Estimating the Effects of Voice Quality and Speech Intelligibility of Audio Compression in Automatic Emotion Recognition
Автор: A. Pramod Reddy, Dileep kumar Ravikanti, Rakesh Betala, K. Venkatesh Sharma, K. Shirisha Reddy
Журнал: International Journal of Image, Graphics and Signal Processing @ijigsp
Статья в выпуске: 3 vol.15, 2023 года.
Бесплатный доступ
This paper projects, the impact & accuracy of speech compression on AER systems. The effects of various codecs like MP3, Speex, and Adaptive multi-rate(NB & WB) are compared with the uncompressed speech signal. Loudness enlistment, or a steeper-than-normal increase in perceived loudness with presentation level, is associated with sensorineural hearing loss. Amplitude compression is frequently used to compensate for this abnormality, such as in a hearing aid. As an alternative, one may enlarge these by methods of expansion as speech intelligibility has been represented as the perception of rapid energy changes, may make communication more understandable. However, even if these signal-processing methods improve speech understanding, their design and implementation may be constrained by insufficient sound quality. Therefore, syllabic compression and temporal envelope expansion were assessed for in speech intelligibility and sound quality. An adaptive technique based on brief, commonplace words either in noise or with another speaker competing was used to assess the speech intelligibility. Speech intelligibility was tested in steady-state noise with a single competing speaker using everyday sentences. The sound quality of four artistic excerpts and quiet speech was evaluated using a rating scale. With a state-of-art, spectral error, compression error ratio, and human labeling effects, The experiments are carried out using the Telugu dataset and well-known EMO-DB. The results showed that all speech compression techniques resulted in reduce of emotion recognition accuracy. It is observed that human labeling has better recognition accuracy. For high compression, it is advised to use the overall mean of the unweighted average recall for the AMR-WB and SPEEX codecs with 6.6 bit rates to provide the optimum quality for data storage.
Speech Compression, speech intelligibility, emotion recognition, CER
Короткий адрес: https://sciup.org/15018763
IDR: 15018763 | DOI: 10.5815/ijigsp.2023.03.06
Текст научной статьи Estimating the Effects of Voice Quality and Speech Intelligibility of Audio Compression in Automatic Emotion Recognition
Speech Enhancement may improve the perceptual nature of correspondences, but it doesn’t ensure an improvement in discourse undesirability. In boisterous situations, speech up- grade (or commotion decreases) the calculations are used regularly to improve the nature of speech. The overall aim of the speech enhancement algorithm is to evaluate the range of the clamor sign or ascertain the clean voice signal to improve the general sign-to-noise ratio. (SNR). Tragically, the in general (time-freq) SNR isn’t profoundly connected with coherence. At the end of the day, an improvement in SNR doesn’t build appreciation. The general voice quality and speech intelligibility correlation is higher with an improvement by utilizing recurrence area SNRs portioned by the view of the human sound-related framework. In-Line, we have presented the impact of speech compression using different codecs compared over compressed and uncompressed data and compressed error rate (CER) is analyzed to conclude compression quality with state of art. In the second section, literature survey next to it a brief discussion about speech corpus is discussed followed by various available compression codecs in the fourth section. In the fifth section the experimental design is discussed next to this results are presented, here rank randomization is used for quality evolution and is concluded with the last section. In our previous work [1] we studied spectral and temporal features, their combinations without affecting the performance of the system, and in [20] most salient 3 independent wav, mp3, and SPX encoding methods, dimensions such as Mel-frequency Cepstral Coefficients. (MFCC), wavelet and Mel- Frequency Discreet wavelet transform. (MFDWT) [2] are modeled and classified by KNN, Support Vector Machine. (SVM), Hidden Markov Model. (HMM) were experimented. Better convergence rates are traditional. Having higher voice bias than other details is suppressed during voice and speech compression Automatic Emotion Recognition (AER)’s lower precision. The variance is noted, that high recognition rate with bit- rate higher in low distortion conditions. Three Sound Preassure Levels in both quiet and noise were used to examine the intelligibility, pleasantness, and naturalness of speech that had been processed linearly versus nonlinearly by its three listeners. The vast majority of the linearly processed circumstances were used to gauge how enjoyable speech in noise was, however one of the three listeners favoured the nonlinear processing at all Sound Preassure Leves. We tested speech intelligibility and sound quality for a variety of syllabic compression and expansion settings while systematically varying the number of independent frequency bands and the compression/expansion ratio. Short, everyday phrases were used to test speech perception in relatively stable noises and with a single competitive speaker. The audio quality of six musical segments and calm discourse was evaluated using a rating scale. Because the signal processing was done off-line, we were able to design a system with no time delays. As a result, instead of trailing behind, as most real-world compression methods do, the amplification was optimally matched to the actual input envelope level at each time sample. Therefore we designed a model based on the above constraints and selected Telugu dataset and the reasons for choosing the Telugu dataset as follows, (1) There aren't many standard Telugu local databases. This is one of the typical things to consider when developing the information resource. (2) In order to incorporate the distinctive can be found for different vocal terms inside the specific areas of Telugu speaking, we constructed a dataset using post-graduate and basic learners. Five individuals, three of them were women, freely took part, ranging in age from seventeen to be able to twenty-one years. Current research indicates that these age groups fall below harsh variety of situations, which is why they are at the back of the age grouping defendant. We investigated speech intelligibility and sound quality in a variety of syllabic compression and expansion settings in which the number of independent frequency bands and the compression or expansion ratio were systematically adjusted. Speech intelligibility was tested in steady-state noise with a single competing speaker using everyday sentences. The sound quality of four artistic excerpts and quiet speech was evaluated using a rating scale. Speech intelligibility was tested in steady-state noise with a single competing speaker using everyday sentences. The sound quality of four artistic excerpts and quiet speech was evaluated using a rating scale. Because the signal processing was carried out off-line, we were able to build a system without temporal delays. Therefore, the amplification was optimally matched to the actual input envelope level at each time sample rather than slightly lagging behind as in most real-world compression techniques.
2. Litrature Review
Multidimensional amplification is used in many hearing devices on the marketplace currently. Attenuation is typically material to a certain receiver input known as the hinge point. The input is magnified by a threshold amount above this level. Trimming is a common complication of nonlinear amplification in which the threshold point corresponds to the maximum output level. However, the goal of many other multidimensional signal - to - noise application domains is to compensate for listeners with sensorineural hearing loss who have a narrower dynamic range. Nonlinear amplification has reportedly been used in hearing devices from about 1936, but these applications were essentially output compressors, according to Caraway and Carhart in 1967.
There were no audio aides upon this market at the time they authored their study that "had compression working over all or at least a major part of the operational range". Researchers concluded as a result that "the anomalies in the noise contour produced into being by enlistment don't really render the listener better proficient of extracting information from compressed speech than from uncompressed speech. Researchers concluded as a result that "the anomalies in the noise contour produced into being by enlistment don't really render the listener better proficient of extracting information from compressed speech than from uncompressed speech" [3]. In contrast villchur et. al [2, 3] claimed substantial gains in speech acknowledging for 6 audiences with sensory function difficulties using a device which implemented compression in 2 distinct frequency ranges with fully customized compression quantitative relation varying between 2 and 3. Aside from enhanced CVC intelligibility, he discovered that his 6 audiences desired compressed speech to unprocessed speech. Villchur only compared linear amplification with frequency shaping against the combination of compression and frequency shaping, which makes it difficult to understand his findings. Especially contrasted to sequential augmentation, Lippman et al.[6] discovered that compressing typically results in a modest reduction in speech intelligibility. Only when the voice content had considerable level changes or when the input level was low did compression perform well. Nabelek 1983 [7] In addition to echoing, noise filtering, and apex ripping, a wide range of compression ratios and attack/release timings were explored. Consequently, nabelek came to the conclusion that compression is only beneficial to speech intelligibility for specific compression levels, such as ratio ( snr, attack/release periods, as well as at higher S/N proportions. According to Bustamante and Braida's 1987 [8] study, the compression conditions at best yielded speech-intelligibility scores comparable to the condition with linear amplification, albeit compression processing maintained its score over a wider range of input levels. With the obvious exception that the fixed amount of linear amplification was insufficient for the experiments' lowest input level, 55 dB SPL, Levitt and Neuman (1991) [9] found that none of their fundamental compressing approaches improved linear amplification. By Mare' et al. in 1992, three alternative compression curves were contrasted with linear amplification. Both expansion and compression will affect the clarity of the voice as well as the sound quality. Any signal processing approach must properly execute a hearing aid's pleasing sound quality; hearing aids with poor sound quality are unlikely to be accepted by listeners with hearing loss. We shall mention three instances where sound quality for amplitude compression and expansion has not been extensively examined, in contrast to assessments of speech intelligibility. Byrne and Walker (1982) [10] evaluated the compression and expansion capabilities of their system as well as the speech understandability. The intelligibility, pleasantness, and naturalness of speech that had been processed linearly versus nonlinearly by its three listeners were compared using three SPLs in both quiet and noise. When evaluating how pleasant speech in noise was, the vast majority of situations that were chosen were those that had been treated linearly, however one of the three listeners preferred the nonlinear processing at all SPLs. For a number of syllabic compression and expansion settings, we tested speech intelligibility and sound quality while systematically varying the number of independent frequency bands and the compression/expansion ratio. Short ordinary phrases were used to test speech perception in relatively stable noises and with an unique competitive speakers. Using a rating-scale approach, the audio quality of six musical segments and calm discourse was assessed. We were able to design a system with no time delays because the signal processing was done off-line. As a result, rather than lagging behind, as most real-world compression methods do, the amplification was optimally matched to the actual input envelope level at each time sample.
3. Speech Corpus
Heretofore, Emotion recognition techniques are foreseen to make use of recorded data along with certain classifiers. About, designing such techniques the annotated data source is a pre-requisite. The particular training plus evolution count upon the viability, simply as the obvious aftereffects of emotions recognizer, are to a higher degree subject matter to the directories utilized. A sum-up of emotional conversation database is located within putlik [9, 12] thoughts exactly where more than forty-eight databases are explored concerning acquaint emotions, info assortment technique, vocabulary, content, discourse kind (common, reenacted or even evoked) along with other physiological signs contained within the particular accounts which can be used for emotion recognition. Considering the method that review has been done more compared to 48 databases, one may accept that this absence of talk information is within truth false. However, in spite associated with the occurrence connected with these databases, are not almost all publicly available.
You can find three distinctive enthusiastic databases essentially grouped as Acted, motivated—enthusiastic, and Spontaneous or perhaps Natural. The Served dataset is noted by the specialist and non-professional celebrities. Each actor provides to speak typically the given utterance in addition to they are annotated according to the desired feeling. Another set regarding databases contains motivating—inspiring emotions. These inner thoughts are neither real nor reproduced. They will are initiated for the members by different modalities, like stories, films, expanded reality, or on the other hand imagining. The very last established are databases regarding unconstrained discourse which often contains real thoughts.
Actual emotions are the most attractive for enthusiastic web directories. In any case, making this particular kind of story is challenging since proper emotions are amazingly unusual and their very own term is usually extremely brief. Also, correct feelings may become deliberately protected upward and also changed simply by individuals, as for every their notice of interpersonal principles and their privacy. Moreover, presently there are a couple of thoughts that never enter into a particular condition, plus because of in the order to scanty details class can’t become carried out. The unconstrained directories regularly include the high determine associated with unbiased expression and only a tiny arrangement connected with enthusiastic ones. Since the result of these types of difficulties in recording authentic information, the majority of the available web directories consist of acted discussion emotions, which shows they are generally not genuineyet rather intentionally communicated.
A couple of techniques were used for the grouping of unconstrained enthusiastic databases. On one hand, the individuals had human examiners and acted in interviews, phone conversations, or were adequately connected with social occasions. On the opposite side Human-Computer -Interaction. (HCI) HCI interfaces as exchange conveyance frameworks. The ongoing situations sony AIBO [9, 10] driving recreations in virtual conditions [15], Natural [16], PC shooting match-ups, or tremor emulators[17].
Generally—typically, the served feeling dataset is progressively smaller in size with essential classes related to feeling discussed in Robert Plutchik [12, 14, 15] are just matches related to conceptualized expressions of those sentiments. The realness is the way that affirmation that inside authentic data of musings occurs when in the while and what’s more account isn’t good concerning an assortment of sentiments, presently in addition to again unpleasant names, for example, certain or not-sure are utilized. There are normally in like manner endeavors to distinguish significant level feelings like distress and disappointment[26], anxiety [18], bothering, and stress [24]. The information source with served discourse offers a higher acknowledgment cost due to high excitement in addition to the general execution in recognition of sentiments is much more. Acted musings could be effectively separated just by people through genuine sentiments, The specific current investigations may extend that will acted contemplation could be handily recognized through common emotions discourse. In the current scenario emotion recognition from the speech is moved from acted to continuous/spontaneous speech data and is also widely encouraged by research scholars. when it comes to spontaneous data, capturing emotions more accurately challenges continuous speech and has the best recognition accuracy. The major challenge comes with pre-processing the second duration of the speech signal, the third is different background noises which makes the system more complex.
3.1 Telugu database
4. Audio Coding Formats
Included in our experiments, we have now created the Telugu language database, in addition, it can be the subsequent highest speaking Native Indian native indigenous vocabulary (well-known southern Native Indian Language) just like a major the necessity to make certain that because an important component quality is usually managed for correct acceptance of emotions. The particular standard aspects with regard order to develop the information resource are subsequent, (1) there are usually virtually no standard Telugu local databases. (2) within the particular areas of Telugu speaking says the peculiar can be found the same which implies for different vocal words, in-order to incorporate we developed a dataset by making use of post-graduate and basic learners 5 people and 3 female participated voluntarily as well as come in typically the particular age of seventeen to be able to 21 numerous yrs. The reason why at typically the rear of picking age group party is always that current scientific studies announce these kinds of era groups drops beneath ruthlessness in various conditions. All audio speakers are well certified and have inside order to communicate 15 phrases each in 5 diverse emotions with consideration to the recording. Typically whole documenting method continues to end up being carried out over an electronic music workstation plus Shure SM57-LC Cardioid Dynamic Mic will be applied for documenting possessing a sampling level of recurrence of sixteen kHz plus is usually coded along together with 24-bit and 32-bit carry on. The below Figure 1depicts the information for speakers who were involved during the process which is referred to as speaker ID on the axis and the other hand the number of sentences pronounced is shown. Table 1 describes the overview of Telugu-dataset subset samples used for classificationin the model.
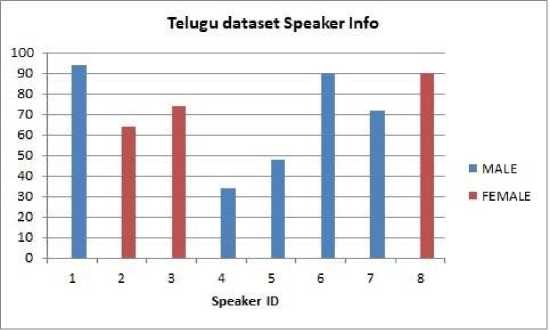
Fig. 1. Telugu database speaker Information
Table 1. shows Telugu-dataset samples used for classification
Angry |
Sad |
Neutral |
Happy |
Fear |
surprised |
bor |
||
AER |
MALE |
96 |
63 |
51 |
76 |
59 |
42 |
34 |
FEMALE |
42 |
27 |
21 |
32 |
25 |
18 |
14 |
|
Human Labeling |
Male & Female |
4 |
3 |
2 |
3 |
3 |
2 |
2 |
In a correspondence mobile phone, a codec (Coder/Decoder) is among the fundamental constructions that hinder the powerful transmission of information. Source code relates to the particular utilization associated with variable-length rules for reducing images towards the absolute minimal to respond to the transmitting data in particular. The lossless information stress reduces to chunks by understanding and getting purged related to factual repeating. Within any kind of case, information pressure will be susceptible to space-time di- verse character tradeoff (like the particular amount associated with pressure, the particular way associated with measuring escarre presented while utilizing lossy details stress, computational sources necessary to bundle and uncompress the specific information). An ideal strategy standard connected with the codec, consequently, is based on persistent bartering associated with the specific previously pointed out specific tradeoff which means to maintain upwards the Quality of service requirements.
In March 2001 tusentalet, the 3rd Generation Partnership Project (3GPPTM) recommended the specific particulars for that Adaptive Multi-Rate Wideband (AMR -WB) coding computation, as the feature associated with 3GPPTM Launch 5. The particular ITU-T Research Group sixteen affirmed the similar Wide-band (WB) coding computation as Suggestion G.722. 2 as well as annexed in 2002 January[19].
-
A. AMR-WB
The first codec used in Cellular Services (3GPP) and Wireless (ITU-T) services is a G.722.2/AMR- WB. AMR WB/G.722.2 was introduced for teleconferencing and voice-over packet imple- mentations at the ITU to accommodate a wide variety of bit rates between 6.6 and 23.85 kbps. The compulsory broadband speech codec in GSM and WCDMA networks is AMR- WB and is included in the PacketCable 2.0 Requirements of the CableLabs system. The G.722.2/AMR-WB facilitates dynamic network adaptation by using lower bitrate and im- proving audio quality while maintaining a network overload or degradation.’ [ 1 3 , 1 4] In GSM, connect adjustment is utilized to advance the apparent transmission quality depen- dent on estimation reports of the radio channel quality. AMR-WB is required in 3GPPTM for MMS, PSS , MBMS and Packet-Switched Conversational Services when 16 kHz exam- ined discourse is utilized. Notwithstanding 3GPPTM remote applications [22], further ITU-T applications focuses with centralized normalization, including Public Switched Telephone Network. (PSTN), Voice over Internet Protocol (VoIP), Internet applications, and Integrated Services Digital Network (ISDN) wideband communication, and sound/video remotely coordinating. For broadband speech and Multimedia Service, the codec needed for AMR-WB are GSM and WCDMA networks for the wideband sample (with a sample frequency of 16 kHz). These include Multi Media Messaging Service (MMS), IMS Messaging and Pres- ence Services, Packet-Switched Streaming (PSS) (PoC). Other features include VoIP, meet- ing, telephony for Wi-Fi, satellite telephony, video telephony, internet broadcasting, audio recording and playback, voicemail and storage notes, talk environment, multimedia,real time communication software, wireless radio, etc.
-
B. AMR-NB
Adaptive multi-rate 3GPP [23] was ideal for portable 1998 mobile communication with narrowband language codecs. The codec resides on each 20 ms edge of 8 kHz tested dis- course signals and generates bundled piece streams with bitrates ranging from 4.75 kbps to 12.2 kbps.. The bit-rate can be changed at a 20 ms outline limit. The codec utilizes ACELP strategy to pack discourse at all piece rates. The codec gives VAD and solace clamor age CNG calculations for a decrease in bit rate, and a natural bundle PLC calculation for taking care of edge deletions. The codec was essentially created for versatile communication overGSM and UMTS systems.
-
C. Adaptive Multi-Rate (AMR) codec
The spectrum of available GSM channel bit rate between the speech coding are modified us- ing AMR-codec and the channel code (22800 for the full-rate or 11400 bps for the half-rate) allowing the optimal use of radio properties. The device must calculate the channel quality for uplink codec mode changes, detect and submit the right codec mode to the Mobilile Station (handset, etc.) over the Air interface, for the current spreading requirements. The Mobile Station needs to calculate the output of the downlink channel and send quality data to the system for downlink codec modification. This detail is used to characterize a codec mode ’proposed.’ Each link can use a different codec mode but it is compulsory to use a similar channel mode for all connections (either full-rate or half-rate). The Radio Resource mode is selected by the executives: it is performed on the ring or during a cell transmission. During a call as a component of the channel conditions, the channel type can also be change.
-
4.1 MP3 compression
The MP3 is a sound framework for the advanced sound configuration which utilizes a lossy information pressure position. The mp3 is a typical arrangement for gushing, stockpiling, what’s more, the standard of computerized sound pressure, or move to playback the music on the soundest advanced players. It has been basically intended to diminish the memory size utilized for information stockpiling by the factor of at least ten contrasted and the first size, with keeping a sound quality is about equivalent to uncompressed sound quality for nearly audience members. The MP3 document can be made at sequential piece rates, including higher or lower coming about quality. The pressure works by diminishing the exactness of specific pieces of sound that are viewed as past the sound-related goals capacity of a great many people.
This technique is generally alluded to as perceptual coding. It utilizes psycho acoustic models and the Mel scale to dispose of or decrease the accuracy of parts less discernible to human hearing and afterward records the rest of the data in an effective way. Table 2 provides the summary of bit-rate the audio compression codec used in this work. speex(SPX) allows a rage of 2.15 -24.6 over NB and 4-44.2 with WB with a delay of 20ms and 10 ms in NB and 14ms WB. AMR- WB is operated with a range of 6.6kbps to 23.85kbps with frame delay of 20ms and look ahead of 5 ms . MP3, a MPEG-1/MPEG-2 of audio layer III 8kbps - 320kbps and wav with 256kbps. Figure 2 shows Codec Quality
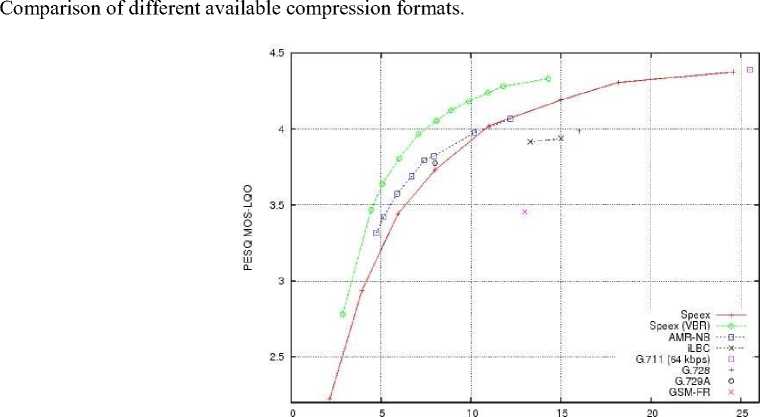
Bitrate (kbps)
Fig. 2. Codec Quality Comparison
Table 2. Summary of bit-rates for selected audio codec’s
Name |
Speex |
AMR-WB |
MP3 |
wav |
1. Introduced year |
2003 |
2001 |
1993 |
1991 |
2. compression |
Yes |
Yes |
Yes |
No |
LossLess |
No |
No |
No |
- |
3. bit-rate(kbps) |
6.6, 11.11, 22.09 |
6.60, 8.85, 12.65, 14.25, |
8, 16, 24, 32, 64, 96 |
256 |
15.85, 18.25, 19.85, 23.05 and 23.85
4.2 Speex
Speex, which the Xiph.Org Foundation started as a corporation in 2002, is a loss codec too. In comparison to MP3, it implements a by-union examination technique by which linear coefficients of pre-coding (LPC) are sent to the discourse, and then a blend is done to re- fresh the talk signal, evaluated with the code-excited linear prediction. (CELP) measure. TheSpeex code’c requires bit -rates between 3.95kbps and 44.2kbps.
5. Experiment Design
5.1 MoS5.2 CER
5.3 UAR
MOS is a mathematical component of a calculation about the general character of an occurrence or experience made by humans. Mean Opinion Score in media relations is an indicator of the essence and content of speech and video meetings. These values have been commonly determined for 1 (poor) to 5 (excellent) and have been obtained using the POLQA P.874, an ITU-T standard [24]. Meaning views Different other unusual borders are natural. While mean opinion scores were initially obtained from master eyewitness tests, an empirical calculation is also used to construct a MOS today.. Conventionally, a Mean Opinion Score can be uti- lized anyplace human emotional experience and sentiment is helpful. Practically speaking, it is frequently used to pass judgment on digital approximations. Usually utilized spaces where Mean Opinion Score is applied incorporate static compression techniques on image (for example JPEG, PNG,GIF), sound codecs (for example, 3gp,m4r, MP3, Vorbis,wav, AAC) and video codecs (for example H.264,MPEG-4, AVC,VC1). It is likewise generally utilized in gushing meetings where system impacts can corrupt interchanges quality. Today, audio tracks and video clip communications isn’t have scored by a -panel of people, but by sim- ply a amount of methods (Objective Measurement Methods) that make an effort to approx. ITU-T’s P.800.1[19] talks about goal and abstract scoring of handset transmission quality, while proposals, for example, P.863 and J.247 spread discourse and video quality [25], individually. POLAQ supports two working modes narrow-band (ranges from 300Hz-3400Hz) and super-wide-band (ranges from 50Hz- 1400Hz) [24] Relating to both methods most of the prediction algorithm in fact reaches a vividness stage at the particular value. Here weused super-wideband mode with a saturation point 4.75.
Next to MoS compressed Error Rate is employed[23]. Using this technique the difference between original and compressed spectrogram s is/are obtained which ranges between 1 and 0, ”no conflict” and ”maximum conflict”. With the normalized spectrograms and the CER, calculates that the absolute error on each window is determined by the root mean squared (RMSE) error, which is then recorded for all windows. The overall maximum issue is split between the total samples partitioned and the effects compared over each degraded codec talk sample and the bit rate.
/; , ■-'
" n(n2-1)
The Unweighted Average Recall [21] is used to measure the overall level class analysis. In the current context, the emotions of predicted classes and human labeling results are calcu- lated in line with the original known emotions.For each class of labels the UAR is measured and then multiplied for all groups of human labeling UARh.The UAR is determined for each validation step in the emotional recognition experiment and then multiplied over all steps. UARa. The statistical measure of the strength of a ordinal and/or relationship between two sets of data is extracted with Spearman’s rank correlation coefficient (Rs) [26] defined equation 1.
RMSE =
Д1
( Predicted j| — Actual j) N
X Ra and Y Ra represents ranks of X and Y respectively. The sum of differences is extracted as S d = ( X Ra Mx ) ( Y Ra My ), and My are the difference between the orginal ranks and mean of ranks. The error rate was calculated for each acoustic character using root mean squared error(RMSE) derived using equation 2 and compression error ratio using absolute error is derived from compression of speech signals with different codec and connected original signal with equation 3.
ERC
= Z
(RMSE(t)) m
Spearman rank correlation factor
The association between two ordinal ones is determined by the Spearman rank correlation factor (Rs) and/or scaled metric calculation [27] .Values below 0 usually indicate a reverse dependence, 0,2 - 0,5 - 0,8 - 0,2 - 0,2 - 0,5 from 0 to 0,2 weak - zero reference, 0. Relation-ship and beyond 0.8 values are with good association.
-
5.4 Human Labeling
We, utilized Human Labeling for examine impact over compressed data along the human capacity to perceive emotions. This labeling is carried out with five native Telugu speaking participants, of which 3 male and 2 female,analogous to this experiment [28], was conducted. No member in the team had experienced this type of study. Toward the start of the naming, all members needed to experience a preparation stage by tuning in to chosen tests of one speaker having the equivalent sentence recorded in each of the five emotion states. Thus, the comprehension of how the various emotions are expressed by the on-screen characters was guaranteed for all members. Tests of the preparing speaker were not utilized in the primary analysis. A random of 426 samples are given to all participants and is fixed. The participants could listen each example a few times, yet they couldn’t reconsider them and the entire procedure took on approx. 100 minutes.
Sensorineurally impaired ear’s limited vibrant range is accompanied by a steeper-than-average increase in perceived loudness. Fundamentally, it has been assumed that externally compensating for this defect with a compression amplifier will significantly improve speech understanding. However, this is not always the case. To determine whether the faster increase in loudness is accompanied by greater sensitivity to level variations such as those seen in modulated signals, it may be useful to first assess just-noticeable differences in SPL in listeners with sensorineural hearing impairment. These results present a further conundrum: in the presence of steady-state noise, one nonlinear amplification technique helped certain listeners, yet in the presence of a single competing speaker, a second nonlinear amplification technique helped the same listeners. This needs a highly complex signal analysis before processing, assuming such an analysis is even conceivable in a practical hearing aid, and whether the minor gains in SRT are realistic given the poor reliability of this analysis. But at this time, our findings do not provide a universal curative for all listeners with sensorineural hearing loss. The experimental results presented here have the advantage that, compared to linear amplification, there exist processing circumstances in which both speech intelligibility and sound quality scarcely degrade. If the comfort gains outweigh the performance losses, such as when the listener no longer has to manually adjust the amplification when there are significant volume changes, then nonlinear processing of the kind used in our research may be evaluated for use in real-world applications. In the first comparison, The Spearman’s Rank Relation Key is a technique to sum up the performance and position of the relation between two variables (negative or positive). The outcome will consistently be among 1 and le 1. Strategy - computing the coefficient. Make a table from your information. The human labeling and predicted classes are evaluated separately before conducting comparison of methods.
-
A. Rank Randomization
For the quality evaluation the Rs was used. Initial, an immediate correlation among MOS and CER was directed. A while later, the mean UAR of the human naming and the pro- grammed acknowledgment test were fused, to state if and how well these measures asso- ciate The mean values of unweighted average recall, mean opinion score and compressed error ratio is presented in Table 3. By directing a top-down approach ranking the positions inside measures were resolved by higher-ranking the most significant value with the best position ”1”. Spearman’s Rho(example for rank randomization test) is a non-parametric test used Measuring the consistency of the relationship between two variables, where the value r = 1 methods an optimal positive correlation, and the value r = - 1. For example, in these lines, you will use this test to see how the age and size of individuals are related (they will be - the taller individuals are, the greater their feet are probably going to be). The compari- son between MoS & CER, Spearman’s Rho evidenced r s = 0.99798, p (2-tailed) = 0. which shows the perfect fit of selected database. X R a and Y R a mean=50 and std.dev= 28.72 with a combined covariance of 823.33. This shows the clear relationship between the emotions. The relationship is derived using equation 1 where d is difference in ranks. N is number ofpairs of data.
Table 3. Quality cost of MoS and CER over mean of all emotions
Code/bitr AMR. |
AMR. 12.6 |
AMR. 23.85 |
MP3. 8 |
MP3.16 |
MP3.24 |
MP3. 32 |
MP3.96 |
SPX. 6.6 |
SPX. 11.11 |
WAV. 256 FLAC |
Opus |
||
ate |
6.6kbps |
||||||||||||
UAR(A) |
7.376 |
7.611 |
7.517 |
7.019 |
6.993 |
7.559 |
7.967 |
7.934 |
7.783 |
7.429 |
7.528 |
7.725 |
8.1136 |
UAR(H) |
8.946 |
9.082 |
9.031 |
8.895 |
9.065 |
9.15 |
9.201 |
9.201 |
8.556 |
9.552 |
9.269 |
9.439 |
9.8406 |
CER |
0.09749 |
0.09787 |
0.09661 |
0.0971 |
0.09771 |
0.09809 |
0.09836 |
0.09971 |
0.096 |
0.09674 |
0.09757 |
0.1 |
0.107239 |
MOS |
0.271 |
0.351 |
0.379 |
0.168 |
0.282 |
0.351 |
0.404 |
0.461 |
0.207 |
0.296 |
0.391 |
0.459 |
0.2981 |
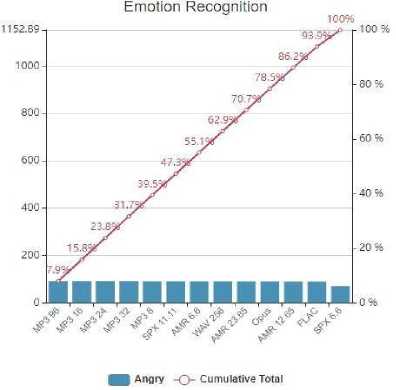
Fig. 3. Emotion Recognition cumulative curve for angry emotion class”
It is observed that FLAC has minor reduction of 86.14% The noted codec’s and direct contenders MP3 and WMA involve parallel compression ratios with a little favorable position for MP3: 13.81% (16kbits) to 86.18% (160kbit/s or more) for MP3 and 13.30% (24kbps) to 95.44% (160kbps) for wma. Higher bitrates > 256kbps has are larger thanthe orginal file which is three times more space occupied. figure 5 show some compressed codec’s.
Emotion Recognition
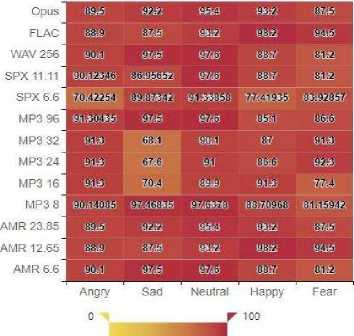
Fig. 4. Means of each bitrate for emotion classes of Telugu database
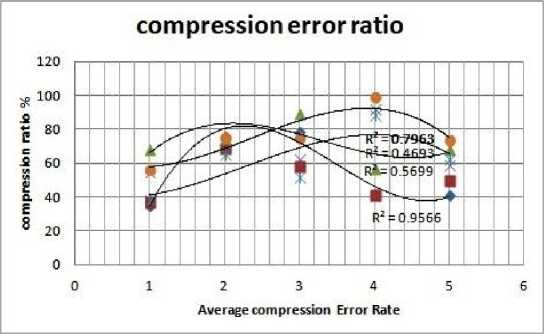
Fig. 5. Average compression error ratios of different codec and bitrate
-
B. Human labeling results
In View, the positioning of the distinctive codec’s and bit -rates it tends to be seen, that codec’s with a large filesize decrease bring about a lower UAR and better quality deviation. The UAR increases continuously with expanding bit-rates inside various codecs, aside from MP3 with a bitrate of 96 kbps. Figure 4 represents the effect of human labeling determined for each one, examined bit- rate for all possible emotions. These emotions can be distinguished with UAR of 75% level index in any event. Just in the highlighted cases a normal review of over 80% couldn’t be accomplished. In the two cases these outcomes were acquired by the most minimal bitrate of two distinctive codecs (SPX and AMR-WB). For these codec setups the UAR indicate what can most minimal incentive for specific feelings, contrasted with the remaining codec setups. figure 3 shows The incentive at the lower quarter means that the lower quartile s, the incentive at the middle offers a center and the incentive at the upper quarty is the upper quartile, unless the entire repeated bent into the quarters is is isolated. The lower quartile is ( n + 1) / 4 t h gain (n is the total recurrence, for example 289 for this situation) and the upper quartile is the 3(n+1)/4(866 in this scenario) value. Henceforth speex 6.6 bitrate, Flac and AMR-WB high bitrate has better recognition accuracy and falls under upper quartile. The distinction between these two is the inter-quartile range(IQR). It should also to be noted form figure 4 that UAR mean for emotions Angry and Neutral are consistently achieved more-than 90.00% decision making during the labeling process.
C. Compression Error ratio
7. Conclusion
The CER (in dB) is determined by 3 for the distinctive researched codecs is delineated. No, deviations observed in Free Lossless Audio Codec. (FLAC). With high compression techniques it is observed there are bitter results. with high compression ratios MP3, AAC, opus, the error rate reaches to congestion level.We accept that a specific portion of the error can’t be diminished, due to the codec algorithms for presumed data. Furthermore our CER supports state of art. Opus, AMR-WB speex achieve high accuracy and performed well than mp3. Speex as a particular codec has a error obviously over the best codecs, yet at extremelylow bitrate.
The analysis introduced in this paper look at a few issue articulations. First of all, it is evaluated on the probability that a trend of an emotional grouping using appropriate con- sistency metrics is possible to visualize Perceptual Objective Listening Quality Assess- ment (POLQA) , MOS and CER. As spectral data greatly influence a classifier’s device efficiency, it was supposed to be better suited to the CER and to draw a direct relation to the coefficient of the spearman. The encoding codecs with speech were analyzed at varying bit rates. Our findings confirmed previous tests on the efficiency of codec compression. The cognitive content of accepting mental deterioration is: If compression of the informationis required, which codec accomplishes the best UAR for emotion acknowledgment. Which codec achieves the best UAR for emotional recognition in case if compression is required. In the analysis, the MP3 codec with 32kbps bit rates and higher is ideal for the fulfillment of each emotion with a generally good UAR for a small file-sized encoding (bit-rate over 24kbit/s). For a low bit rate, SPeex(SPX) with a bit rate of 6.6kb/s can be used. In case of heavy compression, the SPX is to be used. This codec setup demonstrates both a low corre- lation and also the greatest drop of approximately 3.28% of WAV file size. It is observed that human labeling has better recognition accuracy, with minor errors which are insignificant. The speech system is predicted, and able to strongly differentiate emotions for human auditory system. Remarkably there’s a reversal tendency with in findings of human marking with prescribed codec settings for files with smaller sizes for automated emotional identification. From an outlook, a good comprehension towards classification technique of the codec is required to fully analyze the results. In comparison to linear amplification, neither compression nor expansion consistently increase speech understanding or sound quality. Given the measurable impact of expansion on speech understanding, the in its current form shouldn't be applied to forecast speech understanding under such signal processing settings.
Acknowledgements
We thank all the volunteers’ who helped us in making the Telugu database. Presently the database is under review with the committee for endorsement and will be made available publicly.
Список литературы Estimating the Effects of Voice Quality and Speech Intelligibility of Audio Compression in Automatic Emotion Recognition
- A. Pramod Reddy and V. Vijayarajan, “Recognition of human emotion with spectral features using multi layer-perceptron,” Int. J. Knowledge-Based Intell. Eng. Syst., vol. 24, no. 3, 2020, doi: 10.3233/KES-200044.
- A. P. Reddy and V. Vijayarajan, “Audio compression with multi-algorithm fusion and its impact in speech emotion recognition,” Int. J. Speech Technol., pp. 1–9, 2020.
- E. Villchur, “Signal processing to improve speech intelligibility in perceptive deafness,” J. Acoust. Soc. Am., vol. 53, no. 6, pp. 1646–1657, 1973.
- K. Bengtsson, “Talandet som levd erfarenhet.: En studie av fyra barn med Downs syndrom.,” Estetisk-filosofiska fakulteten, 2006.
- L. Laaksonen, H. Pulakka, V. Myllylä, and P. Alku, “Development, evaluation and implementation of an artificial bandwidth extension method of telephone speech in mobile terminal,” IEEE Trans. Consum. Electron., vol. 55, no. 2, pp. 780–787, 2009, doi: 10.1109/TCE.2009.5174454.
- R. P. Lippmann, L. D. Braida, and N. I. Durlach, “Study of multichannel amplitude compression and linear amplification for persons with sensorineural hearing loss,” J. Acoust. Soc. Am., vol. 69, no. 2, pp. 524–534, 1981.
- I. V Nábělek, “Performance of hearing-impaired listeners under various types of amplitude compression,” J. Acoust. Soc. Am., vol. 74, no. 3, pp. 776–791, 1983.
- D. K. Bustamante and L. D. Braida, “Multiband compression limiting for hearing-impaired listeners,” J. Rehabil. Res. Dev., vol. 24, no. 4, pp. 149–160, 1987.
- H. Levitt, M. Bakke, J. Kates, A. Neuman, T. Schwander, and M. Weiss, “Signal processing for hearing impairment.,” Scand. Audiol. Suppl., vol. 38, pp. 7–19, 1993.
- G. Walker, D. Byrne, and H. Dillon, “Learning effects with a closed response set nonsense syllable test,” Aust. New Zeal. J. Audiol., vol. 4, no. 1, pp. 27–31, 1982.
- R. Plutchik, “A general psychoevolutionary theory of emotion,” in Theories of emotion, Elsevier, 1980, pp. 3–33.
- R. Plutchik, Emotion: A psychoevolutionary synthesis. Harpercollins College Division, 1980.
- J. Boyd, “Sony unleashes new Aibo robot dog,” IEEE Spectrum. IEEE, 2017.
- Y. Attabi and P. Dumouchel, “Anchor models for emotion recognition from speech,” IEEE Trans. Affect. Comput., vol. 4, no. 3, pp. 280–290, 2013, doi: 10.1109/T-AFFC.2013.17.
- M. F. Teng, “Emotional Development and Construction of Teacher Identity: Narrative Interactions about the Pre-Service Teachers’ Practicum Experiences.,” Aust. J. Teach. Educ., vol. 42, no. 11, pp. 117–134, 2017.
- R. Plutchik, “A psychoevolutionary theory of emotions.” Sage Publications, 1982.
- Y. Qian and A. Mita, “Acceleration-based damage indicators for building structures using neural network emulators,” Struct. Control Heal. Monit. Off. J. Int. Assoc. Struct. Control Monit. Eur. Assoc. Control Struct., vol. 15, no. 6, pp. 901–920, 2008.
- D. King, S. M. Ritchie, M. Sandhu, S. Henderson, and B. Boland, “Temporality of emotion: Antecedent and successive variants of frustration when learning chemistry,” Sci. Educ., vol. 101, no. 4, pp. 639–672, 2017.
- I. Varga, R. D. De Lacovo, and P. Usai, “Standardization of the AMR wideband speech codec in 3GPP and ITU-T,” IEEE Commun. Mag., vol. 44, no. 5, pp. 66–73, 2006.
- K. Pisanski et al., “Vocal indicators of body size in men and women: A meta-analysis,” Anim. Behav., vol. 95, pp. 89–99, 2014, doi: 10.1016/j.anbehav.2014.06.011.
- A. M. Kondoz, Digital speech: coding for low bit rate communication systems. John Wiley \& Sons, 2005.
- M. Agiwal, A. Roy, and N. Saxena, “Next generation 5G wireless networks: A comprehensive survey,” IEEE Commun. Surv. \& Tutorials, vol. 18, no. 3, pp. 1617–1655, 2016.
- A. Nishimura, “Data hiding in pitch delay data of the adaptive multi-rate narrow-band speech codec,” in 2009 fifth international conference on intelligent information hiding and multimedia signal processing, 2009, pp. 483–486.
- J. G. Beerends et al., “Perceptual objective listening quality assessment (POLQA), the third generation ITU-T standard for end-to-end speech quality measurement part I—Temporal alignment,” J. Audio Eng. Soc., vol. 61, no. 6, pp. 366–384, 2013.
- P. Coverdale, S. Moller, A. Raake, and A. Takahashi, “Multimedia quality assessment standards in ITU-T SG12,” IEEE Signal Process. Mag., vol. 28, no. 6, pp. 91–97, 2011.
- C. Spearman, “The proof and measurement of association between two things.,” 1961.
- C. Spearman, “The proof and measurement of association between two things,” Am. J. Psychol., vol. 100, no. 3/4, pp. 441–471, 1987.
- A. F. Lotz, I. Siegert, M. Maruschke, and A. Wendemuth, “Audio Compression And Its Impact On Emotion Recognition in Affective Computing,” Elektron. Sprachsignalverarbeitung 2017, pp. 1–8, 2017.