International Journal of Intelligent Systems and Applications @ijisa
Статьи журнала - International Journal of Intelligent Systems and Applications
Все статьи: 1173
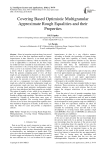
Covering Based Optimistic Multigranular Approximate Rough Equalities and their Properties
Статья научная
Since its inception rough set theory has proved itself to be one of the most important models to capture impreciseness in data. However, it was based upon the notion of equivalence relations, which are relatively rare as far as applicability is concerned. So, the basic rough set model has been extended in many directions. One of these extensions is the covering based rough set notion, where a cover is an extension of the concept of partition; a notion which is equivalent to equivalence relation. From the granular computing point of view, all these rough sets are unigranular in character; i.e. they consider only a singular granular structure on the universe. So, there arose the necessity to define multigranular rough sets and as a consequence two types of multigranular rough sets, called the optimistic multigranular rough sets and pessimistic rough sets have been introduced. Four types of covering based optimistic multigranular rough sets have been introduced and their properties are studied. The notion of equality of sets, which is too stringent for real life applications, was extended by Novotny and Pawlak to define rough equalities. This notion was further extended by Tripathy to define three more types of approximate equalities. The covering based optimistic versions of two of these four approximate equalities have been studied by Nagaraju et al recently. In this article, we study the other two cases and provide a comparative analysis.
Бесплатно