Improving the security of wireless communication channels for unmanned aerial vehicles by creating false information fields
Автор: Basan E.S., Proshkin N.A., Silin O.I.
Журнал: Siberian Aerospace Journal @vestnik-sibsau-en
Рубрика: Aviation and spacecraft engineering
Статья в выпуске: 4 vol.23, 2022 года.
Бесплатный доступ
To date, the problems associated with the safety of unmanned aerial vehicles (UAVs) are quite acute. As a rule, when it comes to commercial small-sized UAVs, wireless communication channels are used to con-trol them. Most often, communication is implemented at a frequency of 2.4 GHz using the Wi-Fi protocol. Such a UAV is quite easy to detect by analyzing the radio frequency range or the data link layer. An attack-er, however, may not even have specialized equipment and use open source software. The detected UAV becomes the target for attacks. If it is known that the UAV operates as a wireless access point, then all Wi-Fi-specific attacks become relevant for the UAV. In this study, it is proposed to use the technology of creat-ing false information fields as the first line of defense to increase the resistance of the UAV to attacks. This technology will allow to hide a legitimate UAV communication channel behind a lot of fake ones. The goal is to create fake access points with the characteristics of real ones and emulate data transmission over the channels on which these access points are deployed. In addition to the fact that the technology allows to hide a legitimate UAV communication channel, it will also allow to mislead the attacker. It is important to make the intruder think that not a single UAV is approaching him, but a group. If the intruder attempts to attack decoys, attacker will compromise himself and be able to be detected. Thus, you can use the UAV as a bait. As a result of the pilot study, channels were identified on which the creation of fake access points is most effective. Using small computing power and the necessary antenna, you can achieve high results. This article demonstrates the effectiveness of creating 9 fake access points. A comparison was also made with real wireless network traffic. We can say that the emulated activity is quite close to the real activity.
Wireless communication channels, access point, radio intelligence, security, vulnerabilities
Короткий адрес: https://sciup.org/148329659
IDR: 148329659 | DOI: 10.31772/2712-8970-2022-23-4-657-670
Текст научной статьи Improving the security of wireless communication channels for unmanned aerial vehicles by creating false information fields
Unmanned aerial vehicles (UAVs) today are becoming an increasingly popular solution for performing various tasks [1]. Moreover, such tasks are often critical [2]. At the same time, UAVs are very vulnerable to attacks, since they are physically unprotected [3]. The most commonly used attacks exploit communication channel vulnerabilities. The UAV can be hidden by providing some physical protection [4]. Many countries use UAVs for military purposes, therefore, potential enemy countries also actively monitor the presence of drones flying nearby. The detection of small-sized UAVs, in particular, is carried out using the radar and optical methods. Various methods with different approaches for detecting unmanned aerial vehicles have been proposed, for example, based on the analysis of audio information [5–7], video images using cameras [8–10], and radio frequency sensing [11; 12]. However, each of these approaches has its own advantages and limitations. Sound methods are not effective in noisy environments, have limited range, and cannot detect UAVs using noise reduction methods. Whereas camera-based approaches require good lighting conditions, high quality lenses and ultra-high resolution cameras to detect UAVs at long distances, which is certainly much more expensive and more difficult to implement. Radio frequency methods based on the use of active radar are vulnerable to radio frequency interference [13]. However, the use of deep learning methods has a great advantage in the detection and classification of UAVs using deep neural networks (DNNs), which are also known as multilayer perceptrons (MLPs). Newer deep learning architectures such as Concentric Neural Networks (CNNs) are being used in UAV detection. CNNs are used to detect UAVs using CCTV cameras [14] from surveillance images [15] and Doppler signatures [16].
At present, there is a large number of works devoted to the application of deep learning methods for the classification of radio frequency signals. Examples include solving problems of determining the spectrum [17], MIMO detection [18], channel estimation and signal detection [19], communication at the physical layer [20], interference detection [21], stealth suppression [22; 23], power control [24], signal spoofing detection [25], and transmitter-receiver scheduling [26]. Classification of RF signals can be used for various applications, such as radio capture [27], which can eventually be used in cognitive radio systems [28] subject to dynamic and non-deterministic interference [29]. Modulation classification using deep neural networks is considered in [30–33], where the goal is to classify a given signal according to a known modulation type. Various types of data sets have been used to train a deep neural network in order to classify the modulation.
There is, of course, opposition to radio monitoring, for example, the transmission of noise on this frequency or false data, but this does not prevent the detection of UAVs.
To solve the problem of physical insecurity of communication channels, noise is added to the communication channel. Such noise does not affect the quality of the transmitted information, but allows you to hide the flow of legitimate data.
В беспроводных сетях Wi-Fi можно достаточно просто применить подобный способ защиты In wireless Wi-Fi networks, you can simply apply a similar method of protection by implementing an attack. For example, a beacon attack simulates the presence of many neighboring access points, which should make it difficult for a client to access a legitimate access point. Moreover, this attack does not actually affect legitimate users. Let us consider Fig. 1. The legitimate access point has a MAC address of 50:FF:20:38:AA:A1. Let's carry out a beacon attack and try to find a legitimate access point. Fig. 1b shows that in this case, in addition to the legitimate access point, there are many other points without an identifier. At the same time, if you imitate the access point ID by assigning the ID of a UAV commercial company, you can mislead the enemy.
CH 3 ][ Elapsed: 6 s ][ 2029-07-12 18:56
BSSID PWR Beacons #Data, #/s CH MB ENC CIPHER AUTH ESSID
50:FF:2O:38:AA:A1 -75 37 005 270 WPA2 CCMP PSK asw518
52:FF:2O:48:AA:A1 -69 37 005 270 WPA2 CCMP PSK «length: 0>
B0:95:75:4F:8D:5B -71 28 005 270 WPA2 CCMP PSK asw518_EXT
14:CC:2O:85:D2:1E -79 12 207 405 WPA2 CCMP PSK Megalink_12908
BSSID STATION PWR Rate Lost Frames Probe
(not associated) 9E:B2:61:06:CC:BB -40 0-1 1310
48:2C:AO:DC:34:D1 -46 0 - le 15065
B2:95:74:4F:8D:5A -72 0 - le 01
B2:95:74:4F:8D:5B -72 0 - le 12
FC:F1:36:6F:79:B9 -1 0e- 0 01
50:FF:20:38:AA:Al
50:FF:20:38:AA:Al
14:CC:2O:85:D2:1E
BSSID |
STATION |
PWR |
Rate |
Lost |
Frames |
(not associated) |
26:AC:B2:0A:86:D5 |
-38 |
0 - 1 |
0 |
3 |
(not associated) |
82:B2:C2:89:1A:50 |
-41 |
0 - 1 |
0 |
11 |
(not associated) |
3A:90:89:39:E4:8C |
-42 |
0 - 1 |
ID |
16 |
(not associated) |
EA:56:91:61:DE:72 |
-43 |
0 - 1 |
0 |
5 |
(not associated) |
8A:26:46:65:AB:96 |
-43 |
0 - 1 |
0 |
12 |
(not associated) |
9A:C8:BA:58:B2:CC |
-49 |
0 - 1 |
6 |
11 |
(not associated) |
2E:F8:F4:62:D3:13 |
-54 |
0 - 1 |
0 |
1 |
(not associated) |
5A:BD:79:15:E5:98 |
-65 |
0 - 1 |
0 |
13 |
(not associated) |
5E:57:50:D2:DC:1B |
-69 |
0 - 1 |
0 |
2 |
(nnt accnriatprl) |
. 04:B4:29:B1:CB:41 |
-90 |
0 - 1 |
0 |
3 |
lsO:FF:20:3B:AA:Al |
| 60:D9:A0:E8:3A:4E |
-34 |
le- 1 |
0 |
39 |
б
Рис. 1. Анализ сетевой активности ( а ) в нормальных условиях ( б ) при атаке с использованием beacon-флуда
Fig. 1. Analysis of network activity ( a ) under normal conditions ( b ) during an attack using a beacon flood
The table provides information about the most popular quadcopters and the characteristics of access points.
This study proposes a way to hide the UAV by creating false information fields. The method was tested by analyzing the radio spectrum and comparing the created fake fields with real ones. The results showed the effectiveness of the developed software, which allows you to create false access points that can be detected by an intruder and allow you to hide the real transmission.
Characteristics of UAVs as Wi-Fi hotspots
UAV name |
SSID |
Password |
HUBSAN X4 STAR PRO |
Hubsan_h507a_ ***** |
12345678 |
XIRO XPLORER MINI |
XPLORER_Mini_0b5abe |
XIRO1234 |
MJX X601H |
MJX H *** |
embedded in the application |
Parrot BEBOP |
BebopDrone-BO56122 |
embedded in the application |
XK Innovations X300-W |
XK innovat |
embedded in the application |
Module for creating false information fields around the UAV
The wireless data transmission medium is a priori unsafe, since it is practically impossible to protect it physically. Often the radio silence mode is used to hide the UAV. In this mode, the possibility of transmitting information to the UAV is not provided. At the same time, taking into account the current trends in the creation of a single cyberspace, when devices must not only collect and transmit data, but also exchange data with each other to coordinate their actions, operation in radio silence may be unacceptable [34]. Methods of imposing radio interference on the communication channel can also be used, which mask the legitimate transmission of information [35]. This method requires additional equipment and costs, so it is not always applicable [36].
The proposed software module ensures the concealment of a legitimate communication channel by creating multiple wireless access points. The study made it possible to determine the parameters of civilian UAVs for network communication and to model these parameters in order to hide a legitimate UAV [37]. Civil UAVs work as follows: the UAV network adapter switches to the access point creation mode after the operator has created an access point and, knowing the connection parameters (as a rule, this is the MAC address of the access point and its ID), can connect to it from an authorized device [38]. Thus, the task of the software module is to create several access points that would notify the civilian UAV about the parameters. The software module allows you to change the number of generated information fields. This approach will minimize the risks associated with the possibility of implementing attacks via UAV wireless communication channels [39]. Therefore, when information scanning the network, the enemy will see the picture shown in Fig. 2.
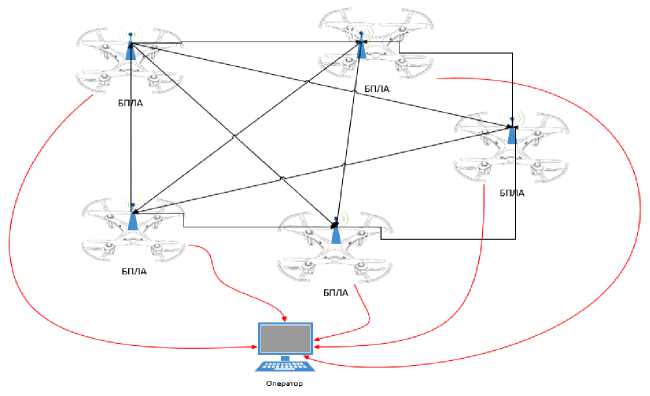
Рис. 2. Абстракция представления БПЛА для противника при радиоразведке
Fig 2. Abstraction of the representation of the UAV for the enemy in radio intelligence
Each access point operates on a separate radio channel, the IEEE 802.11 standard is implemented in such a way that broadcasting on a certain channel occurs constantly, therefore, analyzing the Wi-Fi frequency with the module turned on will show the activity of several devices (UAVs), as shown in Fig. 2 [40]. Thus, false information fields are created around the UAV. These information fields serve several purposes. Firstly, the software module allows you to hide the real UAV from the enemy, who uses the radio reconnaissance method. In addition, the module allows you to mislead the enemy by presenting false information that not one, but a group of UAVs is approaching the enemy. Secondly, the software module allows you to create a “bait” for the enemy so that he tries to gain access to a fake UAV, thereby impersonating himself. The software module is implemented for the Raspberry Pi 3
model B single board computer and requires the use of an external Wi-Fi adapter to create fake wireless access points. The emulation module consists of a Raspberry Pi 3 Model B microcomputer, microcomputer battery for offline operation, Linux Raspbian OS for Raspberry Pi, ZyXEL G-202 EE wireless USB Wi-Fi adapter, and implemented software. The developed software module runs a script to create a set of fake Wi-Fi access points automatically when an event occurs [41]. The airbase-ng utility [42] in Linux OS is used to create fake access points [43]. The developed software module consists of three subsystems:
-
1) interface initialization subsystem is necessary for the correct determination of the network interface on which false information fields will be created;
-
2) subsystem for generating false information fields serves to transfer the desired interface to the monitor mode and create fake access points on it;
-
3) event response subsystem acts as a connecting shell between the two previous subsystems, performs the functions of receiving and transmitting data from one subsystem to another, and also automatically turns on when necessary.
Analysis of the frequency spectrum emitted by the module to create false information fields
The experiments were carried out using a GW In-stek spectrum analyzer (GSP827) under conditions of weak exposure to radiating antennas. The laboratory stand is shown in Fig. 3 [44]. The 2.4 GHz wireless standard only allows 14 channels with a channel width of 20-22 MHz. Channels 1, 6, 11 are optimal for simultaneous use; 2, 7, 12; 3, 8, 13 or 4, 9, 14. But in this study there is no need to transmit useful data. Active radio traffic is generated by additional emulation flags set in the program. The 5 GHz standard has 140 frequency-separated channels, so you can deploy 10 times more APs, but the radiation radius is halved. In this study, an experiment with a frequency of 2.4 GHz with a radiation radius of up to 150 m with a transmitter power of 18 dB was carried out in an open area. The maximum number of 14 channels at 2.4 GHz does not mean that only 14 access points can be deployed. Two or more access points operating on the same channel simply overlap each other and transmit traffic in turn. This is shown in Fig. 4 [3].
Figure 5 shows a comparison of 2.4 and 5 GHz Wi-Fi communication ranges in open areas.
In a legitimate implementation of multiple access points, of course, loss of communication efficiency is possible, which is not important. In the RF method of emulating several UAVs, on the contrary, active radio traffic on the radar is welcomed, which is similar to an active TCP connection and packet transmission.
To begin with, let's analyze the frequency spectrum of a working phone as an access point, but without connecting any devices to it, the access point sends Beacon packets (beacon packets) with a certain period, but there is no radio traffic. Let us note the general explanations of the terms in Fig. 6 for each spectrum of signals on the example of the spectrum of two access points operating on different channels with a spectrum width of 75 MHz
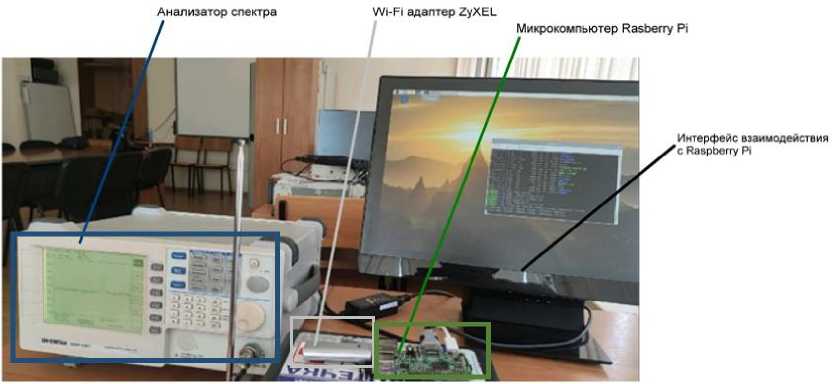
Рис. 3. Экспериментальный стенд для анализа спектра
Fig. 3. Experimental stand for spectrum analysis
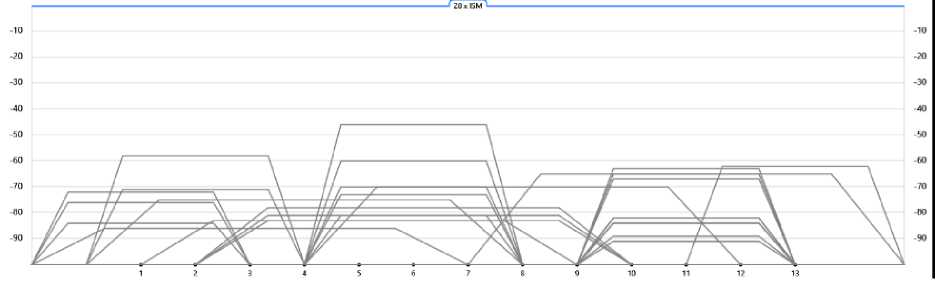
Рис. 4. Эффект наложения друг на друга точек доступа
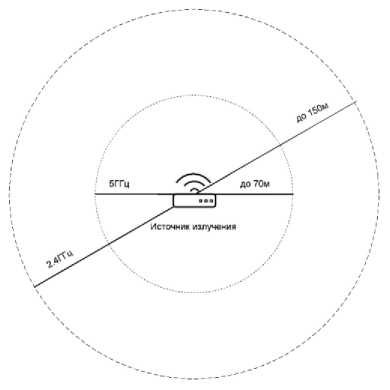
Рис. 5. Дальность связи Wi-Fi Рис. 6. Пояснение к терминам, использованным при анализе
Fig. 4. The effect of overlapping access points
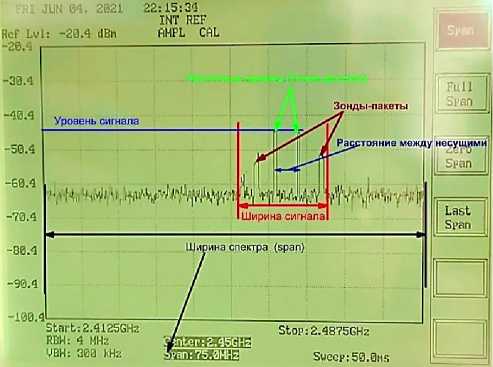
Fig. 5. Wifi range Fig. 6. Explanation of the terms used in the analysis
Bandwidth is 400 MHz, center carrier is 2.4 GHz, spectrum start is 2.2 GHz. The maximum signal level reaches approximately -30 dB at a frequency of approximately 2.46 GHz, which corresponds to channel 11 in the Wi-Fi standard. The frequency of occurrence of carriers is approximately 2 times per second. The difference between sending beacon packets and an active TCP connection on the spec- trum analyzer is that the signal width and carrier frequency have increased, the signal has become continuous, this indicates that some device is communicating with another device over the wireless network at a particular moment. When it is clear what the signal of a simple access point and an access point with a device connected to it and active packet exchange looks like, let's move on to the analysis of fake access points, how the number and division by channels affect the type of signal, the frequency of occurrence peaks and the width of the radio traffic as a whole.
With the increase in the number of access points, we should observe a picture such as in Fig. 7: each individual channel, i.e. a separate access point, should look like one peak in the spectrum (one carrier) (Fig. 7, a), but due to the imperfection of the antenna and the physical properties of the electromagnetic wave, you can see the combination of these peaks (Fig. 7b).
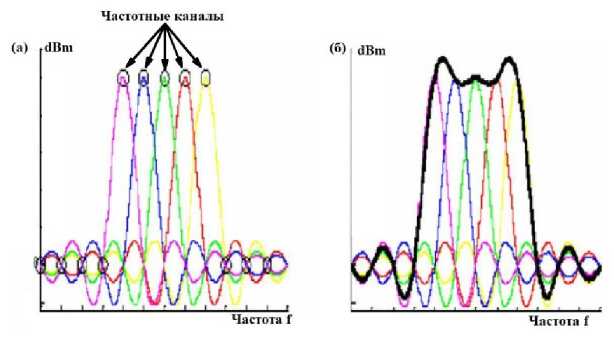
а
б
Рис. 7. Спектр множества точек доступа: а – совершенные несущие; б – огибающая несущих
Fig. 7. Spectrum of multiple access points: a – perfect carriers; b – envelope of carriers
In the next experiment, 25 access points were created, their number was gradually increased without disabling the previous ones. The signal is completely similar to the signal in Fig. 6, but with a lower level of -50 dBm. Due to the lower transmitter power, channel 12 was set, which corresponds to the middle frequency of the signal. Fig. 8 shows the signal spectrum of AP 1, but with active emulation flags enabled. The peak frequency increased by about 2 times, and the signal level slightly increased by 0.2–0.4 dB. There is another carrier created by the option to send additional probe packets for known devices. Fig. 8 shows the spectra of signals from a different number of false access points. The number of carriers increases, the distance between them decreases, and traffic becomes more active due to the increase in the number of access points.
It can be concluded that the increase in emulation efficiency becomes smaller after nine access points. However, when the number of access points increased to twenty-five, the average carrier frequency of the signal shifted to a frequency of 2.46 GHz and the signal width became about 25 MH
To determine the range of the effective number of successively connected APs the spectra of the signals of the 5th, 3rd, 2nd, and 1st access points on a spectrum width of 75 MHz are shown in Figures 9 and 10.
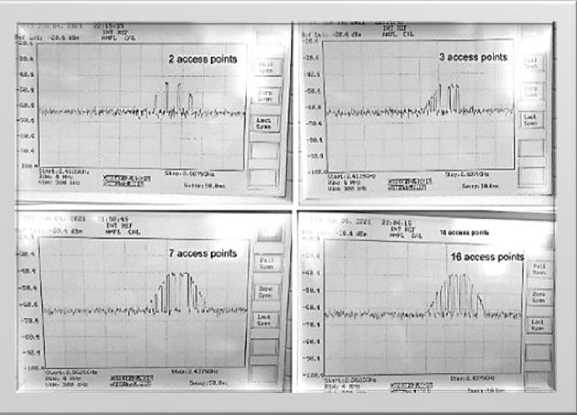
Рис. 8. Сигналы разного количества точек доступа
Fig. 8. Signals of a different number of access points
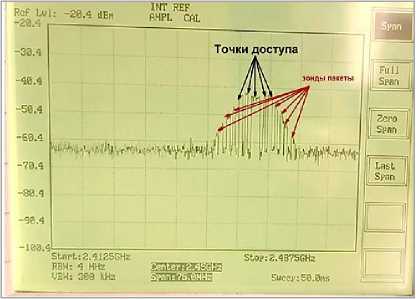
а
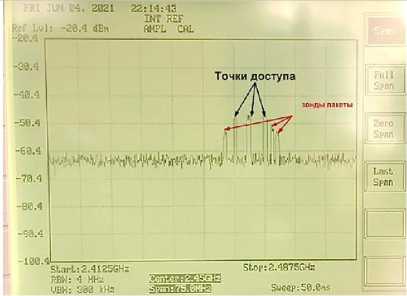
б
Рис. 9. Сравнение сигналов 5-и и 3-х точек доступа. Ширина спектра 75 МГц: а – 5 точек доступа; б – 3 точки доступа
Fig. 9. Comparison of signals of 5 and 3 APs. Spectrum width 75 MHz: a – 5 access points; b – 3 access points
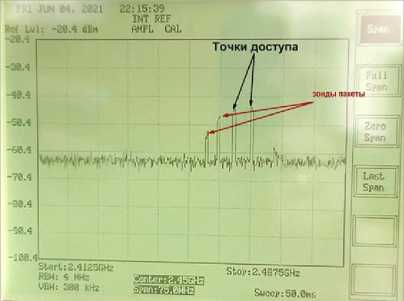
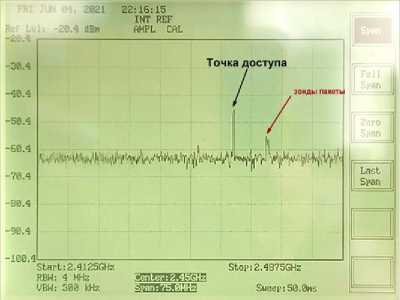
б
Рис. 10. Сравнение сигналов 2-х и 1-й точек доступа. Ширина спектра 75 МГц: а – 2 точки доступа; б – 1 точка доступа
Fig. 10. Comparison of signals of the 2nd and 1st AP. Spectrum width 75 MHz: a – 2 APs; b – 1 AP
After analyzing Fig. 8-10, it is clear that with an increase in the number of access points, the distance between the carriers decreases, the frequency of peaks increases, the signal width increases to the number of access points equal to 7. Fig. 11 shows the dependencies of the parameters: signal level, number of carriers and frequency of occurrence of carriers on the number of access points. The ZyXEL Wi-Fi adapter can only broadcast 14 channels, each 20-22 MHz wide. The software is used to set channel numbers 1, 6, 11, 16, 21, 26, 31, 36, 41, 46, etc. Therefore, a channel with a number greater than 14 will have a number calculated by formula (1):
Nk = NPk mod 14 , (1)
where Nk is a current channel number; NPk is a programmable channel number; mod is the integer remainder of the division.
Signal strength Number of carriers Frequency of occurrence of signals

а б в
Рис. 11. Зависимость уровня сигнала от количества точек доступа ( а ), количества несущих от количества точек доступа ( б ), частоты появления несущих от количества точек доступа ( в )
Fig. 11. Dependence of ( a ) signal level on the number of access points ( b ) the number of carriers on the number of access points ( c ) the frequency of occurrence of carriers on the number of access points
Fig. 12 shows that the actual channels overlap each other, especially noisy channels are 2, 7, 12.
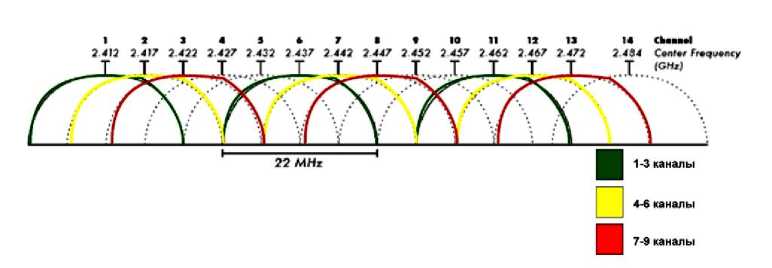
Рис. 12. Перекрытие каналов поддельных точек доступа
-
Fig. 12. Overlapping fake AP channels
It follows that the created channels overlap each other. However, since the adapter cannot broadcast simultaneously on 14 channels, the broadcast occurs in turn, but the queue does not consist of real channels, but of channels that were specified by the program, packets are transmitted first by 1, then 6, then 11, 2, 7 channels, etc. Due to this organization of the queue for sending packets, wave interference is much less. Figure 13 shows a comparison of a real TCP connection at 2.4 GHz and 5 dummy access points. The shape of the signal parameters are similar to each other, which will give an erroneous idea of the reconnaissance object.
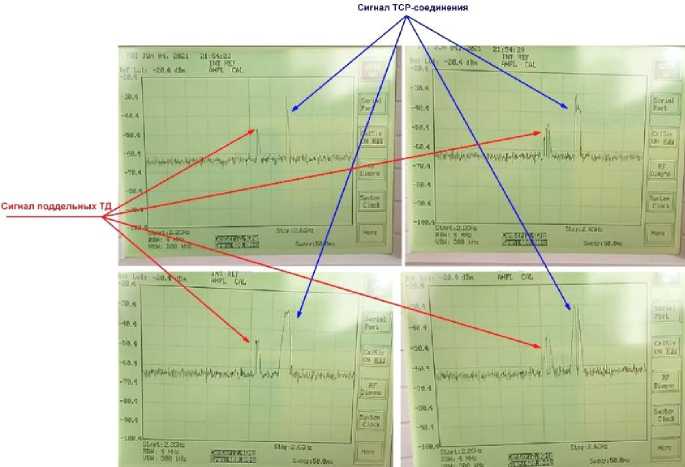
Рис. 13. Сравнение сигналов пяти точек доступа и TCP-соединения
-
Fig. 13. Comparison of the signals of five access points and a TCP connection
After activating the module, 14 fake Wi-Fi access points are created within a few seconds. This can be observed using any device with Wi-Fi (Fig. 13).
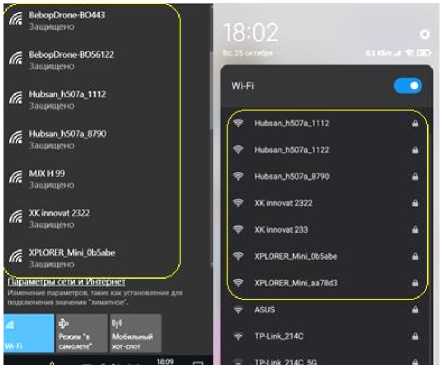
Рис. 14. Демонстрация работы модуля
-
Fig. 14. Demonstration of the module
Figure 14 shows that the Wi-Fi of the phone (Figure 14, on the right) and the computer adapter (Figure 14, on the left) alone see fake access points, and they are on the list before the legitimate ones.
Conclusion
This study demonstrates the operation of an RF emulation module for multiple UAV signals by creating fake access points that transmit traffic consisting only of beacon and probe packets. An analysis of the spectra of an increasing number of access points showed that with an increase in the number of access points, the number of carrier frequencies changes, which shows several working separate Wi-Fi devices, the frequency of peaks increases - the likelihood of adversary confusion increases - imitation of active radio traffic, while 9 simultaneously operating access points do not affect the efficiency in any way. An analysis of the spectrum of the actual operation of the device showed that when 13 access points are turned on simultaneously, the signal becomes more continuous and efficient compared to 9 access points. When 13 access points are created and launched at the same time, each of them broadcasts at an equal time interval from the neighboring one, then the effect of continuous radio traffic is achieved. Within the framework of studies, the following tasks were solved:
-
- an analysis of the statistics of UAV information fields was carried out;
-
- key characteristics of information fields are defined;
-
- the correspondence of the characteristics of false information fields to the characteristics of real UAV access points is substantiated;
-
- the process of creating legitimate information fields has been implemented.
In conclusion, it should be noted that the experiment was carried out with the simplest Wi-Fi adapter and a weak antenna. In real conditions, a much more powerful emitter should be used to increase the range and signal level, and the enemy will use a more sensitive antenna.
Acknowledgements . The work was financially supported by the Council for Grants of the President of the Russian Federation at the expense of the scholarship of the President of the Russian Federation for young scientists and graduate students (Competition SP-2022) No. SP-858.2022.5 on the topic “Technology for ensuring cybersecurity of automated systems from active information attacks based on the principle of reflection”.
Список литературы Improving the security of wireless communication channels for unmanned aerial vehicles by creating false information fields
- Xu C., Liao X., Tan J., Ye H., Lu H. Recent Research Progress of Unmanned Aerial Vehicle Regulation Policies and Technologies in Urban Low Altitude. IEEE Access. 2020, Vol. 8. P. 74175–74194. Doi: 10.1109/ACCESS.2020.2987622.
- Shi Y., Bai M., Li Y. Study on UAV Remote Sensing Technology in Irrigation District Infor-mationization Construction and Application. 10th International Conference on Measuring Technology and Mechatronics Automation (ICMTMA). Changsha, China, 2018, P. 252–255. Doi: 10.1109/ICMTMA.2018.00067.
- Gao X., Jia H., Chen Z., Yuan G., Yang S. UAV security situation awareness method based on semantic analysis. 2020 IEEE International Conference on Power, Intelligent Computing and Systems (ICPICS). Shenyang, China, 2020, P. 272–276. Doi: 10.1109/ICPICS50287.2020.9201954.
- Basan E., Medvedev M., Teterevyatnikov S. Analysis of the Impact of Denial-of-Service At-tacks on the Group of Robots. International Conference on Cyber-Enabled Distributed Computing and Knowledge Discovery (CyberC), China, 2018, P. 63–68. Doi: 10.1109/CyberC.2018.00023.
- Bernardini A., Mangiatordi F., Pallotti E., Capodiferro L. Drone detection by acoustic signature identification. IS TInt. Symp. Electron. Imaging Sci. Technol. 2017, P. 60–64.
- Kim J., Park C., Ahn J., Ko Y., Park J., Gallagher J. C. Real-time UAV sound detection and analysis system. IEEE Sensor Application Symposium (SAS). United States, 2017, P. 1–5.
- Nijim M., Mantrawadi N. Drone classification and identification system by phenome analysis using data mining techniques. IEEE Symposium on Technologies for Homeland Security. Waltham, MA, United States, 2016, P. 1–5.
- Aker C., Kalkan S. Using deep networks for drone detection. IEEE International Conference on Advanced Video and Signal Based Surveillance (AVSS). Lecce, Italy, 2017, P. 1–6. Doi: 10.1109/AVSS.2017.8078539.
- Saqib M., Daud Khan S., Sharma N., Blumenstein M. A study on detecting drones using deep convolutional neural networks. 14th IEEE International Conference on Advanced Video and Signal Based Surveillance (AVSS). Lecce, Italy, 2017, P. 1–5. Doi: 10.1109/AVSS.2017.8078541.
- Nguyen P., Ravindranathan M., Nguyen A., Han R., Vu T. Investigating cost-effective RF-based detection of drones. 2nd Workshop on Micro Aerial Vehicle Networks, Systems, and Applica-tions for Civilian Use (DroNet '16). Association for Computing Machinery. New York, NY, USA, 2016, P. 17–22. Doi: https://doi.org/10.1145/2935620.2935632.
- Ezuma M., Erden F., Anjinappa C. K., Ozdemir O., Guvenc I. Micro-UAV Detection and Clas-sification from RF Fingerprints Using Machine Learning Techniques. IEEE Aerospace Conference. Big Sky, MT, USA, 2019, P. 1–13. Doi: 10.1109/AERO.2019.8741970.
- Abeywickrama S., Jayasinghe L., Fu H., Nissanka S., Yuen C. RF-based Direction Finding of UAVs Using DNN. IEEE International Conference on Communication Systems (ICCS). Chengdu, China, 2019, P. 157–161. Doi: 10.1109/ICCS.2018.8689177.
- Fonseca R., Creixell W. Tracking and following a moving object with a quadcopter. 14th IEEE International Conference on Advanced Video and Signal Based Surveillance (AVSS). Lecce, Italy, 2017, P. 1–6. Doi: 10.1109/AVSS.2017.8078463.
- Kim B. K., Kang H. S., Park S. O. Drone classification using convolutional neural networks with merged doppler images. IEEE Geoscience and Remote Sensing Letters. 2017, Vol. 14, No. 1, P. 38–42.
- Davaslioglu K., Sagduyu Y. E. Generative adversarial learning for spectrum sensing. IEEE In-ternational Conference on Communications (ICC). Kansas City, MO, USA, 2018, P. 1–6. Doi: 10.1109/ICC.2018.8422223.
- He H., Wen C.-K., Jin S., Li G. Y. A model-driven deep learning network for MIMO detection. IEEE Transactions on Signal Processing. 2018, Vol. 68, P. 1702–1715.
- Ye H., Li G. Y., Juang B.-H. Power of deep learning for channel estimation and signal detec-tion in OFDM systems. IEEE Wireless Communications Letters. 2018, Vol. 7, No. 1, P. 114–117. Doi: 10.1109/LWC.2017.2757490.
- O’Shea T. J., Hoydis J. An introduction to deep learning for the physical layer // IEEE Transac-tions on Cognitive Communications and Networking (TCCN). 2017. Vol. 3, No. 4. P. 563–575. Doi: 10.1109/TCCN.2017.2758370.
- Shi Y., Sagduyu Y. E., Erpek T., Davaslioglu K., Lu Z., Li J. Adversarial deep learning for cognitive radio security: jamming attack and defense strategies. IEEE ICC 2018 Workshop – Promises and Challenges of Machine Learning in Comm. Networks. 2018, P. 1–6. Doi: 10.1109/ICCW.2018.8403655.
- Shi Y., Erpek T., Sagduyu Y. E., Li J. Spectrum data poisoning with adversarial deep learning. IEEE Military Communications Conference. Los Angeles, CA, USA, 2018, P. 407–412. Doi: 10.1109/MILCOM.2018.8599832.
- Sagduyu Y. E., Shi Y., Erpek T. IoT network security from the perspective of adversarial deep learning. IEEE International Conference on Sensing, Communication and Networking (SECON) Workshop on Machine Learning for Communication and Networking in IoT. Boston, MA, USA, 2019, P. 1–9. Doi: 10.1109/SAHCN.2019.8824956.
- Erpek T., Sagduyu Y. E., Shi Y. Deep learning for launching and mitigating wireless jamming attacks. IEEE Transactions on Cognitive Communications and Networking. 2019, Vol. 5, No. 1, P. 2–14. Doi: 10.1109/TCCN.2018.2884910.
- Shi Y., Davaslioglu K., Sagduyu Y. E. Generative adversarial network for wireless signal spoofing. ACM Conference on Security and Privacy in Wireless and Mobile Networks (WiSec) Work-shop on Wireless Security and Machine Learning (WiseML). Miami FL USA, 2019, P. 55–60. Doi: https://doi.org/10.1145/3324921.3329695.
- Abu Zainab N. et al. QoS and jamming-aware wireless networking using deep reinforcement learning. IEEE Military Communications Conference (MILCOM). Norfolk, VA, USA, 2019, P. 610–615. Doi: 10.1109/MILCOM47813.2019.9020985.
- Restuccia F. et al. DeepRadioID: Real-time channel-resilient optimization of deep learning-based radio fingerprinting algorithms. ACM Intl. Symposium on Mobile Ad Hoc Networking and Computing (MobiHoc). New York, NY, United States, 2019, P. 51–60. Doi: https://doi.org/10.1145/3323679.3326503.
- Soltani S. Distributed cognitive radio network architecture, SDR implementation and emulation testbed. IEEE Military Communications Conference (MILCOM). Tampa, FL, USA, 2015, P. 438–443. Doi: 10.1109/MILCOM.2015.7357482.
- Sagduyu Y. E., Berry R., Ephremides A. Jamming games in wireless networks with incomplete information. IEEE Communications Magazine. 2011, Vol. 49, No. 8, P. 112–118. Doi: 10.1109/MCOM.2011.5978424.
- O’Shea T., Corgan J., Clancy C. Convolutional radio modulation recognition networks // Communications in Computer and Information Science. 2016, Vol. 629. Springer, Cham. Doi: https://doi.org/10.1007/978-3-319-44188-7_16.
- O’Shea T. J., Roy T., Clancy T. C. Over-the-air deep learning-based radio signal classification. IEEE Journal of Selected Topics in Signal Processing. 2018, Vol. 12, No. 1, P. 168–179. Doi: 10.1109/JSTSP.2018.2797022.
- Ali A., Fan Y. Unsupervised feature learning and automatic modulation classification using deep learning model. Physical Communication. 2017, Vol. 25, P. 75–84.
- Shi Y. et al. Deep learning for signal classification in unknown and dynamic spectrum envi-ronments. IEEE International Symposium on Dynamic Spectrum Access Networks (DySPAN). New-ark, NJ, USA, 2019, P. 1–10. Doi: 10.1109/DySPAN.2019.8935684.
- Kiranyaz S., Zabihi M., Rad A. B., Tahir A., Ince T., Hamila R. Real-time PCG Anomaly De-tection by Adaptive 1D Convolutional Neural Networks. Signal Processing. 2016, P. 1–12. Doi: https://doi.org/10.48550/arXiv.1902.07238.
- Zheng Y., Liu Q., Chen E., Ge Y., Zhao J. L. Exploiting multi-channels deep convolutional neural networks for multivariate time series classification. Front. Comput. Sci. 2016, Vol. 10, No. 1, P. 96–112.
- Mikhalevich I. F., Trapeznikov V. A. Critical Infrastructure Security: Alignment of Views. In Systems of Signals Generating and Processing in the Field of on Board Communications. Moscow Technical University of Сommunications and Informatics, Russia, 2019, P. 1–5. Doi: 10.1109/SOSG.2019.8706821.
- Ilioudis C. V., Clemente C., Soraghan J. Understanding the potential of Self-Protection Jam-ming on board of miniature UAVs. In International Radar Conference (RADAR). Toulon, France, 2019, P. 1–6. Doi: 10.1109/RADAR41533.2019.171405.
- Li X., Ju R., Wang H., Sun Y. The Design and Research of Data Transmission on Remote Ra-dio Control in Different Noise Channel. 13th World Congress on Intelligent Control and Automation (WCICA). Changsha, China, 2018, P. 1306–1311. Doi: 10.1109/WCICA.2018.8630340.
- Proshkin N., Basan E., Lapina M. Radio Frequency Method for Emulating Multiple UAVs. 17th International Conference on Intelligent Environments (IE). Dubai, United Arab Republic, 2021, P. 1–4. Doi: 10.1109/IE51775.2021.9486599.
- Basan E., Basan A., Nekrasov A., Fidge C., Sushkin N., Peskova O. GPS-Spoofing Attack De-tection Technology for UAVs Based on Kullback–Leibler Divergence. Drones. 2022, No. 6(1), P. 8. Doi: https://doi.org/10.3390/drones6010008.
- Astaburuaga I., Lombardi A., La Torre B., Hughes C., Sengupta S. Vulnerability Analysis of AR. Drone 2.0, an Embedded Linux System. IEEE 9th Annual Computing and Communication Work-shop and Conference (CCWC). United States, 2019, P. 0666–0672. Doi: 10.1109/CCWC. 2019.8666464.
- Caballé M. C., Augé A. C., Lopez-Aguilera E., Garcia-Villegas E., Demirkol I., Aspas J. P. An Alternative to IEEE 802.11ba: Wake-Up Radio with Legacy IEEE 802.11 Transmitters. IEEE Access. 2019, Vol. 7, P. 48068–48086. Doi: 10.1109/ACCESS.2019.2909847.
- Madruga S., Tavares A., Brito A., Nascimento T. A Project of an Embedded Control System for Autonomous Quadrotor UAVs. Latin American Robotic Symposium, Brazilian Symposium on Robotics (SBR) and 2018 Workshop on Robotics in Education (WRE). João Pessoa, Brazil, 2018, P. 483–489. Doi: 10.1109/LARS/SBR/WRE.2018.00091.
- Carranza A., Mayorga D., DeCusatis C. Comparison of Wireless Network Penetration Testing Tools on Desktops and Raspberry Pi Platforms. 16th LACCEI International Multi-Conference for En-gineering, Education and Technology. Boca Raton, Florida, USA, 2018, P. 1–5.
- Abril-García J. H. et al. Application to monitoring a USB control with Python in Windows, Mac OS and Raspbian OS. ECORFAN Journal Democratic Republic of Congo. 2019, Vol. 5 (8), P. 1–6.
- Korneev S. Digital spectrum analyzer GSP-7830 manufactured by GW Instek. Components and technologies. 2008, Vol. 1 (78), P. 158–162.