International Journal of Intelligent Systems and Applications @ijisa
Статьи журнала - International Journal of Intelligent Systems and Applications
Все статьи: 1173
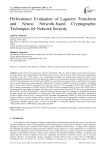
Статья научная
As the world evolves day by day with new technologies, there is a need to design a secure network in such a way that intruders and unauthorized persons should not have access to the network as well as information regarding the personnel in any firm. In this study, a new cryptographic technique for securing data transmission based on the LaplaceLaguerre polynomial (LLP) is developed and compared to an existing auto-associative neural network technique (AANNT).The performance of the LLPT and AANNT was tested with some selected files in a MATLAB environment and the results obtained provided comparative information (in respect of AANNT versus LLPT) as follows: encryption time (1.67 ms versus 3.9931s), decryption time (1.833 ms versus 2.1172s), throughput (26.2975 Kb/s versus 0.01098 Kb/s), memory consumption (3.349 KB versus 15.958 KB). From the compared results, it shows that AANNT offers a faster processing time, higher throughput, and takes up less memory space than the LLPT. However, cryptanalysis of the AANNT is possible if the network's weight and design are known; hence, the technique is unreliable for ensuring the data integrity and confidentiality of encrypted data. The proposed LLP cryptographic algorithm is designed to provide a higher security level by making the LLP algorithm computationally tedious to invert using the standard Laplace transform inversion method. When compared to the AANN-based cryptographic technique, cracking the algorithm to uncover the encryption key takes time. This shows the strength and robustness of the proposed LLP cryptographic algorithm against attacks, as well as its suitability for solving the problem of data privacy and security when compared to the AANN-based cryptographic algorithm.
Бесплатно
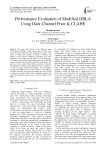
Performance Evaluation of Modified DBLA Using Dark Channel Prior & CLAHE
Статья научная
This paper has focused on the different image enhancement techniques. Image enhancement has found to be one of the most important vision applications because it has ability to enhance the visibility of images. It enhances the quality of poor pictures. Distinctive procedures have been proposed so far for improving the quality of the digital images. To enhance picture quality image enhancement can specifically improve and limit some data presented in the input picture. It is a kind of vision system which reductions picture commotion, kill antiquities, and keep up the informative parts. Its object is to open up certain picture characteristics for investigation, conclusion and further use. The main objective of this paper is to modify the DBLA using the dark channel prior and CLAHE to enhance the results further. The comparative analysis has shown the significant improvement over the CLAHE and the DBLA.
Бесплатно
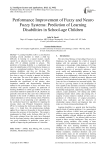
Статья научная
Learning Disability (LD) is a classification including several disorders in which a child has difficulty in learning in a typical manner, usually caused by an unknown factor or factors. LD affects about 15% of children enrolled in schools. The prediction of learning disability is a complicated task since the identification of LD from diverse features or signs is a complicated problem. There is no cure for learning disabilities and they are life-long. The problems of children with specific learning disabilities have been a cause of concern to parents and teachers for some time. The aim of this paper is to develop a new algorithm for imputing missing values and to determine the significance of the missing value imputation method and dimensionality reduction method in the performance of fuzzy and neuro fuzzy classifiers with specific emphasis on prediction of learning disabilities in school age children. In the basic assessment method for prediction of LD, checklists are generally used and the data cases thus collected fully depends on the mood of children and may have also contain redundant as well as missing values. Therefore, in this study, we are proposing a new algorithm, viz. the correlation based new algorithm for imputing the missing values and Principal Component Analysis (PCA) for reducing the irrelevant attributes. After the study, it is found that, the preprocessing methods applied by us improves the quality of data and thereby increases the accuracy of the classifiers. The system is implemented in Math works Software Mat Lab 7.10. The results obtained from this study have illustrated that the developed missing value imputation method is very good contribution in prediction system and is capable of improving the performance of a classifier.
Бесплатно
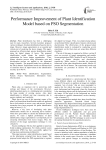
Performance Improvement of Plant Identification Model based on PSO Segmentation
Статья научная
Plant identification has been a challenging task for many researchers. Several researches proposed various techniques for plant identification based on leaves shape. However, image segmentation is an essential and critical part of analyzing the leaves images. This paper, proposed an efficient plant species identification model using the digital images of leaves. The proposed identification model adopts the particle swarm optimization for leaves images segmentation. Then, feature selection process using information gain and discritization process are applied to the segmented image's features. The proposed model was evaluated on the Flavia dataset. Experimental results on different kind of classifiers show an improvement in the identification accuracy up to 98.7%.
Бесплатно
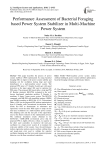
Статья научная
This paper describes the process of power system stabilizer (PSS) optimization by using bacterial foraging (BG) to improve the power system stability and damping out the oscillation during large and small disturbances in a multi-machine power system. The proposed PSS type is P. Kundur (Lead-Lag) with speed deviation as the input signal. BG used to optimize the PSS gains. The proposed BG based delta w lead-lag PSS (P. Kundur structure) (BG-PSS) evaluated in the well-known benchmark simulation problem P. Kundur 4-machines 11-buses 2-areas power system. The BG-PSS compared with MB-PSS with simplified settings: IEEE® type PSS4B according to IEEE Std. 421.5, Conventional Delta w PSS (as the proposed PSS without optimization) from P. Kundur, and Conventional Acceleration Power (Delta Pa) PSS to demonstrate its robustness and superiority versus the three PSSs types to damp out the inter-area oscillations in a multi-machine power system. The damping ratio and the real part of the eigenvalues used as the fitness function in the optimization process. The nonlinear simulation results obtained in the MATLAB / SIMULINK environment prove that the proposed PSS is highly effective, robust, & superior to the other used controllers in restrictive the inter-area oscillation in a large power system & to maintain the wide-area stability of the system. Also, the performance indices eigenvalue analysis, peak overshoot, settling time, and steady-state error used to validate the superior oscillation damping and fast recovered transient dynamic behavior over the three considered controllers.
Бесплатно
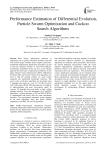
Статья научная
Most design optimization problems in engineering are in general extremely nonlinear and deal with various design variables under complex restrictions. Traditional mathematical optimization procedure may fail to find the optimum solution to real-world problems. Evolutionary Algorithms (EAs) can serve as an efficient approach for these types of optimization problems. In this paper, Particle Swarm Optimization (PSO), Differential Evolution (DE) and Cuckoo Search (CS) algorithms are used to find the optimal solution for some typical unimodal and multimodal benchmark functions. The source codes of all these algorithms are developed using C language and tested on a core i5, 2.4 GHz processor with 8 GB internal RAM. PSO algorithm has a simplicity of implementation and good convergence speed. In contrast, CS algorithm has good ability to find a global optimum solution. To use the advantages of CS and PSO algorithms, a hybrid algorithm of CS and PSO (CSPSO) is implemented and tested with the same benchmark functions. The experimental simulation results obtained by all these algorithms show that hybrid CSPSO outperforms with PSO, DE and CS algorithms.
Бесплатно
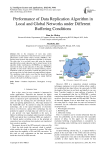
Статья научная
Due to the emergence of more data centric applications, the replication of data has become a more common phenomenon. In the similar context, recently, (PDDRA) a Pre-fetching based dynamic data replication algorithm is developed. The main idea is to pre-fetch some data using the heuristic algorithm before actual replication start to reduce latency In the algorithm further modifications (M-PDDRA) are suggested to minimize the delay in data replication. In this paper, M-PDDRA algorithm is tested under shared and output buffering scheme. Simulation results are presented to estimate the packet loss rate and average delay for both shared and output buffered schemes. The simulation results clearly reveal that the shared buffering with load balancing scheme is as good as output buffered scheme with much less buffering resources.
Бесплатно
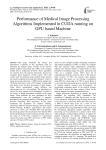
Performance of medical image processing algorithms implemented in CUDA running on GPU based machine
Статья научная
This paper illustrates the design and performance evaluation of few algorithms used for analysing the medical image volumes on the massive parallel graphics processing unit (GPU) with compute unified device architecture (CUDA). These algorithms are selected from the general framework, devised for computer aided diagnostic (CAD) system. The CAD system used for analysing large medical image datasets are usually a pipeline processing that includes a variety of image processing operations. A MRI scanner captures the 3D human head into a series of 2D images. Considerable time spent in pre and post processing of these images. Noise filters, segmentation, image diffusion and enhancement are few such methods. The algorithms are chosen for study requires local information, available in few pixels or global information available in the entire image. These problems are best candidates for GPU implementation, since the parallelism is naturally provided by the proposed Per-Pixel Threading (PPT) or Per-Slice Threading (PST) operations. In this paper implement the algorithms for adaptive filtering, anisotropic diffusion, bilateral filtering, non-local means (NLM) filtering, K-Means segmentation and feature extraction in 1536 core’s NVIDIA GPU and estimated the speed up gained. Our experiments show that the GPU based implementation achieved typical speedup values in the range of 3-338 times compared to conventional central processing unit (CPU) processor in PPT model and up to 30 times in PST model.
Бесплатно
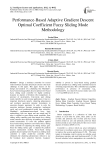
Performance-Based Adaptive Gradient Descent Optimal Coefficient Fuzzy Sliding Mode Methodology
Статья научная
Design a nonlinear controller for second order nonlinear uncertain dynamical systems is the main challenge in this paper. This paper focuses on the design and analysis of a chattering free Mamdani’s fuzzy-based tuning gradient descent optimal error-based fuzzy sliding mode controller for highly nonlinear dynamic six degrees of freedom robot manipulator, in presence of uncertainties. Conversely, pure sliding mode controller is used in many applications; it has two important drawbacks namely; chattering phenomenon and nonlinear equivalent dynamic formulation in uncertain dynamic parameter. In order to solve the uncertain nonlinear dynamic parameters, implement easily and avoid mathematical model base controller, Mamdani’s performance/error-based fuzzy logic methodology with two inputs and one output and 49 rules is applied to pure sliding mode controller. Pure sliding mode controller and error-based fuzzy sliding mode controller have difficulty in handling unstructured model uncertainties. To solve this problem applied fuzzy-based tuning method to error-based fuzzy sliding mode controller for adjusting the sliding surface gain. Since the sliding surface gain is adjusted by gradient descent optimization method. Fuzzy-based tuning gradient descent optimal error-based fuzzy sliding mode controller is stable model-free controller which eliminates the chattering phenomenon without to use the boundary layer saturation function. Lyapunov stability is proved in fuzzy-based tuning gradient descent optimal fuzzy sliding mode controller based on switching (sign) function. This controller has acceptable performance in presence of uncertainty (e.g., overshoot=0%, rise time=0.8 second, steady state error = 1e-9 and RMS error=1.8e-12).
Бесплатно
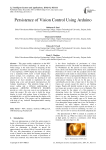
Persistence of Vision control using Arduino
Статья научная
This paper mainly emphasizes on the POV (Persistence Of Vision) technology. In current era in which energy is the main factor in designing all the applications, maximum and efficient use of the energy is very important. A POV display has many advantages over a traditional CRT, LCD or LED display, like power savings, less complexity, easy configuration, attractiveness etc. To overcome the drawback of old processor we have decided to implement the same display atop a new and advanced microprocessor, the Arduino duemilanove. This platform brings with it newer coding and a different understanding of peripherals. ARDUINO INTERFACE BOARDS provide us with a low-cost, easy-to use technology to create the project. We also aim to build the newer display to work with modern forms of interfaces. To accomplish this, we will be interfacing the display with an Android device. This project can be implemented with help of any Android Smartphone/tablet running Android 4.0+.
Бесплатно
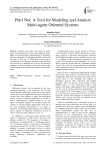
Petri Net: A Tool for Modeling and Analyze Multi-agent Oriented Systems
Статья научная
Analysis and proper assessment of multi-agent system properties are very much important. In this paper, we discussed about methodologies for modeling, analysis and design of multi-agent oriented system with the help of Petri net. A Multi-agent system can be considered as a discrete-event dynamic system and Petri nets are used as a modeling tool to assess the structural properties of the multi-agent system. Petri net provides an assessment of the interaction properties of the multi-agent.
Бесплатно
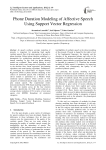
Phone Duration Modeling of Affective Speech Using Support Vector Regression
Статья научная
In speech synthesis accurate modeling of prosody is important for producing high quality synthetic speech. One of the main aspects of prosody is phone duration. Robust phone duration modeling is a prerequisite for synthesizing emotional speech with natural sounding. In this work ten phone duration models are evaluated. These models belong to well known and widely used categories of algorithms, such as the decision trees, linear regression, lazy-learning algorithms and meta-learning algorithms. Furthermore, we investigate the effectiveness of Support Vector Regression (SVR) in phone duration modeling in the context of emotional speech. The evaluation of the eleven models is performed on a Modern Greek emotional speech database which consists of four categories of emotional speech (anger, fear, joy, sadness) plus neutral speech. The experimental results demonstrated that the SVR-based modeling outperforms the other ten models across all the four emotion categories. Specifically, the SVR model achieved an average relative reduction of 8% in terms of root mean square error (RMSE) throughout all emotional categories.
Бесплатно
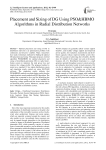
Placement and Sizing of DG Using PSO&HBMO Algorithms in Radial Distribution Networks
Статья научная
Optimal placement and sizing of DG in distribution network is an optimization problem with continuous and discrete variables. Many researchers have used evolutionary methods for finding the opti-mal DG placement and sizing. This paper proposes a hybrid algorithm PSO&HBMO for optimal placement and sizing of distributed generation (DG) in radial distribution system to minimize the total power loss and improve the voltage profile. The proposed method is tested on a standard 13 bus radial distribution system and simulation results carried out using MATLAB software. The simulation results indicate that PSO&HBMO method can obtain better results than the simple heuristic search method and PSO algorithm. The method has a potential to be a tool for identifying the best location and rating of a DG to be installed for improving voltage profile and line losses reduction in an electrical power system. Moreover, current reduction is obtained in distribution system.
Бесплатно
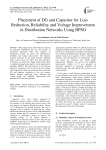
Статья научная
This paper presents multi-objective function for optimally determining the size and location of distributed generation (DG) and capacitor in distribution systems for power loss minimization, reliability and voltage improvement. The objective function proposed in this paper includes reliability index, active power loss index, DG's and capacitor's investment cost index and voltage profile index which is minimized using binary particle swarm optimization algorithm (BPSO). The effectiveness of the proposed method is examined in the 10 and 33 bus test systems and comparative studies are conducted before and after DG and capacitor installation in the test systems. Results illustrate significant losses reduction and voltage profile and reliability improvement with presence of DG unit and capacitor.
Бесплатно
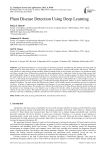
Plant Disease Detection Using Deep Learning
Статья научная
Agricultural development is a critical strategy for promoting prosperity and addressing the challenge of feeding nearly 10 billion people by 2050. Plant diseases can significantly impact food production, reducing both quantity and diversity. Therefore, early detection of plant diseases through automatic detection methods based on deep learning can improve food production quality and reduce economic losses. While previous models have been implemented for a single type of plant to ensure high accuracy, they require high-quality images for proper classification and are not effective with low-resolution images. To address these limitations, this paper proposes the use of pre-trained model based on convolutional neural networks (CNN) for plant disease detection. The focus is on fine-tuning the hyperparameters of popular pre-trained model such as EfficientNetV2S, to achieve higher accuracy in detecting plant diseases in lower resolution images, crowded and misleading backgrounds, shadows on leaves, different textures, and changes in brightness. The study utilized the Plant Diseases Dataset, which includes infected and uninfected crop leaves comprising 38 classes. In pursuit of improving the adaptability and robustness of our neural networks, we intentionally exposed them to a deliberately noisy training dataset. This strategic move followed the modification of the Plant Diseases Dataset, tailored to better suit the demands of our training process. Our objective was to enhance the network's ability to generalize effectively and perform robustly in real-world scenarios. This approach represents a critical step in our study's overarching goal of advancing plant disease detection, especially in challenging conditions, and underscores the importance of dataset optimization in deep learning applications.
Бесплатно
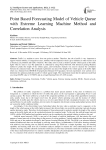
Статья научная
Traffic is a medium to move from one point to another. Therefore, the role of traffic is very important to support vehicle mobility. If congestion occurs, mobility will be hampered so that it gives influence to other sectors such as financial, air pollution and traffic violations. This study aims to create a model to predict vehicle queue at the traffic lights when its status is red. The prediction is conducted by using Neural Network with Extreme Learning Machine method to predict the length of the vehicle queue, and Correlation Analysis was used to measure the correlation between the connected roads. The conducted experiments use data of the length of the vehicle queue at the traffic lights which was obtained from DISHUB (Transportation Bureau) DI Yogyakarta. Several experiments were carried out to determine the optimum prediction model of vehicle queue length. The experiments found that the optimum model had an average MAPE value of 15.5882% and an average Running Time of 5.2226 seconds.
Бесплатно
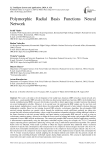
Polymorphic Radial Basis Functions Neural Network
Статья научная
The work is devoted to the development of the radial basis functions (RBF networks) neural network new architecture – a polymorphic RBF network in which the one-dimensional radial basis functions (RBFs) in the hidden layer instead, multidimensional RBFs are used, which makes it possible to better approximate complex functions that depend on several independent variables. Moreover, in its second layer, the summing the RBF outputs one by one from each group instead, multiplication is used, which allows the polymorphic RBF network to better identify relations between independent variables. Based on the training classical RBF networks evolutionary algorithm, the polymorphic RBF network training algorithm was created, which, through the initializing weight coefficients methods use taking into account the tasks structure and preliminary values, using the mutations tournament selection, adding additional criteria to the fitness function to take into account stability and speed training a polymorphic RBF network, as well as using an evolutionary mutation strategy, allowed us to obtain the lowest errors in training and testing a polymorphic RBF network compared to known RBF network architectures. The created polymorphic RBF network practical application possibility is demonstrated experimentally using the helicopters turboshaft engines (using the example, the TV3-117 turboshaft engine) operating process parameters optimizing solving task using a multicriteria optimization algorithm. The optimal Pareto front was obtained, which made it possible to obtain the engine operation three additional modes: maximum reduction of specific fuel consumption at the total pressure in the compressor increase degree increased value by 5.0 %, specific fuel consumption minimization at the total pressure in the compressor increase degree reduced value by 1.0 %, the total pressure in the compressor increases degree optimal value with a slight increase in specific fuel consumption by 10.5 %. Future research prospects include adapting the developed methods and models into the general concept for monitoring and controlling helicopter turboshaft engines during flight operations. This concept is implemented in the neural network expert system and the on-board automatic control system.
Бесплатно
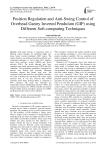
Статья научная
This paper presents a comparison study of different control strategies for stabilizing highly non-linear Gantry inverted pendulum (GIP) system. The control objective was achieved using three different soft-computing techniques i.e. Fuzzy logic (FL), Adaptive neuro fuzzy inference system (ANFIS) and Neural networks (NN's). The results obtained from fuzzy controller were further optimized using ANFIS and NN's controllers. The performance parameters considered for analysis were Settling time (seconds), Maximum Overshoot (degree) and Steady state error. The simulation results that both fuzzy and ANFIS controllers were able to stabilize the non-linear GIP system within specified time. It was also observed that ANFIS controller shows better learning ability as compared to NN's controller. The study also elaborates the relationship between Membership functions (MF's) and training error tolerance for ANFIS controller and relation between hidden neurons and Mean squared error (MSE) and Regression (R) value for NN's controller.
Бесплатно
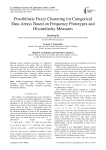
Статья научная
Fuzzy clustering procedures for categorical data are proposed in the paper. Most of well-known conventional clustering methods face certain difficulties while processing this sort of data because a notion of similarity is missing in these data. A detailed description of a possibilistic fuzzy clustering method based on frequency-based cluster prototypes and dissimilarity measures for categorical data is given.
Бесплатно
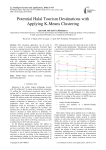
Potential halal tourism destinations with applying K-Means clustering
Статья научная
The clustering application can be used to develop a variety of tourism potential. Currently, halal tourism is a national income that increases every year and is a favorite for Indonesia. The development of halal tourism is supported by a majority population Muslim and as a halal tourist destination in the world. The objective of this study is to investigate the number of clustering with partitioning approach i.e. K-Means (KM) with two simulation scenarios. The characteristics similarity of this method refers to 11 indicators in 2017 Global Muslim Travel Index (GMTI). The output of this study is to display the information in the form of a map and make it easier for the public to determine which halal tourism destinations are high, medium, and low potential.
Бесплатно