International Journal of Image, Graphics and Signal Processing @ijigsp
Статьи журнала - International Journal of Image, Graphics and Signal Processing
Все статьи: 1110
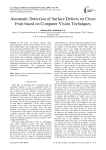
Automatic Detection of Surface Defects on Citrus Fruit based on Computer Vision Techniques
Статья научная
In this paper, we present computer vision based technique to detect surface defects of citrus fruits. The method begins with background removal using k-means clustering technique. Mean shift segmentation is used for fruit region segmentation. The candidate defects are detected using threshold based segmentation. In this stage, it is very difficult to differentiate stem-end from actual defects due to similarity in appearance. Therefore, we proposed a novel technique to differentiate stem-end from actual defects based on the shape features. We conducted experiments on our citrus data set captured in controlled environment. The experiment results demonstrate that our technique outperforms the existing techniques.
Бесплатно
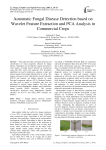
Статья научная
This paper describes automatic detection and classification of visual symptoms affected by fungal disease. Algorithms are developed to acquire and process color images of fungal disease affected on commercial crops like chili, cotton and sugarcane. The developed algorithms are used to preprocess, segment, extract and reduce features from fungal affected parts of a crop. The feature extraction is done with discrete wavelet transform (DWT) and features are further reduced by using Principal component analysis (PCA). Reduced features are then used as inputs to classifiers and tests are performed to classify image samples. We have used statistical based Mahalanobis distance and Probabilistic neural network (PNN) classifiers. The average classification accuracies using Mahalanobis distance classifier are 83.17% and using PNN classifier are 86.48%
Бесплатно
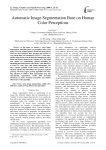
Automatic Image Segmentation Base on Human Color Perceptions
Статья научная
In this paper we propose a color image segmentation algorithm based on perceptual color vision model. First, the original image is divide into image blocks which are not overlapped; then, the mean and variance of every image back was calculated in CIEL*a*b* color space, and the image blocks were divided into homogeneous color blocks and texture blocks by the variance of it. The initial seed regions are automatically selected depending on calculating the homogeneous color blocks' color difference in CIEL*a*b* color space and spatial information. The color contrast gradient of the texture blocks need to calculate and the edge information are stored for regional growing. The fuzzy region growing algorithm and coloredge detection to obtain a final segmentation map. The experimental segmentation results hold favorable consistency in terms of human perception, and confirm effectiveness of the algorithm.
Бесплатно
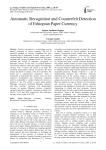
Automatic Recognition and Counterfeit Detection of Ethiopian Paper Currency
Статья научная
Currency recognition is a technology used to identify currencies of various countries. The use of automatic methods of currency recognition has been increasing due its importance in many sectors such as vending machine, railway ticket counter, banking system, shopping mall, currency exchange service, etc. This paper describes the design of automatic recognition of Ethiopian currency. In this work, we propose hardware and software solutions which take images of an Ethiopian currency from a scanner and camera as an input. We combined characteristic features of currency and local feature descriptors to design a four level classifier. The design has a categorization component, which is responsible to denominate the currency notes into their respective denomination and verification component which is responsible to validate whether the currency is genuine or not. The system is tested using genuine Ethiopian currencies, counterfeit Ethiopian currencies and other countries' currencies. The denomination accuracy for genuine Ethiopian currency, counterfeit currencies and other countries' currencies is found to be 90.42%, 83.3% and 100% respectively. The verification accuracy of our system is 96.13%.
Бесплатно
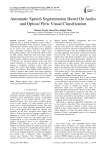
Automatic Speech Segmentation Based On Audio and Optical Flow Visual Classification
Статья научная
Automatic speech segmentation as an important part of speech recognition system (ASR) is highly noise dependent. Noise is made by changes in the communication channel, background, level of speaking etc. In recent years, many researchers have proposed noise cancelation techniques and have added visual features from speaker’s face to reduce the effect of noise on ASR systems. Removing noise from audio signals depends on the type of the noise; so it cannot be used as a general solution. Adding visual features improve this lack of efficiency, but advanced methods of this type need manual extraction of visual features. In this paper we propose a completely automatic system which uses optical flow vectors from speaker’s image sequence to obtain visual features. Then, Hidden Markov Models are trained to segment audio signals from image sequences and audio features based on extracted optical flow. The developed segmentation system based on such method acts totally automatic and become more robust to noise.
Бесплатно
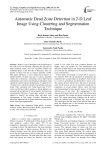
Automatic dead zone detection in 2-D leaf image using clustering and segmentation technique
Статья научная
Plant is a gift of almighty to the living being in the earth. Leaf is an essential component for any types of plant including crops, fruit and vegetables. Before the scheduled decay of the leaf due to deficiency there are patches of dead zone spot or sections generally visible. This paper introduces a novel image based analysis to identify patches of dead zone spot or sections generally visible due to deficiency. Clustering, colour object based segmentation and colour transformation techniques using significant salient features identification are applied over 12 plant leaves collected naturally from gardens and crop fields. Hue, saturation and Value based and L*a*b* colour model based object analysis is being applied over diseased leaf and portion of leaf to identify the dead zone automatically. Derivative based edge analysis is being applied to identify the outline edge and dead zone segmentation in leaf image. K-means clustering has played an important role to cluster dead zone using colour based object area segmentation.
Бесплатно
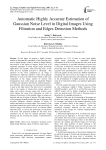
Статья научная
In this paper we propose a highly accurate method of automatically estimation of the Gaussian noise level in digital images, which is based on image filtering and analysis of the region of interest. Noise level is an important parameter to many digital image processing applications, for example, when removing noise. As the noise level its standard deviation is calculated. The selection of the noise component in an image is performed by high-pass filtration, where the Laplacian difference is used as the filter kernel. Based on the noise component of the image, regions of interest with homogeneous areas of the image are calculated. Region of interest are selected by the iterative method using low-pass filtration, where Gaussian two-dimensional function is used as the filter kernel. The noise level is calculated only in the regions of interest that contain almost no edges and textures, because edges and textures cause errors during the noise level estimation. In order to improve the accuracy of the method, edges of images are detected and out of region of interest. The high accuracy of the proposed method provides the use of high-pass and low-pass filtrations, iterative selection of region of interest and analysis of image edges. The accuracy of the developed method has been tested on the processing of 100 test images with different levels of software added Gaussian noise, as well as the processing of real photos with noise. The proposed method for the noise level estimation can be used for optimal automatic image filtering and for assessing the quality of photosensitive sensors.
Бесплатно
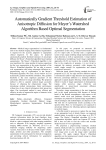
Статья научная
Medical image segmentation is a fundamental task in the medical imaging field. Optimal segmentation is required for the accurate judgment or appropriate clinical diagnosis. In this paper, we proposed automatically gradient threshold estimator of anisotropic diffusion for Meyer’s Watershed algorithm based optimal segmentation. The Meyer’s Watershed algorithm is the most significant for a large number of regions separations but the over segmentation is the major drawback of the Meyer’s Watershed algorithm. We are able to remove over segmentation after using anisotropic diffusion as a preprocessing step of segmentation in the Meyer’s Watershed algorithm. We used a fixed window size for dynamically gradient threshold estimation. The gradient threshold is the most important parameter of the anisotropic diffusion for image smoothing. The proposed method is able to segment medical image accurately because of obtaining the enhancement image. The introducing method demonstrates better performance without loss of any clinical information while preserving edges. Our investigated method is more efficient and effective in order to segment the region of interests in the medical images indeed.
Бесплатно
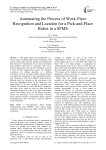
Automating the Process of Work-Piece Recognition and Location for a Pick-and-Place Robot in a SFMS
Статья научная
This paper reports the development of a vision system to automatically classify work-pieces with respect to their shape and color together with determining their location for manipulation by an in-house developed pick-and-place robot from its work-plane. The vision-based pick-and-place robot has been developed as part of a smart flexible manufacturing system for unloading work-pieces for drilling operations at a drilling workstation from an automatic guided vehicle designed to transport the work-pieces in the manufacturing work-cell. Work-pieces with three different shapes and five different colors are scattered on the work-plane of the robot and manipulated based on the shape and color specification by the user through a graphical user interface. The number of corners and the hue, saturation, and value of the colors are used for shape and color recognition respectively in this work. Due to the distinct nature of the feature vectors for the fifteen work-piece classes, all work-pieces were successfully classified using minimum distance classification during repeated experimentations with work-pieces scattered randomly on the work-plane.
Бесплатно
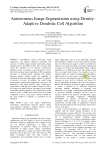
Autonomous Image Segmentation using Density-Adaptive Dendritic Cell Algorithm
Статья научная
Contemporary image processing based applications like medical diagnosis automation and analysis of satellite imagery include autonomous image segmentation as inevitable facility. The research done shows the efficiency of an adaptive evolutionary algorithm based on immune system dynamics for the task of autonomous image segmentation. The recognition dynamics of immune-kernels modeled with infinite Gaussian mixture models exhibit the capability to automatically determine appropriate number of segments in presence of noise. In addition, the model using representative density-kernel-parameters processes the information with much reduced space requirements. Experiments conducted with synthetic images as well as real images recorded assured convergence and optimal autonomous model estimation. The segmentation results tested in terms of PBM-index values have been found comparable to those of the Fuzzy C-Means (FCM) for the same number of segments as generated by our algorithm.
Бесплатно
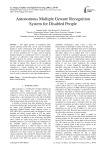
Autonomous Multiple Gesture Recognition System for Disabled People
Статья научная
The paper presents an intelligent multi gesture spotting system that can be used by disabled people to easily communicate with machines resulting into easement in day-to-day works. The system makes use of pose estimation for 10 signs used by hearing impaired people to communicate. Pose is extracted on the basis of silhouettes using timed motion history (tMHI) followed by gesture recognition with Hu-Moments. Signs involving motion are recognized with the help of optical flow. Based on the recognized gestures, particular instructions are sent to the robot connected to system resulting into an appropriate action/movement by the robot. The system is unique as it can act as a assisting device and can communicate in local as well as wide area to assist the disabled person.
Бесплатно
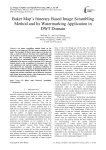
Baker Map's Itinerary Based Image Scrambling Method and Its Watermarking Application in DWT Domain
Статья научная
An image scrambling method based on the itinerary of the improved 3D Baker map is proposed in this paper. The standard 3D Baker map is improved by the tent map, so that the itinerary becomes more complicated and can be used to encode the image pixel positions to scramble the image. The scrambling method is applied to the preprocessing in watermarking. The watermark bits are embedded in the discrete wavelet transform (DWT) spectral domain based on the scrambling of watermark, the odd-even adjustment rule and the neighbor mean value. The watermark bits are embedded in medium coefficients in DWT domain of the host image. Experimental results show that the watermarked image looks visually identical to the original one and the watermark can be effectively extracted upon image processing attacks, which demonstrates strong robustness against a variety of attacks.
Бесплатно
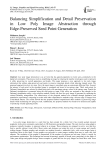
Статья научная
Low poly image abstraction is an art form that has gained popularity in recent years, particularly in the digital art community. The process involves simplifying an image by reducing the number of polygons used to represent it while preserving its overall appearance and details. This paper proposes a new approach to low poly image abstraction that utilizes edge-preserved seed points to preserve important details while reducing triangle count. The proposed approach involves six steps. First, the input image is smoothed using an anisotropic diffusion filter. Second, the entropy of each pixel in the smoothed image is computed and stored in an entropy map. Third, seed points for Delaunay triangulation are selected by identifying pixels with maximum entropy values in the entropy map. Fourth, the Delaunay triangulation is generated using the seed points as input. Fifth, colors are assigned to the triangles in the Delaunay triangulation using a color selection module. Finally, the final low poly image is generated by rendering the colored Delaunay triangulation. The effectiveness of the proposed method was evaluated through qualitative and quantitative experiments, comparing its results with other comprehensive methods using a diverse range of images from a benchmark dataset. The results showed that the proposed method outperformed other methods in preserving image details while maintaining low polygon count. Additionally, the proposed method was found to be efficient and capable of producing visually appealing results.
Бесплатно
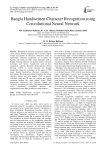
Bangla Handwritten Character Recognition using Convolutional Neural Network
Статья научная
Handwritten character recognition complexity varies among different languages due to distinct shapes, strokes and number of characters. Numerous works in handwritten character recognition are available for English with respect to other major languages such as Bangla. Existing methods use distinct feature extraction techniques and various classification tools in their recognition schemes. Recently, Convolutional Neural Network (CNN) is found efficient for English handwritten character recognition. In this paper, a CNN based Bangla handwritten character recognition is investigated. The proposed method normalizes the written character images and then employ CNN to classify individual characters. It does not employ any feature extraction method like other related works. 20000 handwritten characters with different shapes and variations are used in this study. The proposed method is shown satisfactory recognition accuracy and outperformed some other prominent exiting methods.
Бесплатно
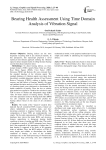
Bearing Health Assessment Using Time Domain Analysis of Vibration Signal
Статья научная
Objective: Bearing defects are the most frequently occurring fault in any electrical machine. In this perspective, this manuscript proposed a novel statistical time-domain approach utilizing the vibration signal to detect incipient faults of rolling-element bearing used in three-phase induction motor. Methodology: To detect bearing defects, six time-domain features (TDFs) namely Mean Value (µ), Peak, Root Mean Square (RMS), Crest Factor (CRF), Skewness (SKW) and Kurtosis (K) were extracted from the standard database of the vibration signal. The standard databases of vibration signals were taken from the publicly available datacenter website of Case Western Reserve University (CWRU) relating to healthy, inner raceway and ball defects of bearing. Initially, the mean and standard deviation analysis of each considered TDFs of vibration signals were performed to discriminate the health conditions of bearing. Then, the box or whisker plot method was applied to visualize the variation in each TDF in terms of median and interquartile range (IQR) value for better analysis of bearing defects. Finally, a new index parameter termed as bearing fault index (BFIT) was also computed and this parameter predicts the bearing defects based on the mean of all considered TDFs. Results: The results of the “mean±σ” analysis have depicted that all considered TDFs except µ feature are almost independent to operating loads, and have discerning potential to diagnose bearing defects. The computations of these TDFs are mathematically very simple. The box plot representation of TDFs of vibration databases have shown that peak, RMS, and skewness features outperforms to demarcate bearing health conditions in terms of median and IQR value. The results of quantitative analysis of BFIT parameter have shown that if the magnitude of this parameter is higher than 1.8 then bearing is supposed to be faulty at all operating loads of machine. Thus, the BFIT analysis of TDFs is more simple and reliable to discriminate the health conditions of bearing. As most of the available techniques rely on the multi-processing of vibration data that requires large processing time and complicated mathematical model, so the proposed method prove to be simple and reliable in identifying the incipient bearing defects.
Бесплатно
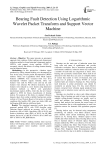
Bearing fault detection using logarithmic wavelet packet transform and support vector machine
Статья научная
Objective: This paper presents an automated approach that combines Fisher ranking and dimensional reduction method as kernel principal component analysis (KPCA) with support vector machine (SVM) to accurately classify the defects of rolling element bearing used in induction motor. Methodology: In this perspective, vibration signal produced by rolling element bearing was decomposed to four levels using wavelet packet decomposition (WPD) method. Thirty one Logarithmic Root Mean Square Features (LRMSF) were extracted from four level decomposed vibration signals. Initially, thirty one features were rank by Fisher score and top ten rank features were selected. For effective detection, top ten features were reduced to a new feature using dimension reduction methods as KPCA and generalized discriminant analysis (GDA). After this, the new feature applied to SVM for binary classification of bearing defects. For analysis of this thirty six standard vibration datasets taken from online available bearing data center website of Case Western Reserve University on bearing conditions like healthy (NF), inner race defect (IR) and ball bearing (BB) defects at different loads. Results: The simulated numerical results show that proposed method KPCA with SVM classifier using Gaussian Kernel achieved an accuracy (AC) of 100, Sensitivity (SE) of 100%, Specificity (SP) of 99.3% and Positive prediction value (PPV) of 99.3% for NF_IRB dataset, and an AC of 100, SE of 99.8%, SP of 100% and PPV of 100% for NF_BBB dataset.
Бесплатно
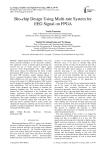
Bio-chip design using multi-rate system for EEG signal on FPGA
Статья научная
Digital Signal Processing (DSP) is one of the fastest growing techniques in the electronics industry. The signal-rate system in digital signal processing has evolved the key of fastest speed in digital signal processor. Field Programmable Gate Array (FPGA) offers good solution for addressing the needs of high performance DSP systems. The focus of this paper is on the basic DSP functions, namely filtering signals to remove unwanted frequency bands. Multi-rate Digital Filters (MDFs) are the main theme to build bio-chip design in this paper. For different purposes DSP systems need to change the sampling rate of the signal to achieve some applications. This can be done using multi-rate system where designers can increase or decrease the operating sampling rate. This bio-chip has attractive features like, low requirement of the coefficient word lengths, significant saving in computation time and storage which results in a reduction in its dynamic power consumption. This paper introduces an efficient FPGA realization of multi-rate digital filter with narrow pass-band and narrow transition band to reduce noises and changing the frequency sampling rate by factor which is required according to application. This bio-chip works on bio-signals like EEG signal.
Бесплатно
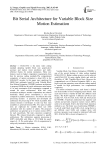
Bit Serial Architecture for Variable Block Size Motion Estimation
Статья научная
H.264/AVC is the latest video coding standard adopting variable block size, quarter-pixel accuracy and motion vector prediction and multi-reference frames for motion estimations. These new features result in higher computation requirements than that for previous coding standards.The computational complexity of motion estimation is about 60% in the H.264/AVC encoder. In this paper most significant bit (MSB first) arithmetic based bit serial Variable Block Size Motion Estimation (VBSME) hardware architecture is proposed. MSB first bit serial architecture main feature is, its early termination SAD computation compared to normal bit serial architectures. With this early termination technique, number computations are reduced drastically. Hence power consumption is also less compared to parallel architectures. An efficient bit serial processing element is proposed and developed 2D architecture for processing of 4x4 block in parallel .Inter connect structure is developed in such way that data reusability is achieved between PEs. Two types of adder trees are employed for variable block size SAD calculation with less number of adders. The proposed architecture can generate up to 41 motion vectors (MVs) for each macroblock. The inter connection complexity between PEs reduced drastically compared to parallel architectures. The architecture supports processing of SDTV (640x480) with 30fps at 172.8 MHz for search range [+8, -7]. We could reduce 14% of computations by using early termination technique.
Бесплатно
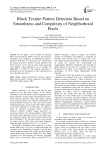
Block Texture Pattern Detection Based on Smoothness and Complexity of Neighborhood Pixels
Статья научная
In this paper, a novel method for detecting Block Texture Patterns (BTP), based on two measures: smoothness and complexity of neighborhood pixels is proposed. With these two measures, a new classification for texture detection is defined. Texture detection with these measures can be used in many image processing and computer vision applications. As an example, the applicability of BTP on data hiding algorithms is discussed, and the advantages of this classification on these algorithms are shown.
Бесплатно
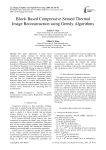
Block-Based Compressive Sensed Thermal Image Reconstruction using Greedy Algorithms
Статья научная
This paper implements a block based compressive sensing technique for thermal image reconstruction using greedy algorithms. A total of fourteen different sensing patterns were tested for data acquisition. Orthogonal Matching Pursuit (OMP) and Regularized Orthogonal Matching Pursuit (ROMP) with two different thresholds were implemented for image reconstruction with OMP having an edge over ROMP in terms of error and PSNR. ROMP was faster in terms of iterations needed for reconstruction. As the threshold for ROMP was increased the number of iterations needed decreased. Gaussian, Bernoulli and Hadamard patterns were the best for reconstruction. Hadamard matrix, Bernoulli matrix with +/-1 entries and Bernoulli matrix with 0/1 entries have the added advantage of being more conducive for hardware implementation. This paper used Discrete Cosine Transform as the sparsifying basis for reconstruction.
Бесплатно