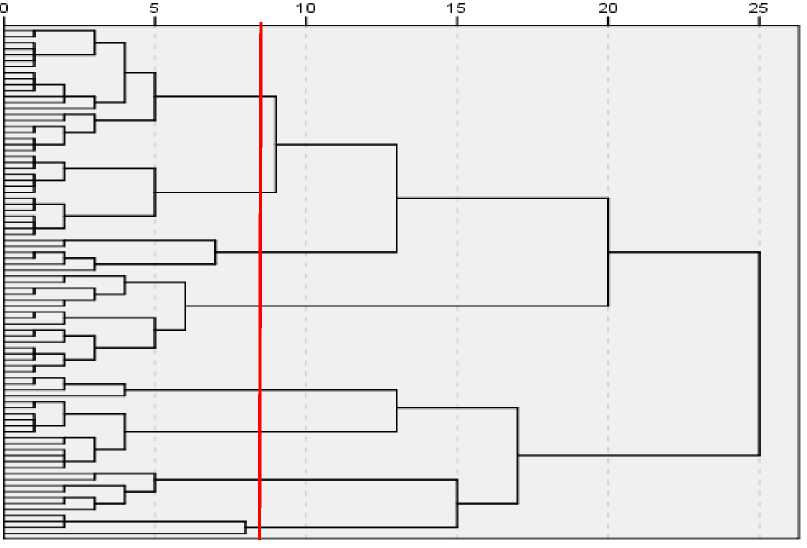
Source: own compilation.
indicators for which the group of regions is leading compared to the average values for the entire sample of Russia’s entities. We should note that the first cluster group is the only one of the eight that has reached the values planned by the national project “Health care” according to the target indicator “infant mortality rate”15. And the seventh cluster group is the only one who has reached the target for life expectancy.
Figure 4 shows a cartographic visualization of cluster groups based on the formation of conditions in the regions to increase life expectancy.
Let us take a closer look at the obtained characteristics of the regions. The first group of regions (“The rich and happy”) is characterized by high values for all indicators selected for clustering. Moscow and Saint Petersburg stand out especially in this group. The group of Russia’s entities, united under the name “Overcoming psycho-emotional barriers”, is characterized by high suicide rates, which, together with low relative to the national average indicators of real incomes and life expectancy in general, creates an image of regions where there are a number of problems in the formation of conditions for a long and healthy public life.
The entities included in the group “Overcoming natural and environmental barriers” are characterized by a low indicator of the availability of clean drinking water compared to the national average. This, along with the low values of life expectancy, necessitates an additional analysis of the level of anthropogenic load in these territories.
Table 5. Quantitative characteristics of the obtained clusters
Е Е Е Е Е 1 |
I |
Д |
g |
Е Ё > О |
д |
о Ё > О |
д |
Е Е > Ё Ё р |
Е "го > го Ё р |
д |
р |
д |
ь- |
7 |
р |
д |
S |
^5 CD с ГО ° 5 О) If 1 го ■25 "55 Е -^ о го !| Ё ® го 55 2 "Ё ^ | 1 § го “g го х S- Ф С/D с: Ё Ё ° 7 ” “ X Я ° ш| S я § S g |
||
ей |
Д |
д |
д |
г? |
д |
д |
СО |
|||||||||||||
О |
Д |
д |
д |
д |
д |
g |
||||||||||||||
д |
д |
со |
д |
д |
||||||||||||||||
Е |
о Е о 1 Ё о |
"го C/D 1 § |
S >. _Е _с= ГО^ го _го "го § о с о ID § 2 Е ^ ^ го 1 Е ей го s 5 Е го о Е ^ ^ го^ "^ "го Е" 5 го о _го 5 ^ го го 1 « о ^ |
Е S 1 S > _го Л Го го CZ) с: го I! ^ ^ Л у « 2 О 5 |
Е LLJ ГО — ^ ГО ГО > о S § О) “ 5 "го го ^ § $ "го ^ ^ го Е Ё ^ о го > ° 2 "го ^ ГО Го ^ ОО о "го Е о Е =s — ° о | 1 о 5 S |
го ^ го ^ го Н S го "го го 1 -Е "го ^ m .^ 1 ^ о S |
"го ОО го S о "Ё го Е го ^ го S го "го о |
S S го .Ё S |
i |
■ 5 |
||||||||||
о |
^ |
CSJ |
со |
^г |
LO |
со |
со |
Figure 4. Cartographic visualization of cluster groups according to the specifics of the formation of conditions for increasing life expectancy
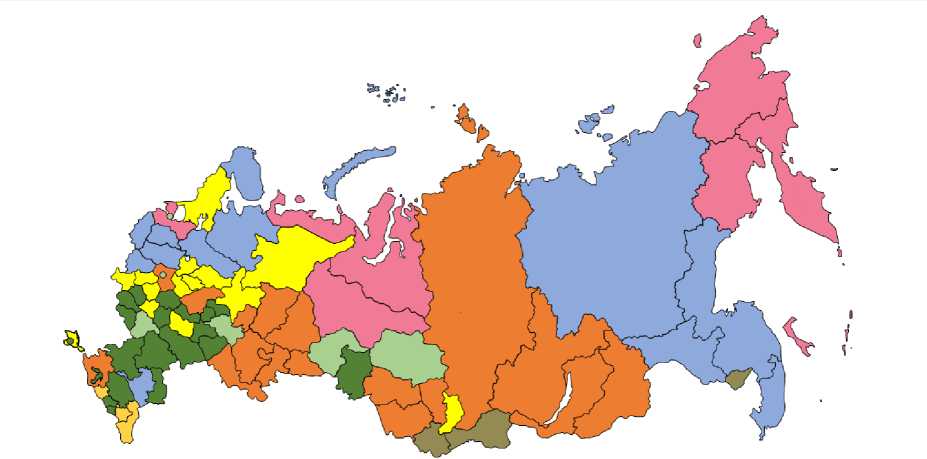
Cluster 1 Cluster 2 Cluster 3 Cluster 4
Cluster 5 Cluster 6 Cluster 7 Cluster 8
Source: own compilation.
Next, we will consider the groups “Optimistic regions of problematic socio-economic development” and “Regions of problematic socioeconomic development” that are quite similar in terms of the analyzed indicators: low incomes, average life expectancy, average availability of natural (water) and human (students) resources. In the future, when modeling, these two groups will be combined.
The group of regions “Resource, low-population” definitely has pronounced features in terms of a high level of availability of natural resources. In addition, except the Leningrad Oblast, the population density in these regions is significantly lower than the national average. The group of regions “The poor but happy” includes four republics of the North Caucasus, united on the basis of high life expectancy, low suicide rates and low incomes.
The last group of regions (“Socially stressed”) included three traditionally depressed entities of Russia with low life expectancy, high suicide rates and low incomes.
Clustering of Russia’s entities according to the specifics of the formation of conditions for increasing life expectancy makes it possible to ensure the uniformity of the distribution of the studied regional data. According to the research methodology described in the paper, we assessed the impact of indicators characterizing regional characteristics on life expectancy in relation to three groups: “Overcoming psycho-emotional barriers”, “Overcoming natural and environmental barriers” and the combined group “Optimistic regions of problematic socio-economic development” and “Regions of problematic socioeconomic development”. The remaining groups identified during clustering combine 21 outlier regions and require additional research due to the specifics of their functioning.
Tables 6–8 show the best models according to the results of statistical tests for each of the three analyzed cluster groups, respectively. In all three models, fixed-effect models were selected based on the results of the F-test, the Breush – Pagan test and the Hausman test.
Let us interpret the results obtained. Table 6 presents a detailed assessment of the selected model (models with fixed effects) for the group of regions “Overcoming psycho-emotional barriers”.
Commenting on the coefficients obtained with control regressors, we note that health care costs (feedback), per capita income parameters (direct link), the number of students (feedback) and suicide rate (feedback) are significant at the 1% level. Thus, in the group of regions “Overcoming psycho-emotional barriers”, measures aimed at reducing stress factors require special attention from the responsible authorities. First of all, this is work in the field of suicide prevention. An interesting result of the model, which requires additional scientific research, is the negative sustained and highly significant impact of health care costs on life expectancy.
Next, we will consider the results of econometric modeling estimates for the combined group “Optimistic regions of problematic socio-economic development” and “Regions of problematic socioeconomic development” ( Tab. 7 ).
Commenting on the coefficients obtained with the control regressors, we note that the parameters of average per capita income (direct relationship),
Table 6. Detailed assessment of the selected model (models with fixed effects) for the group of regions “Overcoming psycho-emotional barriers”
Variable name |
Coefficient |
St. mistake |
t -statistics |
p -value |
const |
70.182 |
1.499 |
46.810 |
0.000*** |
Variable of interest |
||||
Hos_beds |
0.001 |
0.010 |
0.138 |
0.890 |
Doc |
0.007 |
0.019 |
0.377 |
0.707 |
Hea |
-0.069 |
0.018 |
-3.752 |
0.000*** |
Control variables |
||||
Stu |
-0.007 |
0.001 |
-5.664 |
0.000*** |
Sui |
-0,053 |
0,009 |
-5,736 |
0,000*** |
Inc |
0.000 |
0.000 |
5.632 |
0.000*** |
Wat |
0.002 |
0.001 |
1.591 |
0.114 |
Neu_Pol |
-0.008 |
0.005 |
-1.607 |
0.110 |
Model description |
||||
Number of observations |
170 |
|||
Determination coefficient |
0.9645 |
|||
Adjusted determination coefficient |
0.9290 |
|||
Model evaluation criteria |
||||
F-test |
F = 49.917, p-value = 0.000 |
|||
Breusch – Pagan test |
x 2= 288.295, p-value = 0.000 |
|||
Hausman test |
x 2= 25.764, p-value = 0.002 |
|||
Akaike criteria |
177.749 |
|||
Schwartz criteria |
256.145 |
|||
Note: the symbols *, **, *** denote scores that are significant at the 10, 5 and 1% level, respectively. Source: own compilation. |
Table 7. Detailed evaluation of the selected model (model with fixed effects) for the combined group of regions
Next, we will consider the results of econometric modeling estimates for the group of regions “Overcoming natural-environmental barriers” ( Tab. 8 ).
Commenting on the coefficients obtained with control regressors, we note that at the 1% level the parameters of average per capita income are significant (direct relationship); at the 5% level both parameters characterizing the state of the environment (availability of drinking water and
16 In order to compile a complete panel, the regions with gaps in the data (the Republic of Crimea and Sevastopol) were removed.
Table 8. Detailed evaluation of the selected model (model with fixed effects) for the group of regions “Overcoming natural-environmental barriers”
The estimates obtained in the course of simulation helped to identify the specifics of the impact of individual indicators on life expectancy in different groups of Russia’s entities and to outline the directions for further research.
Discussion
Let us briefly discuss the results in the context of the research hypotheses formulated in the paper.
First, hypothesis 1, which consists in the fact that the influence of economic indicators of regional health care system efficiency on life expectancy is positive, has not found its empirical confirmation. For instance, according to the obtained model estimates, health care expenditures have a statistically significant inverse effect on life expectancy in the groups “Overcoming psycho-emotional barriers”, “Optimistic regions of problematic socio-economic development” and “Regions of problematic socio-economic development”. Agreeing with the fact that “the relationship between health care expenditures and health indicators (in particular, life expectancy) is quite complex and studies often give ambiguous results” (Kolosnitsina et al., 2019, p. 128), we note that contrary to the established scientific opinion about the positive relationship of these parameters in a number of works on Russian data were obtained inverse or insignificant dependence.
For example, the significant inverse dependence obtained in (Andreev, Shkolnikov, 2018) is explained by the fact that higher health care costs are objectively characteristic of territories with low health indicators and high mortality rates. In the work (Brainerd, Cutler, 2005), the lack of a significant relationship between increased public spending on health care and reduced mortality prompts scientists to turn to the study of non-traditional morbidity risk factors (e.g., alcohol, smoking, and others). In addition, we should also note that the significant inverse relationship obtained in our study between public spending on health care and life expectancy may indirectly indicate the inefficient use of budgetary funds for health care, in particular the suboptimal structure and directions of spending (Akindinova et al., 2018). Thus, verification of this assumption, which goes against the works determining the expenditures on health care in improving the social performance of health care systems (Kalashnikov, 2011; Panasyuk, Dasaeva, 2014; Tatarkin et al., 2015; Ulumbekova et al., 2019; Chubarova, 2020), requires more in-depth research.
Second, hypothesis 2, which consists in the fact that the influence of medical indicators of the quality of the regional health care system on life expectancy is positive, was partially confirmed. As in the works (Muldoon et al., 2011; Heuvel, Olaroiu, 2017; Zhukova et al., 2016), we obtained statistically significant correlations of the positive influence of the number of doctors on life expectancy by the largest by composition groups “Optimistic regions of problem socio-economic development” and “Regions of problem socio-economic development”. At the same time, a statistically significant inverse relationship was obtained for the indicator “number of hospital beds” for the same groups of regions. We found similar results in (Heuvel, Olaroiu, 2017), the authors of which explain the obtained negative relationship by the priority of preventive measures and social spending of the state instead of increasing the number of hospital beds, as well as in (Skipin et al., 2022), where this result is explained by the priority of the quality of health services, rather than the number of hospital beds.
Third, hypothesis 3, that the nature of the influence of indicators of the general state of regional economic development on life expectancy is determined by territorial features, has been fully confirmed in the course of empirical testing. For instance, except for the parameter of average per capita income, which turned out to be statistically significant for all the analyzed groups of regions, the nature of the influence of other control variables on different groups of regions differs. For example, for the groups “Overcoming psycho-emotional barriers”, “Optimistic regions of problematic socio-economic development” and “Regions of problematic socio-economic development”, the proxy variable of stress factors “suicide rate” (inverse relationship) turned out to be statistically significant. Thus, measures aimed at reducing the stress factors of the population require special attention on the part of the responsible authorities. First of all, it is the work in the sphere of prevention of depression and suicidal behavior development, popularization of healthy lifestyle and informing about the possibility of spread of socially significant diseases.
The influence of the indicator “number of students”, which we selected as the factor “education”, was statistically significant for all groups of regions, except “Overcoming natural-environmental barriers”. At the same time, the effect on life expectancy, in contrast to the results of previous works, which study the relationship of education with the public health level, is the opposite. These results can be explained by the fact that the indicator we selected is not in the strict sense an indicator of the education, which is absent in the statistics for the period. In this regard, for an objective interpretation of the impact of the number of students on life expectancy, more detailed research is needed, taking into account the relevant for the national economy problem of interregional mobility of students and university graduates, actualized in the works (Gabdrakhmanov, 2019; Naumov et al., 2019). Speaking of environmental indicators, we note that according to the results obtained, the selected exogenous variables significantly affect life expectancy only of the regions of the “Overcoming natural-environmental barriers” group, which is consistent with the research results (Muldoon et al., 2011).
Along with the justification of the consistency of our estimates with the results presented in the studies of other scientists, we should outline the objective limitations of the model built. The main limitations include, first, the traditional problem for Russian regional studies – the limited amount of statistical data both by the set of indicators and by the available period of analysis. More complete data would have greatly improved the understanding of the relationships under study. Second, the model did not take into account the short-, medium-, and long-term effects of increased health care expenditures, as well as changes in spending directions by year. Third, we did not decompose the differences in life expectancy by gender or age, which could also have provided interesting insights. Fourth, the model does not highlight expenditures on disease prevention, whose high impact on life expectancy has been proven in a number of papers. Finding a proxy variable for this type of expenditures could add a new determinant to the list of factors increasing life expectancy.
Thus, overcoming the highlighted limitations of the model is, from our point of view, an interesting area for further research.
Conclusion
The work offers a comprehensive toolkit for improving the health care system in regional development and management based on the clustering of regions according to the specifics of the formation of conditions for increasing life expectancy. It is worth highlighting the main scientific results of the study.
-
1. We have studied the existing theoretical and methodological approaches and research methods on the health care system as the basis for ensuring and increasing life expectancy. The review of the research works revealed a number of missing methodological aspects that objectively require improvement, including ignoring the importance of preliminary clustering of heterogeneous territories when modeling the social performance of the health care system.
-
2. We have carried out the clustering of Russian regions according to the specifics of the formation of conditions for increasing life expectancy. The database for clustering regional indicators included the following blocks: social, economic, psychological, and natural-environmental. Using the Ward method, we obtained compact and well-divided clusters, which are sufficiently amenable to economic interpretation and represent an important groundwork for further modeling.
-
3. We have formulated the research hypotheses and prepared the statistical database, influencing life expectancy of Russia’s entities, for 10 years. We used the analysis results of the literary sources, adjusted by the availability of existing statistical information on Russia’s regions, as the basis for the selection of indicators for the formalization of hypotheses.
-
4. We have used econometric modeling to evaluate the hypotheses proposed and to interpret the results obtained. We evaluated three types of models (models with fixed effects, with random effects, pooled LSM) with regard to the groups “Optimistic regions of socio-economic development problems”, “Overcoming psycho-emotional barriers” and “Overcoming natural-environmental barriers”. The obtained estimates helped to identify the specifics of the impact of individual indicators on life expectancy in different groups of Russian’s entities and outline the directions for further research.
Thus, the scientific novelty of our research is the clustering of Russia’s entities according to the specifics of forming the conditions for increasing life expectancy, which is of fundamental importance for increasing the efficiency of territorial management and planning in the field of health care. The practical significance of the study lies in the fact that the obtained results can be useful for regional and local authorities to form a set of measures to increase life expectancy.
The findings add new empirical evidence to the system of scientific works devoted to identifying the determinants of life expectancy at the regional level of the national economy, therefore supporting the discussion on prioritizing the task of health protection and longevity extension in contemporary Russia.
Список литературы Determinants of life expectancy in heterogeneous constituent entities of the Russian Federation
- Akhmeduev A.Sh. (2019). Problems of development and imperatives of reforming the health care in Russia and its regions. Natsional’nye interesy: prioritety i bezopasnost’=National Interests: Priorities and Security, 15, 8(377), 1529–1546 (in Russian).
- Akindinova N.V., Chernyavsky A.V., Chepel’ A.A. (2018). Cross-country analysis of public expenditures structure and efficiency. Voprosy ekonomiki, 12, 5–27 (in Russian).
- Andreev E.M., Shkol’nikov V.M. (2018). The relationship between mortality and economic development in Russia and its regions. Demograficheskoe obozrenie=Demographic Review, 5(1), 6–24 (in Russian).
- Anyanwu J.C., Erhijakpor A.E.O. (2009). Health expenditures and health outcomes in Africa. African Development Review, 21(2), 400–433.
- Arkhipova S.V., Dvoinikov S.I. (2018). Methodological aspects of evaluation of effectiveness in health care. Menedzher zdravookhraneniya=Manager of Health Care, 10, 23–30 (in Russian).
- Asiskovitch S. (2010). Gender and health outcomes: The impact of healthcare systems and their financing on life expectancies of women and men. Social Science & Medicine, 70(6), 886–895.
- Balina T.A., Gorbanev V.A., Stolbov V.A., Chekmeneva L.Yu. (2021). Geographical approach to studying the symbolic capital of the country from the position of the population expected life. Vestnik Udmurtskogo universiteta. Seriya: Biologiya. Nauki o Zemle=Bulletin of Udmurt University. Series Biology. Earth Sciences, 31(2), 198–208 (in Russian).
- Barro R. (2013). Health and economic growth. Annals of Economics and Finance, 14, 329–366.
- Barthold D., Nandi A., Mendoza Rodríguez J.M., Heymann J. (2014). Analyzing whether countries are equally efficient at improving longevity for men and women. American Journal of Public Health, 104(11), 2163–2169.
- Bhargava A. Jamison D.T., Lau L., Murray C.J.L. (2001). Modeling the effects of health on economic growth. Journal of Health Economics, 20(3), 423–440.
- Bloom D.E., Malaney P.N. (1998). Macroeconomic consequences of the Russian mortality crisis. World Development, 26(11), 2073–2085.
- Brainerd E., Cutler D.M. (2005). Autopsy on an empire: Understanding mortality in Russia and the Former Soviet Union. Journal of Economic Perspectives, 19(1), 107–130.
- Chekmeneva L.Yu., Balina T.A. (2019). Comparative analysis of expected duration of life in Russia and the world. Vestnik TvGU. Seriya “Geografiya i geoekologiya”, 3(27), 5–13 (in Russian).
- Chubarova T.V. (2020). Income and consumption of medical care through the lens of protested goods’ approach. Zhurnal Novoi ekonomicheskoi assotsiatsii=The Journal of the New Economic Association, 3(47), 190–196 (in Russian).
- Currie J., Moretti E. (2003). Mother’s education and the intergenerational transmission of human capital: Evidence from college openings. The Quarterly Journal of Economics, 118(4), 1495–1532.
- Demidova O.A., Kayasheva E.V., Dem’yanenko A.V. (2021). Government spending on healthcare and economic growth in Russia: A regional aspect. Prostranstvennaya ekonomika=Spatial Economics, 17(1), 97–122 (in Russian).
- Desai S., Alva S. (1998). Maternal education and child health: Is there a strong causal relationship? Demography, 35(1), 71–81.
- Erakhtina A.D. (2019). Medical investment, life expectancy and economic growth. EKO=ECO, 6(540), 8–25 (in Russian).
- Erzyleva I.A. (2020). Health of the population – the engine of economic growth of the country. Russian Economic Bulletin, 3(3), 231–235 (in Russian).
- Gabdrakhmanov N.K. (2019). Concentration of students in higher education on the map of the Russian Federation. Vestnik Rossiiskogo universiteta druzhby narodov. Seriya: Ekonomika=RUDN Journal of Economics, 27(1), 7–17 (in Russian).
- Gorchakova N.S. (2020). The role of the economy in human capacity-building. Izvestiya Saratovskogo universiteta. Novaya seriya. Seriya: Ekonomika. Upravlenie. Pravo=Izvestiya of Saratov University. Economics, Management, Law, 20(2), 134–140 (in Russian).
- Grishina V.V., Karpova G.G. (2020). Optimization of the healthcare system in the Russian Federation: Causes and consequences. Sbornik trudov konferentsii “Universitetskaya nauka: vzglyad v budushchee”, 172–175 (in Russian).
- Gupta S., Verhoeven M., Tiongson E.R. (2003). Public spending on health care and the poor. Health Economics, 12(8), 685–696.
- Jaba E., Balan C.B., Robu I.-B. (2014). The relationship between life expectancy at birth and health expenditures estimated by a cross-country and time-series analysis. Procedia Economics and Finance, 15, 108–114.
- Kalashnikov K.N. (2011). Healthcare and the economy of the region. Problemy razvitiya territorii=Problems of Territory’s Development, 1(53), 61–66 (in Russian).
- Kaneva M.A. (2019). Effect of health capital on the economic growth in Russian regions. Region: ekonomika i sotsiologiya=Region: Economics and Sociology, 1(101), 47–70 (in Russian).
- Kislitsyna O.A., Chubarova T.V. (2021). Estimation of the healthcare system in Russia: Experience in building a regional rating. Vestnik Instituta ekonomiki Rossiiskoi akademii nauk=The Bulletin of the Institute of Economics of the Russian Academy of Sciences, 3, 35–71 (in Russian).
- Kolosnitsyna M.G., Kossova T.V., Sheluntsova M.A. (2019). Factors of the life expectancy increase: Country-level cluster analysis. Demograficheskoe obozrenie=Demographic Review, 6(1), 124–150 (in Russian).
- Komarova A.V., Kritsyna E.A. (2012). On the proportion on human capital in GRP of Russian regions. Vestnik NGU=Vestnik NSU, 12(3), 5–14 (in Russian).
- Kossova T.V., Kossova E.V., Sheluntsova M.A. (2017). Estimating the impact of alcohol composition on mortality and life expectancy in Russian regions. Ekonomicheskaya politika=Economic Policy, 1, 58–83 (in Russian).
- Krivenko N.V., Ivanov V.M., Kriventsova L.A. (2019). Evaluating the efficiency of health care as a factor of sustainable socio-economic development in the region. Natsional’nye interesy: prioritety i bezopasnost’=National Interests: Priorities and Security, 15, 12(381), 2223–2241 (in Russian).
- Lebedeva-Nesevrya N.A., Kir’yanov D.A. (2012). Suicide and alcohol abuse: Correlation-analysis on the materials of the research in Perm region. Vestnik Permskogo universiteta. Filosofiya. Psikhologiya. Sotsiologiya=Perm University Herald. Series “Philosophy. Psychology. Sociology”, 4, 107–110 (in Russian).
- Masset E. (2011). A review of hunger indices and methods to monitor country commitment to fighting hunger. Food Policy, 36, 102–108.
- Mikhaylova Yu.V., Ivanov I.V., Shikina I.B. et al. (2016). Methodological aspects of carrying out independent assessment of medical organizations of the territorial subjects of the Russian Federation providing outpatient medical care to the catchment area. Sotsial’nye aspekty zdorov’ya naseleniya=Social Aspects of Population Health, 3. Available at: https://cyberleninka.ru/article/n/metodologicheskie-aspekty-provedeniya-nezavisimoy-otsenki-meditsinskih-organizatsiy-subektov-rf-okazyvayuschih-meditsinskuyu (accessed: January 14, 2023; in Russian).
- Muldoon K.А., Galway L.P., Nakajima M. et al. (2011). Health system determinants of infant, child and maternal mortality: A cross-sectional study of UN member countries. Globalization and Health, 7(1). Available at: https://www.researchgate.net/publication/51739233_Health_System_Determinants_of_Infant_Child_and_Maternal_Mortality_A_Cross-Sectional_Study_of_UN_Member_Countries (accessed: January 19, 2023).
- Naumov I.V., Dubrovskaya Yu.V., Kozonogova E.V. (2020). Digitalisation of industrial production in the Russian regions: Spatial relationships. Ekonomika regiona=Economy of Region, 16(3), 896–910 (in Russian).
- Orlov E.M., Sokolova O.N. (2010). Category of efficiency in the healthcare system. Fundamental’nye issledovaniya=Fundamental Research, 4, 70–75 (in Russian).
- Panasyuk M.V., Dasayeva R.D. (2014). Issues of improving the economy of healthcare in the Russian regions. Aktual’nye problemy ekonomiki i prava=Russian Journal of Economics and Law, 2, 61–67 (in Russian).
- Pavlova I.A., Gumennikov I.V., Monastyrnyi E.A., Sharma D. (2018). What is behind composite wellbeing indices? Vestnik nauki Sibiri=Siberian Journal of Science, 4(31), 230–254 (in Russian).
- Potapchik E.G. (2020). How much should the state allocate to health? Does the international experience give a definite answer? Sotsial’nye aspekty zdorov’ya naseleniya=Social Aspects of Population Health, 66(4), 1–10 (in Russian).
- Reprintseva E.V. (2020). Analysis of indicators of the hospital network of the Russian healthcare system. Azimut nauchnykh issledovanii: ekonomika i upravlenie=Azimuth of Scientific Research: Economics and Administration, 2, 281–283 (in Russian).
- Roberts J. (2003). Poverty Reduction Outcomes in Education and Health Public Expenditure and Aid. Working Paper 210. London: Overseas Development Institute. Available at: http://https://www.files.ethz.ch/isn/96850/wp210.pdf (accessed: January 19, 2023).
- Rosenzweig M.R., Schultz T.P. (1982). Child mortality and fertility in Colombia: Individual and community effects. Health Policy and Education, 2(3-4), 305–348.
- Santeramo F.G. (2015). On the composite indicators for food security: Decisions matter! Food Reviews International, 31(1), 63–73.
- Skipin D.L., Yukhtanova Yu.A., Kryzhanovskii O.A., Tokmakova E.G. (2022). Life expectancy in Russia’s regions. Ekonomicheskie i sotsial’nye peremeny: fakty, tendentsii, prognoz=Economic and Social Changes: Facts, Trends, Forecast, 15(2), 156–171 (in Russian).
- Starodubov V.I., Son I.M., Leonov S.A. et al. (2013). Results of the assessment of some performance indicators of medical organizations in 2010. Sotsial’nye aspekty zdorov’ya naseleniya, 1(29) (in Russian).
- Stolbov A.V. (2016). Modern culture and regional human capital: Theoretical foundations of innovative cooperation. Innovatsionnoe razvitie ekonomiki: tendentsii i perspektivy, 1, 498–506 (in Russian).
- Swift R. (2011). The relationship between health and GDP in OECD countries in the very long run. Health Economics, 20(3), 306–322.
- Tatarkin A.I., Krivenko N.V., Kuznetsova N.L. (2015). Dialectics of rational change management in regional social systems. Ekonomika regiona=Economy of Region, 2(42), 125–136 (in Russian).
- Thomas D., Strauss J., Henriques M. (1990). Child survival, height for age and household characteristics in Brazil. Journal of Development Economics, 33(2), 197–234.
- Tuaeva L.A., Sugarova I.V. (2013). Cost effectiveness of the health system. Terra Economicus, 11(3-3), 94–97 (in Russian).
- Ulumbekova G.E., Ginosyan A.B. (2021). Rating of the effectiveness of healthcare system in the regions of the Russian Federation, in 2019. ORGZDRAV: Novosti. Mneniya. Obuchenie. Vestnik VOUZ=Healthcare Management: News, Views, Education. Bulletin of VSHOUZ, 1(23). Available at: https://cyberleninka.ru/article/n/reyting-effektivnosti-sistem-zdravoohraneniya-regionov-rf-v-2019-g (accessed: January 19, 2023; in Russian).
- Ulumbekova G.E., Ginoyan A.B., Chaban E.A. (2016). Quantitative analysis of the factors influencing the state of health of the population in the Russian Federation. Meditsinskoe obrazovanie i professional’noe razvitie=Medical Education and Professional Development, 2(24). Available at: https://cyberleninka.ru/article/n/kolichestvennyy-analiz-faktorov-vliyayuschih-na-sostoyanie-zdorovie-naseleniya-v-rossiyskoy-federatsii (accessed: January 13, 2023; in Russian).
- Ulumbekova G.E., Prokhorenko N.F., Ginoyan A.B., Kalashnikova A.V. (2019). A system approach to achieving the national goal of increasing life expectancy to 78 years by 2024. Ekonomika. Nalogi. Pravo=Economics, Taxes & Law, 12(2), 19–30 (in Russian).
- Van Den Heuvel W.J.A., Olaroiu M. (2017). How important are health care expenditures for life expectancy? A comparative European analysis. Journal of the American Medical Directors Association, 18(3), 9–12.
- Vlasyuk L.I., Stroev P.V. (2017). A methodology for determining the level of human capital development and its differentiation in the regions of Russia. Ekonomika. Nalogi. Pravo=Economics, Taxes & Law, 10(4), 86–95 (in Russian).
- Wang Y., Ni C. (2015). The role of the composition of the human capital on the economic growth: With the special effect among provinces in China. Modern Economy, 6, 770–781.
- Zaitseva N.V., Onishchenko G.G., Popova A.Yu. et al. (2019). Social and economic determinants and potential for growth in life expectancy of the population in the Russian Federation taking into account general differentiation. Analiz riska zdorov’yu=Health Risk Analysis, 4, 14–29 (in Russian).
- Zhang W.-B. (1999). Sinergeticheskaya ekonomika: Vremya i peremeny v nelineinoi ekonomicheskoi teorii [Sinergetic Economics: Time and Change in Nonlinear Economics]. Moscow: Mir.
- Zhukova A.K., Silaev A.M., Silaeva M.V. (2016). Spatial analysis of life expectancy in Russian regions. Prostranstvennaya ekonomika=Spatial Economics, 4–5, 112–128 (in Russian).
- Zubarevich N.V. (2009). Regional development and regional policy in Russia during ten years of economic growth. Zhurnal Novoi ekonomicheskoi assotsiatsii=Journal of the New Economic Association, 1–2, 160–174 (in Russian).