Методы сетевого моделирования структуры семантической памяти: теоретический обзор
Автор: Пиастро Р.А., Бармин А.В.
Журнал: Общество: социология, психология, педагогика @society-spp
Рубрика: Психология
Статья в выпуске: 3, 2024 года.
Бесплатный доступ
Статья посвящена рассмотрению методов моделирования структуры семантической памяти человека. В работе проводится аналитический обзор существующих подходов к моделированию данной структуры, в результате чего устанавливается наиболее методологически оптимальный способ моделирования семантической памяти - основанный на построении и анализе семантических сетей. Авторами изучаются различные методы сетевого моделирования структуры семантической памяти: задача вербальной беглости, задача снежного кома, задача определения семантической связанности. Кроме того, приводятся примеры использования обозначенных методов в рамках психологических исследований, освещаются их методологические преимущества и недостатки. Результаты настоящей работы могут быть полезны при формировании новых методов сетевого моделирования структуры семантической памяти человека, а также при создании интеллектуальных систем, обрабатывающих графовые данные.
Семантическая память, сетевое моделирование, семантическая сеть, теория графов, дистрибутивная семантика, признаковая модель, задача вербальной беглости, парадигма снежного кома, задача семантической связанности
Короткий адрес: https://sciup.org/149145310
IDR: 149145310 | DOI: 10.24158/spp.2024.3.3
Текст научной статьи Методы сетевого моделирования структуры семантической памяти: теоретический обзор
Введение . Согласно классическому пониманию структуры долговременной памяти человека (Tulving, 1983), семантическая память представляет собой один из отделов долговременной декларативной памяти, отвечающий за хранение общих знаний о мире (например, у кошки два
уха; Париж – столица Франции). Другим отделом долговременной декларативной памяти является эпизодическая память, отвечающая за хранение информации, связанной с личным опытом человека, конкретными временем и местом (например, воспоминания о дне рождения, первом дне в университете и т. д.). Следует отметить, что, хотя различие между этими типами памяти неоднократно подтверждалось рядом экспериментов (Gabrieli et al., 1988; O’Kane et al., 2004), сегодня существует тенденция исключения строгого разграничения между ними, которая подкрепляется результатами различных научных работ (De Brigard et al., 2022).
В настоящее время приобретает актуальность проблема моделирования структуры семантической памяти человека. В первую очередь это связано с разработкой новых интеллектуальных систем, базирующихся на принципах организации семантической памяти и используемых в различных сферах жизни общества, например в обучении иностранному языку (Hasnine et al., 2019; Runge, Hovy, 2020), медицине1, исследованиях рекламы (Пиастро, 2023) и др. Применение таких систем в разных областях общественной жизни позволяет автоматизировать сложные рабочие процессы и значительно улучшить показатели.
В современной науке существует множество способов моделирования структуры семантической памяти, однако каждый из них обладает своими преимуществами и недостатками. Это создает проблему выбора наиболее методологически оптимального метода, позволяющего отразить структуру семантической памяти человека максимально релевантным способом. В связи с этим настоящая работа направлена на рассмотрение основных методов моделирования структуры семантической памяти и раскрытие методологически оптимального метода и его разновидностей.
Проблема моделирования структуры семантической памяти в психологии . Сегодня существует несколько подходов к моделированию структуры семантической памяти человека. Среди главных выделяют сетевой, дистрибутивный и подход, основанный на признаках.
Сетевое моделирование представляет собой метод построения и анализа сетевых моделей, отражающих организацию тех или иных изучаемых явлений в виде множества узлов и множества ребер, объединяющих некоторые пары этих узлов (Морозова, 2017). Традиционно рассмотрение сетевых моделей семантической памяти началось с исследований особенностей извлечения семантической информации из памяти человека. Так, впервые А.М. Коллинз и М.Р. Куиллиан провели эксперимент, заключающийся в проверке истинности утверждений при фиксировании времени реакций (Collins, Quillian, 1969) . Ученым удалось установить, что распределение показателей длительности времени реакции при проверке утверждений может свидетельствовать в пользу иерар-хичной сетевой структуры семантической памяти. Позднее принцип иерархичности был опровергнут в работе А.М. Коллинза и Э.Ф. Лофтус, показавших, что структура семантической памяти человека представляет собой сеть со взвешенными ребрами, обеспечивающими распространение активации между узлами в зависимости от актуально стоящей перед человеком задачи (теория распространения активации). В таком виде сетевая структура семантической памяти стала использоваться в дальнейших психологических и когнитивных исследованиях.
Дистрибутивное моделирование используется для объяснения возможных механизмов, лежащих в основе работы семантической памяти человека, и позволяет представить ее структуру в качестве набора статистических показателей распределения слов в текстовых корпусах (коэффициентов совместной встречаемости). В основе дистрибутивных моделей находится дистрибутивная гипотеза, согласно которой «все слова определяются их соседями» (Firth, 1957). В настоящее время разработаны различные классы дистрибутивных семантических моделей, базирующихся на разных типах обучения, например Error-Free Learning и Error-Driven Learning. Одной из самых известных дистрибутивных семантических моделей сегодня является модель GPT (англ. Generative Pre-training of Transformers – трансформер, обученный на генерацию текста).
Признаковые модели основываются на понимании структуры семантической памяти как набора понятий, связанных между собой за счет совпадения (перекрытия) признаков. Например, Э. Смит с коллегами выделяли определяющие (задающие объем категории) и характерные (относящиеся к содержанию понятий этих категорий) признаки понятий (Smith et al., 1974). Так, понятия могут совпадать по определяющим и характерным признакам (например, сова – это птица), только по определяющим признакам (например, гусь – это птица) и только по характерным признакам (например, стрекоза – это птица). На базе ответов испытуемых в специальных задачах, требующих перечисления признаков понятий, создаются банки признаков, которые используются для обучения нейросетей.
Перечисленные методы имеют как преимущества, так и недостатки. Наименее популярным из представленных подходов к моделированию структуры семантической памяти является подход, основанный на признаках. Хотя он успешно применяется в исследованиях семантической памяти человека, данный подход не отличается высокой мощностью и имеет ряд ограничений. Например, в рамках признаковых моделей такие понятия, как мед и пчела, не будут совпадать ни по определяющим, ни по характерным признакам, тогда как в рамках дистрибутивных (мед и пчела часто встречаются вместе в текстовых корпусах) и сетевых (мед и пчела часто ассоциируются друг с другом) моделей они будут связаны. Более эффективным подходом к моделированию структуры семантической памяти выступает дистрибутивный подход. Он обладает высокой вычислительной мощностью, однако не допускает анализа структуры семантической памяти человека на разных структурных уровнях и, будучи ограниченным текстовыми корпусами, не учитывает моторных, перцептивных и аффективных аспектов формирования семантических репрезентаций. В сравнении с представленными моделями сетевой подход выглядит наиболее методологически оптимальным, так как отличается высокой вычислительной мощностью, позволяет проводить анализ структуры семантической памяти на разных структурных уровнях и учитывает поведенческие факторы. Поскольку сетевой подход, возможно, является оптимальным методологическим подходом к моделированию структуры семантической памяти человека, далее рассмотрены его основные разновидности.
Методы сетевого моделирования структуры семантической памяти человека . В настоящее время выделяются три метода сетевого моделирования структуры семантической памяти человека: задача вербальной беглости, задача снежного кома и задача определения семантической связанности. Далее рассмотрен каждый из этих методов и приведены примеры его использования в психологических и когнитивных исследованиях.
В задаче вербальной беглости (Verbal Fluency Task) испытуемым предлагается назвать как можно больше представителей определенной категории (например, животные, мебель и т. д.) за фиксированный промежуток времени (как правило, 1 мин). Результаты выполнения задачи представляют собой сумму верно названных понятий – представителей обозначенной категории. Результаты могут сравниваться со стандартными показателями выполнения данной задачи. Существует множество исследований, где был применен этот метод сетевого моделирования структуры семантической памяти. Например, в нейропсихологическом исследовании Г. Чжана с соавторами с помощью задачи вербальной беглости была смоделирована сетевая структура семантической памяти у здоровых испытуемых и пациентов с болезнью Альцгеймера и Паркинсона и установлены их сетевые особенности (Zhang et al., 2022). С помощью сетевого анализа выявлены структурные различия в полученных сетях: между сетями трех групп по макроуровневым сетевым характеристикам, таким как средний кратчайший путь (средняя минимальная дистанция между двумя узлами в сети), плотность (отношение числа узлов к максимально возможному количеству узлов) и диаметр (средняя максимальная дистанция между двумя узлами в сети). Кроме этого, сети испытуемых с нейродегенеративными заболеваниями показали наличие петель (ребер, инцидентных одной и той же вершине), в отличие от семантических сетей здоровых испытуемых представляющих собой связанные нецикличные графы.
Задача вербальной беглости применяется не только в нейропсихологических исследованиях. Так, в работах М.П. Агустин-Ллах (Agustin-Llach, 2022) и С. Фэн, Ц. Лю (Feng, Liu, 2023) показано, как меняется сетевая структура СП, смоделированная с помощью задачи вербальной беглости, у изучающих иностранный язык на протяжении разных промежутков времени. Так, в исследовании М.П. Агустин-Ллах сравнивались семантические сети испытуемых, различающихся двумя годами изучения иностранного языка, а в исследовании С. Фэн и Ц. Лю – четырьмя годами. Результаты показали, что по мере изучения языка изменяются такие макроскопические сетевые характеристики, как средняя степень узла (Agustin-Llach, 2022; Feng, Liu, 2023), средний кратчайший путь (Feng, Liu, 2023), коэффициент кластеризации (вероятность, что соседи узлов в сети сами будут соседями) (Feng, Liu, 2023), диаметр (Agustin-Llach, 2022; Feng, Liu, 2023), соответствие структуре «малого мира» (особая сетевая структура, характеризующаяся небольшим показателем кратчайшего пути и высоким коэффициентом кластеризации) (Feng, Liu, 2023).
В задаче (парадигме) снежного кома (Snowball Sampling Paradigm) испытуемым предлагается придумать слова – ассоциации к заранее заготовленным экспериментатором словам (в оригинальной работе они назывались «семенами» (seeds), которые используются в качестве источников роста семантических сетей) (Morais et al., 2013). Во второй итерации испытуемые придумывают ассоциации к собственным словам-ассоциациям, данным к заранее заготовленным экспериментатором словам. В третьей итерации предлагается снова придумывать ассоциации к своим ассоциациям из предыдущей итерации. Задача подразумевает бесконечное количество итераций, по мере прохождения которых семантическая сеть разрастается, словно снежный ком.
Эта задача была разработана А.С. Мораис (Morais et al., 2013) для изучения сетевых характеристик индивидуальных крупномасштабных семантических сетей в целях проверки результатов исследования М. Стейверса и Дж.Б. Тененбаума (Steyvers, Tenenbaum, 2005), построивших семантические сети на основе психолингвистических баз данных, например MRC (Coltheart,
1981). Исследование М. Стейверса и Дж.Б. Тененбаума показало, что семантические сети, основанные на разных психолингвистических базах данных, соответствуют структуре «малого мира» и подчиняются степенному закону распределения (сеть, характеризующаяся небольшим количеством узлов с высокой степенью и большим числом степеней с невысокой степенью). Изучение индивидуальных семантических сетей, проведенное А.С. Мораис (Morais et al., 2013), привело к похожим результатам, но с тем отличием, что семантические сети, сгенерированные в результате выполнения задачи снежного кома, также выступают сетями со структурой «малого мира», но подчиняются степенному закону с экспоненциальным отсечением. Следует отметить, что, хотя задача снежного кома позволяет представлять структуру семантической памяти человека в виде крупномасштабной индивидуальной семантической сети, она является весьма трудоемкой. Например, в оригинальном исследовании длительность ее реализации составила около 6 недель почасовых сессий. По этой причине авторы стали разрабатывать модифицированные версии оригинальной задачи. Например, Д.У. Вульф и Р. Мата предложили модифицированную версию задачи снежного кома (Mini-Snowball), состоящую всего из двух итераций (в оригинальном исследовании использовано около шести) (Wulff, Mata, 2022). Сокращенная версия задачи успешно применялась учеными при анализе семантической репрезентации слова «риск».
Наконец, в задаче определения семантической связанности (Semantic Relatedness Task), разработанной Й.Н. Кенеттом с коллегами (Kenett et al., 2017), испытуемым предлагается оценивать семантическую связанность предъявляемых пар слов по шкале от «совсем не связаны» до «сильно связаны». Слова заранее отбираются экспериментаторами и комбинируются в уникальные пары. Выполнение оригинальной задачи занимает около 10 мин. По результатам формируется матрица смежности (в которой столбцы и строки являются узлами, а показатели на их пересечении принимают одно из двух значений: 0 – узлы не связаны, 1 – связаны). Например, в исследовании М. Бенедека с соавторами с помощью задачи семантической связанности была смоделирована сетевая структура семантической памяти у испытуемых, различающихся по уровню креативности (How semantic memory structure…, 2017). Результаты сетевого анализа показали, что креативность связана с более короткой длиной кратчайшего пути и более высоким уровнем кластеризации. Следует отметить, что самая первая версия задачи семантической связанности применялась в работе Й.Н. Кенетта с соавторами как средство оценки длины путей (количества ребер между узлами) между понятиями в семантических сетях (Kenett et al., 2017). Один из выводов этой работы заключается в том, что чем больше шагов требуется сделать от узла A до узла B в сети, тем меньше вероятность того, что испытуемые будут считать слова A и B связанными, и тем ниже будет показатель времени ответа при их оценке.
Примеры результатов выполнения каждой из представленных задач показаны на рисунке 1.
Рассмотренные методы сетевого моделирования структуры семантической памяти имеют определенные преимущества и недостатки. Например, задача вербальной беглости позволяет отразить структуру СП с минимальными временными затратами. Действительно, длительность ее реализации составляет около 1 мин, тогда как, например, оригинальная задача снежного кома занимает 6 недель почасовых сессий. Однако она не допускает построения крупномасштабных индивидуальных семантических сетей и ограничена заранее установленной экспериментатором семантической категорией. В отличие от задачи вербальной беглости задача определения семантической связанности позволяет строить крупномасштабные индивидуальные семантические сети, но она также ограничена заранее отобранным экспериментатором материалом –словами, из которых затем составляются уникальные пары. В отличие от двух предыдущих задач задача снежного кома дает возможность строить крупномасштабные индивидуальные семантические сети и в наименьшей степени ограничена заранее отобранным экспериментатором материалом (используются только слова-«семена»), однако данная задача требует больших временных затрат. Специфика трех оригинальных методов сетевого моделирования отражена в таблице 1.
Семантические сети как средство репрезентации структуры семантической памяти человека являются основой для создания сетевых интеллектуальных моделей – алгоритмов, обрабатывающих данные, представленных в виде семантических сетей (Liu, 2023; PREFER: using a graph-based approach…, 2012; Sun et al., 2020), и формирования сетевых баз данных – графов знаний (Chai et al., 2019; Grundspenkis, Strautmane, 2009; Willrich et al., 2020).
Следует отметить, что в настоящее время существует тенденция объединения различных подходов к моделированию семантической памяти. Например, Р. Спир с коллегами разработали процедуру комбинации ConceptNet – сети с помеченными ребрами с такими дистрибутивными моделями, как word2vec и GloVe (Speer et al., 2016). Мультиплексные сети могут комбинировать данные, полученные в ходе ассоциативных экспериментов, показатели встречаемости слов в текстовых корпусах и данные признаков понятий для создания многослойной структуры семантической памяти человека (Stella et al., 2017).
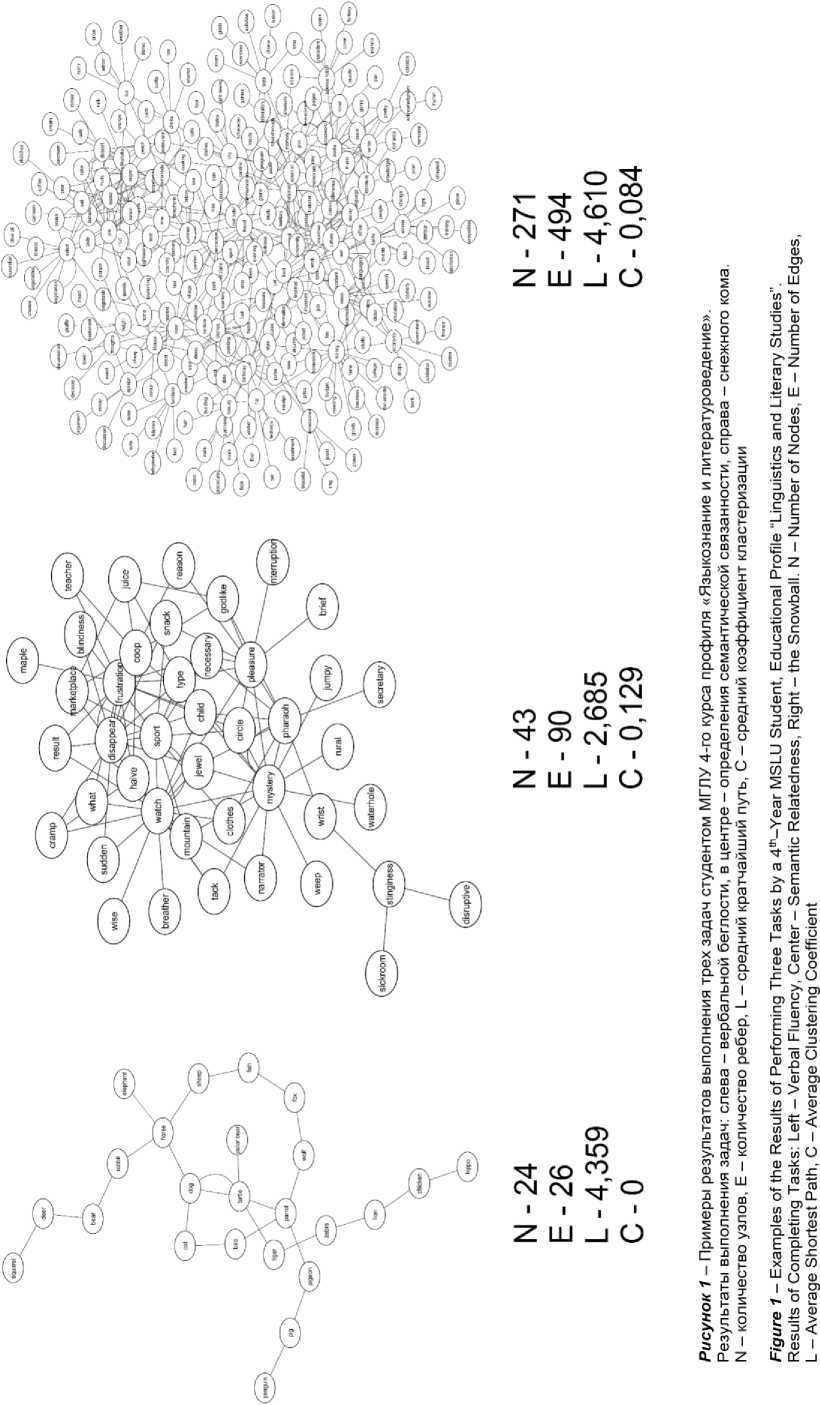
Таблица 1 – Особенности методов сетевого моделирования: возможность построения крупномасштабных семантических сетей, неограниченность выбора ассоциаций, трудоемкость реализации
Table 1 – Features of Existing Network Modeling Methods: The Possibility of Constructing Large-Scale Semantic Networks, Unlimited Choice of Associations, Complexity of Implementation
Метод сетевого моделирования |
Возможность построения крупномасштабных семантических сетей |
Неограниченность выбора ассоциаций |
Трудоемкость реализации |
Задача вербальной беглости |
– |
– |
– |
Задача определения семантической связанности |
+ |
– |
– |
Задача снежного кома |
+ |
+ |
+ |
Заключение . В первой части настоящего теоретического обзора рассмотрена проблема моделирования структуры семантической памяти в психологии. Проанализированы дистрибутивный, сетевой и основанный на признаках подходы. В результате теоретического анализа релевантных научных источников установлен методологически оптимальный способ моделирования структуры семантической памяти человека – сетевой.
Во второй части обзора рассмотрены существующие разновидности сетевого моделирования структуры семантической памяти человека. Проанализированы следующие методы: задача вербальной беглости, задача определения семантической связанности, задача снежного кома, обозначены возможности их применения в исследовании структуры семантической памяти человека.
Результаты представленного в настоящей статье анализа способов сетевого моделирования структуры семантической памяти человека показали свойственные им методологические преимущества и недостатки. Полученные результаты могут быть использованы при создании новых методов сетевого моделирования структуры семантической памяти человека, учитывающих достоинства и недостатки классических методов. Кроме этого, результаты могут быть задействованы при разработке интеллектуальных алгоритмов, основанных на принципах работы семантической памяти человека и при формировании баз данных по типу графов знаний.
Список литературы Методы сетевого моделирования структуры семантической памяти: теоретический обзор
- Морозова О.А. Структурное сетевое моделирование в когнитивной науке // Психологические исследования. 2017. Т. 10, № 55. https://doi.org/10.54359/ps.v10i55.351.
- Пиастро Р.А. Особенности репрезентации рекламы в структуре семантической памяти // Психология XXI в. – 2023: наука как свобода и творчество: сб. тез. участников XXVII Междунар. науч. конф. молодых ученых / отв. ред. А.В. Шаболтас. СПб., 2023. С. 282–284.
- Agustin-Llach M.P. How age and L2 proficiency affectthe L2 lexicon // System. 2022. Vol. 104. Article 102697. https://doi.org/10.1016/j.system.2021.102697.
- Chai A., Le J.P., Lee A.S., Lo S.M. Applying graph theory to examine the dynamics of student discussions in small-group learning // CBE – Life Sciences Education. 2019. Vol. 18, no. 2. https://doi.org/10.1187/cbe.18-11-0222.
- Collins A.M., Loftus E.F. A spreading-activation theory of semantic processing // Psychological Review. 1975. Vol. 82, no. 6. P. 407–428. https://doi.org/10.1037/0033-295X.82.6.407.
- Collins A.M., Quillian M.R. Retrieval time from semantic memory // Journal of verbal learning and verbal behavior. 1969. Vol. 8, no. 2. P. 240–247. https://doi.org/10.1016/S0022-5371(69)80069-1.
- Coltheart M. The MRC psycholinguistic database // The Quarterly Journal of Experimental Psychology. Section A. 1981. Vol. 33, no. 4. P. 497–505. https://doi.org/10.1080/14640748108400805.
- De Brigard F., Umanath S., Irish M. Rethinking the distinction between episodic and semantic memory: Insights from the past, present, and future // Memory & Cognition. 2022. Vol. 50, no. 3. P. 459–463. https://doi.org/10.3758/s13421-022-01299-x.
- Feng X., Liu J. The developmental trajectories of L2 lexical-semantic networks // Humanities and Social Sciences Commu-nications. 2023. Vol. 10, no. 1. https://doi.org/10.1057/s41599-023-01621-1.
- Firth J. A synopsis of linguistic theory, 1930–1955 // Studies in linguistic analysis. Oxford, 1957. P. 1–31.
- Gabrieli J.D., Cohen N.J., Corkin S. The impaired learning of semantic knowledge following bilateral medial temporal-lobe resection // Brain and Cognition. 1988. Vol. 7, no. 2. P. 157–177. https://doi.org/10.1016/0278-2626(88)90027-9.
- Grundspenkis J., Strautmane M. Usage of graph patterns for knowledge assessment based on concept maps // Computer Science. 2009. Vol. 38. P. 61–70. https://doi.org/10.2478/v10143-009-0005-y.
- Hasnine M.N., Flanagan B., Ishikawa M., Ogata H., Mouri K., Kaneko K. A platform for image recommendation in foreign word learning // Companion Proceedings of the 9th International Conference on Learning Analytics and Knowledge (LAK’19). Society for Learning Analytics Research (SoLAR). 2019. P. 187–188.
- How semantic memory structure and intelligence contribute to creative thought: A network science approach / M. Benedek, Y.N. Kenett, K. Umdasch, D Anaki., M. Faust, A.C. Neubauer // Thinking & Reasoning. 2017. Vol. 23, no. 2. P. 158–183. https://doi.org/10.1080/13546783.2016.1278034.
- Kenett Y.N., Levi E., Anaki D., Faust M. The semantic distance task: Quantifying semantic distance with semantic network path length // Journal of Experimental Psychology: Learning, Memory, and Cognition. 2017. Vol. 43, no. 9. P. 1470–1489. https://doi.org/10.1037/xlm0000391.
- Liu P. Design of a knowledge graph-based interactive English teaching platform for college students // Applied Mathematics and Nonlinear Sciences. 2023. https://doi.org/10.2478/amns.2023.2.01213.
- Morais A.S., Olsson H., Schooler L.J. Mapping the structure of semantic memory // Cognitive Science. 2013. Vol. 37, no. 1. P. 125–145. https://doi.org/10.1111/cogs.12013.
- O'Kane G., Kensinger E.A., Corkin S. Evidence for semantic learning in profound amnesia: An investigation with patient H.M. // Hippocampus. 2004. Vol. 14, no. 4. P. 417–425. https://doi.org/10.1002/hipo.20005.
- PREFER: using a graph-based approach to generate paraphrases for language learning / M.H. Chen, S.T. Huang, C.C. Huang, H.C. Liou, J.S. Chang // Proceedings of the Seventh Workshop on Building Educational Applications Using NLP. 2012. P. 80–85.
- Runge A., Hovy E. Exploring neural entity representations for semantic information // Proceedings of the Third BlackboxNLP Workshop on Analyzing and Interpreting Neural Networks for NLP. 2020. P. 204–216. https://doi.org/10.48550/arXiv.2011.08951.
- Smith E.E., Shoben E.J., Rips L.J. Structure and process in semantic memory: A featural model for semantic decisions // Psychological Review. 1974. Vol. 81, no. 3. P. 214–241. https://doi.org/10.1037/h0036351.
- Speer R., Chin J., Havasi C. Conceptnet 5.5: An open multilingual graph of general knowledge // Proceedings of the AAAI conference on artificial intelligence. 2017. Vol. 31, no. 1. P. 4444–4451. https://doi.org/10.1609/aaai.v31i1.11164.
- Stella M., Beckage N.M., Brede M. Multiplex lexical networks reveal patterns in early word acquisition in children // Scientific Reports. 2017. Vol. 7, no. 1. https://doi.org/10.1038/srep46730.
- Steyvers M., Tenenbaum J.B. The large‐scale structure of semantic networks: Statistical analyses and a model of semantic growth // Cognitive Science. 2005. Vol. 29, no. 1. P. 41–78. https://doi.org/10.1207/s15516709cog2901_3.
- Sun F., Yu M., Zhang X., Chang T.W. A vocabulary recommendation system based on knowledge graph for Chinese language learning // 2020 IEEE 20th International Conference on Advanced Learning Technologies (ICALT). 2020. P. 210–212. https://doi.org/10.1109/ICALT49669.2020.00068.
- Tulving E. Ecphoric processes in episodic memory // Philosophical Transactions of the Royal Society of London. Biological Sciences. 1983. Vol. 302, no. 1110. P. 361–371. https://doi.org/10.1098/rstb.1983.0060.
- Willrich R., Mittmann A., Fileto R., dos Santos A.L. Capture and visualisation of text understanding through semantic anno-tations and semantic networks for teaching and learning // Journal of information Science. 2020. Vol. 46, no 4. P. 528–543. https://doi.org/10.1177/01655515198495.
- Wulff D.U., Mata R. On the semantic representation of risk // Science Advances. 2022. Vol. 8, no. 27. https://doi.org/10.1126/sci-adv.abm1883.
- Zhang G., Ma J., Chan P., Ye Z. Graph theoretical analysis of semantic fluency in patients with Parkinson’s disease // Be-havioural Neurology. 2022. Article 6935263. https://doi.org/10.1155/2022/6935263.